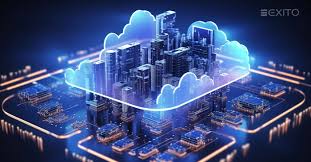
As the digital world continues to evolve, the fusion of edge computing and artificial intelligence (AI) is transforming the way we process, analyze, and utilize data. Edge computing, by processing data near the source of creation, rather than relying on centralized cloud servers, significantly enhances the efficiency, speed, and scalability of AI systems. This convergence of edge computing with AI is revolutionizing various industries, pushing the limits of AI agents and expanding their capabilities.
In this blog, we will explore the impact of edge computing on AI agent capabilities, the key technologies involved, the benefits of combining these two innovations, the challenges faced in their integration, and the future possibilities they hold.
What is Edge Computing?
Edge computing refers to a distributed computing framework that processes data closer to where it is generated, such as on IoT devices, local servers, or edge nodes, instead of relying on distant cloud data centers. This allows for faster processing times, reduced latency, and less reliance on network bandwidth, as data doesn’t need to travel long distances for analysis.
By processing data at the “edge” of the network, close to the source of information, edge computing enables real-time decision-making and minimizes the delay typically experienced when data must be sent to a centralized server. As a result, this approach is particularly beneficial for applications requiring immediate response times, such as autonomous vehicles, industrial automation, smart cities, and AI agents.
The Role of AI in Edge Computing
Artificial intelligence (AI) plays a central role in the growth of edge computing. Traditional AI systems typically rely on powerful data centers to handle processing, which can be slow and inefficient, especially in situations that demand real-time responses. Edge computing, however, allows AI algorithms to run directly on local devices—such as smartphones, sensors, drones, and even industrial machinery—minimizing the need to send large volumes of data to centralized servers.
By bringing AI models closer to the data source, edge computing allows AI agents to perform real-time decision-making, enhancing the capabilities of applications across various fields. The combination of edge computing and AI enables the creation of intelligent, responsive systems that can analyze and respond to data instantaneously without waiting for the cloud to process it.
Enhancing AI Agent Capabilities Through Edge Computing
The integration of edge computing into AI technology has a profound impact on the capabilities of AI agents. Some of the key enhancements are as follows:
1. Faster Response Times
One of the most significant advantages of edge computing is its ability to drastically reduce latency. AI agents operating on the edge can process data in real-time, without the need for round-trip communication with centralized cloud servers. This is especially critical for applications that rely on time-sensitive responses, such as autonomous vehicles, real-time security surveillance, or industrial automation systems.
For example, in the case of autonomous vehicles, the ability to make split-second decisions is paramount for safety. By running AI models locally on the vehicle (edge computing), the vehicle can instantly analyze data from cameras, sensors, and other sources to make driving decisions, without relying on cloud processing, which could introduce dangerous delays.
2. Improved Data Privacy and Security
Edge computing offers a significant advantage when it comes to data privacy and security. Since data is processed locally rather than transmitted to remote data centers, sensitive information does not need to travel over the internet, reducing the risk of data breaches or cyber-attacks.
AI agents operating at the edge can ensure that sensitive data, such as health information from wearable devices or financial transactions, is processed and analyzed securely on the device itself. This local processing helps in complying with privacy regulations such as GDPR (General Data Protection Regulation), as personal data can be anonymized or discarded without leaving the local network.
3. Scalability and Efficiency
Edge computing allows for the scalability of AI applications by distributing processing across a network of devices, rather than relying on a centralized cloud server. This reduces the burden on data centers, making it easier and more cost-effective to deploy AI agents at scale.
By offloading computations to edge devices, businesses can distribute workloads efficiently and minimize the bottlenecks that would otherwise occur in a centralized architecture. For example, AI-powered smart devices in a smart home can work independently, making decisions locally without needing constant communication with the cloud, which helps in managing large-scale deployments.
4. Reduced Bandwidth Consumption
Another key benefit of edge computing is the reduction in bandwidth requirements. By processing data locally, edge devices can significantly minimize the need to send large amounts of raw data to the cloud. This helps conserve network bandwidth and ensures that only relevant or processed information is sent for further analysis or storage.
