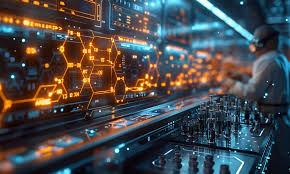
In the modern age of discovery, science is advancing at a pace once thought impossible. From decoding the human genome to exploring distant galaxies, the frontiers of human understanding are constantly expanding. Yet, even as scientific fields grow more complex, a new catalyst has emerged—generative artificial intelligence (AI). This branch of machine learning is no longer confined to artistic endeavors like image creation or language generation. Today, it is rapidly transforming the landscape of scientific research by offering new ways to hypothesize, simulate, and innovate.
This blog explores how generative AI is accelerating discovery across disciplines, from molecular biology to climate science, reshaping the future of human knowledge.
Understanding Generative AI in a Scientific Context
At its core, generative AI refers to systems that can create new data outputs based on learned patterns from massive datasets. These models, such as large language models (LLMs), generative adversarial networks (GANs), or diffusion models, have proven their value in creative applications. However, when trained on scientific data—chemical structures, protein sequences, physics equations—they become powerful engines of discovery.
Unlike traditional computational models that rely on explicit programming, generative AI learns underlying relationships in data and then produces novel hypotheses, materials, or simulations that align with those learned rules. In essence, it can generate ideas that scientists might not think of independently, all while working at speeds far beyond human capacity.
Accelerating Drug Discovery and Medical Research
One of the most promising domains for generative AI is pharmaceutical science. The process of discovering new drugs is time-consuming, expensive, and often yields low success rates. AI is poised to revolutionize this process in multiple ways:
Molecule Generation
Generative AI can design entirely new molecules with properties predicted to treat specific diseases. Models like DeepMind’s AlphaFold and Insilico Medicine’s drug design engines can predict how proteins fold or interact with small molecules, helping identify viable drug candidates in weeks rather than years.
For example, by training on large databases of chemical compounds, a model can suggest molecules likely to bond with a disease-relevant protein. Researchers can then synthesize and test only the most promising candidates—greatly reducing time and cost.
Personalized Medicine
AI is also being used to create customized treatment strategies based on a patient’s genetic makeup. Generative models can analyze individual genomes and simulate how various drug compounds might interact with specific mutations, allowing for tailored therapies and minimizing side effects.
Vaccine Development
Generative models helped during the COVID-19 pandemic by accelerating the modeling of virus structures and immune responses. As pathogens evolve, these models can predict future mutations and help design vaccine candidates ahead of time.
Pioneering Materials Science
From superconductors to biodegradable plastics, the materials used in everyday life are constantly evolving. However, designing new materials with desired properties typically requires years of experimentation.
Generative AI is transforming materials science by enabling the virtual creation and testing of compounds before any laboratory work begins.
Atomic-Level Simulations
AI systems trained on databases of atomic configurations and chemical interactions can propose new materials that are lightweight, heat-resistant, or energy-efficient. These models simulate behavior under different conditions, guiding researchers toward the most viable materials for everything from electric vehicle batteries to aerospace applications.
Green Materials
Environmental sustainability is a growing focus in science. Generative AI helps create eco-friendly materials by predicting biodegradable or recyclable alternatives to plastics, reducing the environmental impact of manufacturing.
Exploring the Cosmos and Physics
Physics and astronomy have long relied on computation for modeling phenomena that can’t be observed directly. Generative AI adds a new layer by identifying hidden patterns in massive datasets from telescopes, particle accelerators, and gravitational wave detectors.
Universe Simulation
AI can generate simulations of galactic formations, black hole dynamics, and cosmic inflation by learning from astronomical data. These simulations help scientists test theories of the universe’s origin and structure without the need for massive computing infrastructure.
Quantum Discovery
Generative models can assist in discovering quantum phenomena by modeling interactions in complex systems. This could lead to breakthroughs in quantum computing, energy storage, or encryption technologies.
Climate Science and Environmental Research
Climate modeling requires managing enormous variables—ocean temperatures, greenhouse gas levels, deforestation, and more. Traditional models struggle with precision at local scales.
Generative AI offers a solution by crafting high-resolution climate projections based on current and historical data.
Simulating Climate Futures
Using AI, scientists can simulate various emission scenarios and their long-term effects on weather patterns, sea levels, and biodiversity. These simulations can guide policymaking, helping governments and organizations plan for mitigation and adaptation strategies.
Biodiversity Modeling
AI models trained on species distribution data, habitat loss rates, and climate variables can predict the future of biodiversity in different ecosystems. This helps conservationists prioritize areas for protection or restoration.
Revolutionizing Engineering and Design
In engineering, design processes are iterative and often trial-and-error. Generative AI flips this process by enabling generative design, where models explore countless variations based on input goals like weight, strength, or cost.
Structural Optimization
In architecture, aerospace, and automotive sectors, AI can suggest structural designs that reduce material usage while maintaining strength. These designs are often novel—shapes that no human designer would intuitively create—yet optimized for performance.
Robotics and Automation
Generative AI aids in designing robotic systems by simulating how new body shapes, limb configurations, or sensor placements will affect movement and adaptability. This is especially useful in designing drones, prosthetics, or autonomous vehicles.
Scientific Writing and Knowledge Synthesis
Scientific progress depends not only on experimentation but also on communication and collaboration. Generative language models like GPT-4 and beyond can support research teams by:
- Drafting literature reviews
- Summarizing complex papers
- Translating technical documents
- Generating experimental hypotheses from past studies
These capabilities allow researchers to focus more on analysis and less on documentation, speeding up the research cycle.
Ethical, Practical, and Interpretability Challenges
While the potential is vast, integrating generative AI into scientific workflows comes with concerns that must be addressed carefully.
Reliability and Reproducibility
AI-generated hypotheses or simulations must still be validated through rigorous testing. There’s a risk of models producing plausible but incorrect outputs if they are not trained on diverse or high-quality data.
Interpretability
Scientific work requires transparency. Black-box models can be difficult to interpret, raising concerns about whether researchers can fully trust AI-generated conclusions. Efforts are underway to improve explainable AI in science, but this remains an area needing attention.
Data Bias and Limitations
Scientific datasets may contain biases—missing data from certain populations, underrepresented regions, or outdated information. Models trained on such data can perpetuate or even amplify those biases.
Misuse and Ethics
As AI begins generating powerful discoveries, questions arise about dual-use risks—where knowledge or technologies could be weaponized. Governance, ethical oversight, and responsible publishing must accompany AI-driven science.
The Road Ahead: A New Age of Discovery
Generative AI is not a replacement for human scientists—it is a collaborator, accelerator, and augmentor. It reduces the barriers to discovery, empowers researchers to test bold ideas, and expands the scope of what science can achieve.
We are entering a period where intelligent systems work side by side with human ingenuity, generating novel paths through the dense maze of data, complexity, and uncertainty. This synergy could unlock answers to some of humanity’s deepest questions—about life, the universe, and everything in between.
As AI models continue to evolve, the next wave of Nobel-worthy discoveries may well be co-authored by an algorithm.