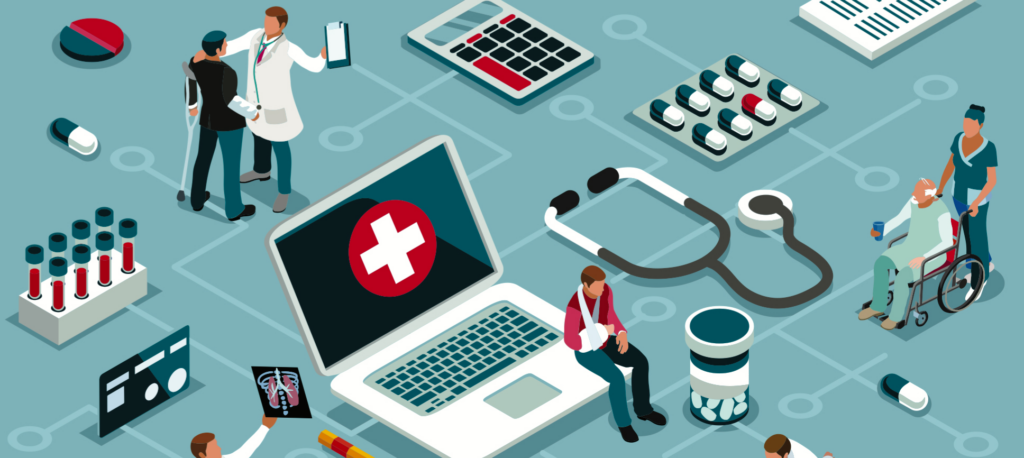
In recent years, the healthcare industry has been undergoing a transformative shift driven by the integration of advanced technologies. Among these innovations, Artificial Intelligence (AI) stands out as a powerful force, especially in the realm of personalized medicine. By tailoring healthcare decisions, treatments, and products to individual patient characteristics, AI-driven personalized medicine promises more accurate diagnoses, targeted therapies, and improved patient outcomes.
This blog explores how AI is reshaping personalized medicine, the technologies and methodologies involved, benefits for patients and healthcare providers, ethical concerns, and real-world applications across the healthcare landscape.
What Is Personalized Medicine?
Personalized medicine—also known as precision medicine—is a medical model that customizes treatment based on individual patient data. Instead of the traditional “one-size-fits-all” approach, it considers a person’s:
- Genetic makeup
- Medical history
- Lifestyle and environment
- Biomarkers and biological responses
This approach enhances the efficacy and safety of medical treatments by predicting how different patients will respond to specific interventions.
The Role of AI in Personalized Medicine
The application of AI in personalized medicine involves the use of algorithms, data analysis, and machine learning models to interpret complex datasets. These models identify patterns, predict outcomes, and recommend tailored treatments that would be difficult or impossible for human clinicians to detect unaided.
AI systems in this context are trained on diverse data sources such as:
- Genomic and proteomic data
- Electronic health records (EHRs)
- Radiology and pathology images
- Wearable sensor data
- Clinical trial results
- Real-time patient monitoring data
AI enables healthcare systems to analyze vast datasets quickly and generate insights that inform individualized care plans.
Key AI Technologies Powering Personalized Medicine
Several AI technologies play a pivotal role in advancing personalized healthcare:
1. Machine Learning (ML)
ML algorithms learn from historical data to make predictions or decisions without being explicitly programmed. In personalized medicine, ML helps predict disease progression, drug responses, and treatment outcomes.
2. Deep Learning
A subset of ML, deep learning uses neural networks to analyze unstructured data such as images or genomics. For instance, deep learning models can identify mutations in cancer cells or interpret MRI scans with remarkable accuracy.
3. Natural Language Processing (NLP)
NLP enables AI to extract meaningful insights from unstructured clinical notes, research papers, and patient records. It plays a critical role in integrating and analyzing diverse data types.
4. Computer Vision
This AI technique interprets medical images such as X-rays, CT scans, and histopathological slides to detect anomalies or track disease progression, aiding diagnosis and treatment planning.
5. Reinforcement Learning
Used in treatment optimization, reinforcement learning helps refine therapy protocols by learning from feedback loops and patient responses over time.
Applications of AI-Driven Personalized Medicine
1. Genomic Medicine
AI helps interpret vast genomic datasets to identify mutations linked to diseases like cancer or inherited disorders. Personalized treatment plans based on genetic profiles are now increasingly used, especially in oncology.
For example, AI algorithms can suggest targeted therapies for breast cancer patients based on HER2 gene expression, significantly improving outcomes.
