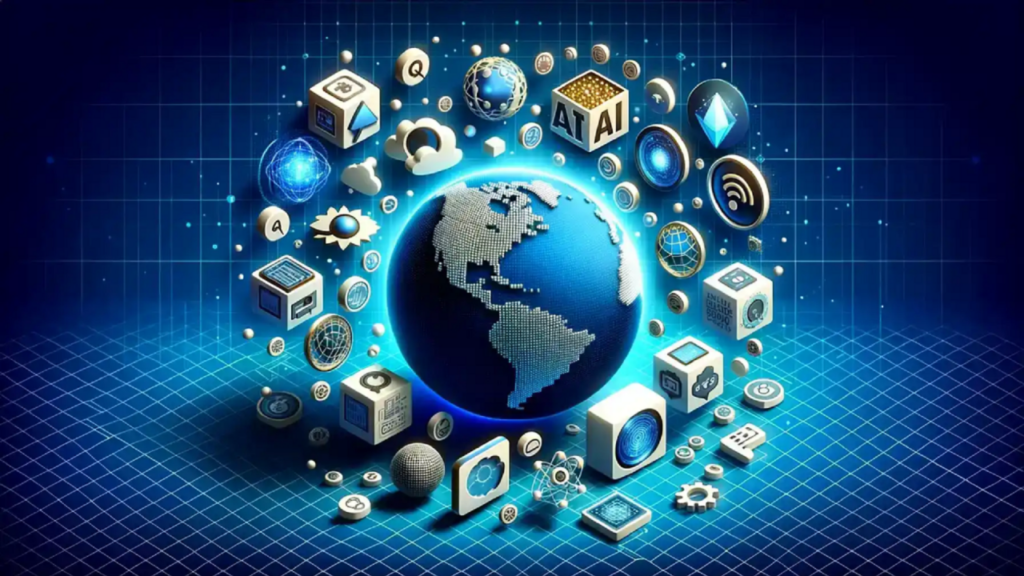
The dawn of generative AI has marked a pivotal moment in human history. With the ability to produce text, images, video, code, and even music autonomously, tools like ChatGPT, DALL·E, Midjourney, and Runway have transformed industries and sparked societal debates. But beyond the hype and innovation lies a deeper question: What does widespread generative AI adoption mean for society?
In this article, we’ll explore the far-reaching societal implications—both positive and problematic—of a world where generative AI is a mainstream technology.
A Paradigm Shift in Creativity and Productivity
Generative AI dramatically alters how humans create, work, and express themselves. By automating once-manual tasks like writing, drawing, composing, and designing, AI has democratized creative potential.
1. Redefining Human Creativity
With AI tools assisting or even generating content autonomously, the very nature of creativity is being re-evaluated. Artists and writers are now collaborators with algorithms, and novices can produce professional-level content with minimal effort.
While some celebrate this empowerment, others question whether over-reliance on AI might dilute original thinking or reduce the incentive to develop human creative skills.
2. Enhanced Productivity Across Sectors
From marketing and customer support to journalism and software development, AI is speeding up workflows. For instance:
- Marketers use AI to generate social media copy and ad creatives.
- Journalists use it for draft writing and headline suggestions.
- Developers use tools like GitHub Copilot to autocomplete code.
This efficiency enables businesses to scale content production and frees up human labor for higher-order tasks—but also introduces concerns about job displacement.
Employment and Economic Disruption
One of the most pressing concerns with generative AI is its impact on the labor market.
3. Job Displacement vs. Job Creation
Roles based on repetitive, predictable outputs—like basic design, copywriting, or coding—face partial automation. McKinsey predicts that up to 30% of work hours in the U.S. could be automated by 2030, in part due to generative AI.
However, new roles are emerging:
- AI trainers, who fine-tune models using domain expertise.
- Prompt engineers, who craft inputs to maximize output quality.
- AI ethicists and compliance officers, needed to ensure responsible use.
The key lies in reskilling the workforce and creating a safety net for those most affected by the transition.
Education, Misinformation, and Critical Thinking
4. Changing the Education Landscape
Generative AI challenges traditional teaching and learning models. Students now use ChatGPT for essays, math problems, and language practice. While it can enhance personalized learning, it also raises cheating concerns.
