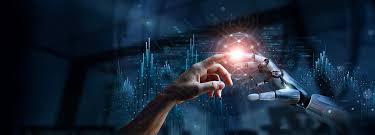
In recent years, artificial intelligence has transitioned from an abstract technological concept into a deeply embedded part of modern life. Among its most intriguing developments is the surge of personalized generative AI. Unlike traditional automation tools, these systems are capable of creating custom-tailored outputs based on individual preferences, behaviors, and contexts. This shift toward personalization marks a new era where machines not only understand users but also adapt, co-create, and respond in profoundly individualized ways.
What Is Personalized Generative AI?
Personalized generative AI refers to systems that produce text, visuals, audio, or other digital content dynamically and uniquely for each user. Instead of providing generic results, these tools tailor outputs by analyzing user data such as browsing history, language use, interests, and even mood.
Imagine an AI writing a bedtime story that includes your child’s name, favorite animals, and preferred plot elements. Or consider a music generation tool that produces soundtracks aligned with your current emotional state. This isn’t science fiction—it’s the practical application of generative algorithms informed by user-specific inputs.
Personalization has long existed in digital spaces, such as recommendation engines on streaming platforms. However, generative AI takes this to the next level by not just suggesting content, but creating it from scratch, in real time, and based on highly individualized cues.
Evolution of Generative AI Toward Personalization
The early days of generative AI were characterized by models trained to perform general tasks—translating languages, summarizing documents, or creating art. These outputs were impressive but not necessarily personal.
The turning point came with the development of models like OpenAI’s GPT series, DALL·E, and Google’s Imagen. These systems could generate text and images based on natural language prompts. As models became more sophisticated, developers began exploring ways to incorporate user-specific data. This included preferences, historical inputs, and contextual signals, such as location or time of day.
What followed was the birth of generative personalization, where the AI system remembers prior interactions, learns a user’s style, and produces responses or content that feel uniquely “yours.”
Key Technologies Driving Personalization
Several advancements have enabled the growth of personalized generative systems:
- Large Language Models (LLMs): These models understand syntax, semantics, tone, and context. When fine-tuned with personal data, they produce responses that reflect an individual’s unique voice or needs.
- Reinforcement Learning with Human Feedback (RLHF): This method improves responses based on user preferences and corrections, helping AI align with human values over time.
- Contextual Memory: Advanced systems can retain conversation history or user data, allowing them to respond more consistently and personally across interactions.
- Embedding Techniques: Embeddings capture the “meaning” of words, images, or actions and store them in vector form. By matching embeddings to user preferences, AI can tailor responses to semantic expectations.
- API Integrations and User Profiles: Many modern platforms allow users to link calendars, playlists, emails, and notes. This data can inform generative outputs with real-world relevance.
Personalized Applications Across Sectors
The adoption of personalized generative AI is accelerating in numerous industries. Let’s explore how different sectors are leveraging it:
Education
In digital classrooms, AI can act as a tutor that understands a student’s strengths and weaknesses. It can explain algebra in simpler terms to one student while challenging another with advanced problems. Systems can also generate study guides or summaries that reflect a learner’s pace and style of comprehension.
Healthcare
Generative AI can create wellness plans based on individual medical histories, dietary restrictions, and activity levels. In mental health, chatbots trained on therapeutic principles can provide supportive conversation tailored to the user’s emotional state.
Entertainment
Content creation is rapidly transforming. Streaming services use generative models to recommend shows or even create custom trailers. AI DJs can spin music sets based on real-time listener feedback or mood inputs. Writers use generative tools to develop storylines matching their voice, genre, and structure.
Retail and E-commerce
Brands now use AI to generate personalized product descriptions, advertisements, and customer interactions. Virtual shopping assistants can respond to queries with detailed, user-specific advice. Clothing companies even simulate outfit suggestions based on body type, color preference, and seasonal trends.
Productivity and Workflows
From custom email drafting to tailored meeting summaries, AI is making workplace tools smarter. Calendar assistants can suggest ideal meeting times based on past behavior. Generative tools write reports that reflect a team’s specific priorities and tone.
Benefits of Personalized Generative AI
The shift toward personalization brings several compelling advantages:
- Improved Engagement: People are more likely to interact with systems that respond in a personal, human-like manner. Personalized content keeps users interested and invested.
- Enhanced Efficiency: AI saves users time by anticipating their needs. Instead of asking for specific actions, users receive proactive and relevant assistance.
- Stronger Customer Loyalty: Brands offering hyper-personalized experiences foster trust and retention. When users feel seen and understood, they’re more likely to return.
- Creative Empowerment: Writers, artists, and musicians can use personalized tools to co-create, generating drafts, visuals, or soundscapes that align with their vision.
Challenges and Ethical Concerns
Despite the promise, the rise of personalized generative AI is not without issues.
- Privacy Risks: AI systems require access to personal data to function effectively. If mishandled, this can lead to data breaches, misuse, or over-surveillance.
- Bias and Fairness: Personalization can unintentionally reinforce existing biases. If a model learns from skewed data, it may amplify harmful stereotypes or exclude diverse perspectives.
- Overdependence: Highly personalized AI might lead users to rely too heavily on machines for tasks they used to perform independently, reducing critical thinking or creativity.
- Transparency: When generative systems tailor content, it’s often unclear what data they are using or why they made certain decisions. This lack of visibility can be problematic, especially in sensitive contexts.
To address these concerns, developers must prioritize transparency, user control, and ethical design. Offering opt-in features, clear data usage policies, and customization settings helps users maintain agency.
The Future of Personalized AI
As we look ahead, several trends will shape the next chapter of personalized generative AI:
- Multimodal Personalization: Future systems will not only remember your words but also your visual style, tone of voice, and preferred formats. Whether it’s an email, a video, or an illustration, the AI will adapt accordingly.
- Edge Computing: Personalized models will increasingly run on personal devices instead of centralized servers, reducing privacy risks and latency while maintaining performance.
- Emotional Intelligence: Future AIs may detect subtle emotional cues and respond with appropriate sensitivity. This could lead to more supportive and empathetic interactions in fields like healthcare or customer service.
- Open Personal AI Agents: Instead of closed platforms, users will have their own AI agents trained on their data, preferences, and behaviors—portable across services and entirely under personal control.
- Collaboration, Not Replacement: Generative AI will become a co-pilot rather than a replacement. Whether helping you brainstorm, visualize, write, or plan, it will act as a personal assistant that enhances human creativity rather than replacing it.
Conclusion
Personalized generative AI is reshaping how we interact with machines and content. These systems move beyond generalized intelligence, crafting responses, visuals, and experiences that resonate on an individual level. From education to retail, entertainment to wellness, their impact is both deep and wide-reaching.
While challenges remain—especially around privacy, fairness, and transparency—the opportunities are immense. As technology continues to evolve, one thing is clear: the future will not be one-size-fits-all. It will be as varied and unique as the individuals who engage with it, powered by intelligent systems that understand us better than ever before.