2. Predictive Analytics for Disease Prevention
AI models can analyze patient data to forecast the risk of developing chronic conditions such as diabetes, cardiovascular diseases, or Alzheimer’s. Early identification allows clinicians to recommend preventive strategies tailored to each individual.
3. Customized Drug Development
AI accelerates drug discovery by predicting how molecules interact with specific genetic profiles. This leads to faster, more cost-effective development of targeted drugs.
4. Treatment Optimization
AI can simulate different treatment pathways and recommend the best course of action for individual patients. For instance, in oncology, AI can analyze tumor characteristics and past patient responses to identify the most effective chemotherapy regimen.
5. Remote Patient Monitoring and Wearables
AI-driven devices can continuously collect data on heart rate, blood glucose levels, or physical activity. This real-time information feeds into personalized care plans and alerts healthcare providers to anomalies.
6. Personalized Mental Health Support
AI tools analyze speech, text, and behavioral patterns to assess mental health conditions and recommend individualized therapies. Chatbots and digital mental health platforms increasingly use AI for early detection and support.
Benefits of AI-Powered Personalized Medicine
A. Improved Patient Outcomes
By aligning treatments with an individual’s biological makeup and lifestyle, AI-driven personalized medicine enhances the effectiveness of interventions and reduces adverse effects.
B. Early Detection and Intervention
AI can identify subtle patterns in medical data that indicate early stages of disease, enabling timely and potentially life-saving interventions.
C. Cost-Effective Healthcare
Targeted treatments reduce trial-and-error prescribing and unnecessary diagnostics, helping to lower healthcare costs and improve resource allocation.
D. Enhanced Clinical Decision Support
AI offers physicians data-driven insights and evidence-based recommendations, supporting faster and more accurate decision-making.
E. Empowered Patients
Patients gain a better understanding of their own health through personalized insights, promoting engagement and adherence to treatment plans.
Ethical and Practical Challenges
Despite its potential, AI-powered personalized medicine raises important concerns and barriers:
1. Data Privacy and Security
Handling sensitive health and genetic data requires stringent data protection protocols. Breaches could have severe implications for patient confidentiality.
2. Bias in AI Algorithms
AI models trained on biased or non-diverse datasets may produce skewed results, potentially disadvantaging underrepresented groups.
3. Interpretability
Black-box AI models may provide accurate predictions, but their lack of transparency can be problematic in critical healthcare decisions. Clinicians and patients need to understand how conclusions are reached.
4. Regulatory Hurdles
There is a lack of standardized regulations and guidelines for integrating AI into personalized medicine, which can slow adoption and innovation.
5. Integration with Clinical Workflows
Introducing AI systems into existing clinical environments requires significant training, system upgrades, and cultural shifts, which can pose resistance.
Real-World Examples of AI in Personalized Medicine
IBM Watson for Oncology
IBM’s Watson analyzes large volumes of medical literature, clinical data, and patient records to assist oncologists in creating personalized cancer treatment plans. It offers evidence-based recommendations tailored to individual cases.
Tempus
Tempus uses AI and genomic sequencing to provide physicians with insights that inform personalized cancer treatment. Their platform combines clinical data with molecular profiles to improve therapeutic decisions.
Deep Genomics
This biotech company leverages AI to identify genetic mutations and design RNA-based treatments, offering a faster, more accurate approach to rare genetic diseases.
PathAI
PathAI applies AI to pathology images, enabling more precise diagnostics and personalized cancer care. It helps identify biomarkers and predict therapy responses.
The Future of Personalized Medicine with AI
As AI technologies mature and data availability grows, the potential of personalized medicine will continue to expand. Key future developments may include:
- Integration of multi-omics data (genomics, proteomics, metabolomics) for deeper insights
- AI-powered digital twins of patients to simulate disease progression and treatment responses
- Decentralized clinical trials using AI for patient selection, monitoring, and analysis
- Federated learning approaches to enable AI training on distributed, privacy-sensitive medical datasets
Ultimately, the fusion of AI and personalized medicine marks a shift toward proactive, predictive, and preventive care—where treatment is not only reactive but deeply individualized.
Conclusion
AI-driven personalized medicine is redefining the way healthcare is delivered. By leveraging the power of data and advanced analytics, it empowers clinicians to deliver more precise, effective, and timely treatments. For patients, this means better outcomes, fewer side effects, and a more engaged role in their own care.
However, realizing the full promise of this transformation requires addressing challenges around ethics, transparency, and implementation. Stakeholders—including healthcare providers, technologists, regulators, and patients—must collaborate to ensure that AI is used responsibly and equitably.
In the coming years, as AI systems become more sophisticated and integrated into clinical practice, personalized medicine is poised to become the standard of care, rather than the exception.