Educators are adapting by:
- Emphasizing critical thinking and human reasoning over rote tasks.
- Teaching students how to use AI responsibly, not just detect it.
5. Misinformation and Deepfakes
AI can fabricate convincing but false content—deepfake videos, fake news articles, synthetic reviews—blurring the line between fact and fiction.
This erodes trust in media, institutions, and even one another. As a result:
- News organizations are investing in AI-detection tools.
- Governments and platforms are exploring digital watermarking and content provenance to verify authenticity.
Psychological and Cultural Impact
6. Shaping Perception and Identity
Generative AI influences how individuals perceive beauty, culture, and self-expression. AI-generated models on social media or synthetic influencers in advertising can distort reality, affecting body image, self-esteem, and social comparisons.
Cultural homogenization is another risk. If global models are trained on dominant languages and media, smaller cultural narratives may be underrepresented or erased.
7. Emotional Attachment to AI
People are forming emotional bonds with chatbots, virtual companions, and AI characters. While this can provide comfort or accessibility (e.g., in mental health apps), it also raises ethical concerns:
- Should people rely emotionally on systems that simulate, but don’t feel?
- What are the implications of anthropomorphizing machines?
Ethics, Bias, and Representation
8. Reinforcing Social Biases
Generative AI models are only as unbiased as their training data. If that data includes stereotypes, prejudiced language, or unequal representation, AI can replicate and amplify those issues.
Examples include:
- AI generating male CEOs and female assistants.
- Underrepresentation of non-Western cultures in image generation.
Efforts to address this include:
- Auditing training datasets.
- Diversifying content sources.
- Embedding fairness constraints into model architectures.
9. Intellectual Property and Creator Rights
Artists and writers have raised alarms about their work being used to train models without consent or compensation. While some platforms now allow opt-outs, legal frameworks around AI training data are still evolving.
This has sparked a broader debate: Is training on copyrighted content fair use, or exploitation? Ongoing lawsuits and regulatory action may shape the future of AI-generated content.
Privacy and Surveillance
10. Data Exposure and Consent
Generative AI can unintentionally memorize and reproduce personal data—such as names, addresses, or internal documents—if that data appeared in training sets.
This raises significant privacy concerns:
- What safeguards exist against re-identification?
- Are users giving informed consent when content is scraped?
11. Surveillance and Manipulation
Governments and corporations can use generative AI to manipulate public opinion (e.g., through fake personas or mass-generated narratives) or automate surveillance through voice/video synthesis and facial recognition.
Robust data governance, transparency, and democratic oversight are vital to prevent abuse.
The Future: Adaptation, Regulation, and Co-Evolution
12. The Need for Regulation
Governments and institutions are beginning to craft laws that address generative AI’s societal impact:
- EU AI Act requires transparency from foundation model providers.
- U.S. Executive Order on AI (2023) encourages safety testing and watermarking.
- China mandates alignment with “socialist core values” in generative output.
However, regulation must walk a tightrope between fostering innovation and protecting society. It needs to be:
- Flexible to keep pace with rapid technological change.
- Collaborative, involving diverse stakeholders (governments, developers, civil society).
- Global, given the borderless nature of digital content.
13. Human-AI Collaboration, Not Replacement
The long-term vision isn’t about AI replacing people—it’s about augmenting human capabilities. In fields from architecture to medicine, humans and AI will increasingly work together, combining creativity and computation.
This requires a cultural shift:
- Embracing lifelong learning and digital fluency.
- Redefining what it means to be “skilled” in an AI-augmented world.
- Reaffirming human judgment, empathy, and values as irreplaceable.
Widespread generative AI adoption is not just a technological shift—it’s a societal transformation. It touches nearly every aspect of life: how we work, learn, communicate, create, and govern.
While the promises are immense—greater accessibility, productivity, and innovation—so are the risks: misinformation, job displacement, ethical dilemmas, and social disruption.
The road ahead calls for collective responsibility. Developers must build ethically. Governments must legislate wisely. Educators must teach critically. And all of us must engage thoughtfully—with curiosity, caution, and a commitment to shaping AI in the service of humanity.
Generative AI is not just a tool—it’s a mirror. What it reflects will depend on how we choose to wield it.