For AI agents in applications such as video surveillance or environmental monitoring, where massive amounts of sensor data are generated, the ability to filter and process data on the edge before transmitting it to the cloud is a game-changer. This not only reduces network congestion but also makes the overall system more efficient.
5. Enhanced Reliability and Fault Tolerance
Edge computing enhances the reliability of AI agents by reducing their dependency on centralized servers. In environments where connectivity to the cloud is unstable or intermittent, AI agents running on edge devices can continue to operate without disruption. This is particularly important in remote or rural areas where network infrastructure may be unreliable.
In industrial settings, for instance, edge computing allows AI agents to control machinery, monitor equipment performance, and detect anomalies in real-time, even if there is a temporary disconnection from the cloud. This leads to increased reliability and ensures continuous operation without downtime.
Use Cases of Edge Computing in AI Agents
The fusion of edge computing and AI is already being leveraged in a wide range of industries, transforming business models and enabling more intelligent, autonomous systems. Some of the most notable use cases include:
1. Autonomous Vehicles
Autonomous vehicles, such as self-driving cars and drones, rely heavily on real-time data processing to navigate and make decisions. Edge computing enables AI agents in these vehicles to process data from cameras, LiDAR, radar, and other sensors in real-time, allowing for immediate decision-making that is critical to ensuring safety and efficiency.
By processing data locally, autonomous vehicles can detect obstacles, calculate routes, and respond to traffic conditions almost instantaneously, without relying on cloud-based systems.
2. Smart Cities
In the context of smart cities, edge computing is revolutionizing urban management systems. From traffic management and energy distribution to waste management and public safety, AI-powered edge devices are collecting and processing data from sensors throughout the city. For example, AI agents in smart traffic lights can adjust signal timings in real-time based on traffic flow, improving efficiency and reducing congestion.
Moreover, edge computing ensures that sensitive data, such as surveillance footage or environmental data, is processed locally to maintain privacy and avoid unnecessary data transfers to remote servers.
3. Healthcare Monitoring
AI-powered healthcare devices, such as wearable health trackers, benefit from edge computing by processing patient data locally on the device itself. These devices can continuously monitor vital signs, detect irregularities, and alert healthcare providers in real-time without needing to send sensitive data to the cloud.
For instance, AI agents embedded in smartwatches or fitness trackers can detect early signs of health issues, such as irregular heart rhythms, and provide immediate feedback or warnings to the user or their healthcare provider.
4. Industrial IoT (IIoT)
Edge computing is playing a pivotal role in the Industrial Internet of Things (IIoT), where AI agents are deployed in manufacturing plants, warehouses, and factories to monitor and control machinery. These AI agents can detect equipment malfunctions, predict failures, and optimize processes by processing data from sensors and machines in real-time, without relying on cloud servers.
This localized data processing not only improves operational efficiency but also ensures that industries can maintain continuous production with minimal downtime.
Challenges of Integrating Edge Computing with AI
While the potential of combining edge computing with AI is immense, there are several challenges to overcome:
1. Limited Computational Power
Edge devices often have limited computational power compared to centralized cloud servers. AI models, especially deep learning models, require significant processing capabilities, which can be challenging to deploy on devices with limited resources. Developers must create lightweight, optimized AI models that can run efficiently on edge devices without compromising performance.
2. Data Management and Coordination
Managing data across a distributed network of edge devices can be complex. With data being processed locally, ensuring consistency, synchronization, and coordination across different devices becomes a challenge. Data from edge devices may need to be aggregated and analyzed in the cloud for higher-level insights, requiring seamless integration between edge and cloud systems.
3. Security and Privacy
While edge computing offers improved security by processing data locally, it also introduces new risks. Devices at the edge can be vulnerable to cyberattacks, and maintaining the security of large-scale distributed systems requires robust measures. Ensuring the privacy of data processed on edge devices, especially when it comes to sensitive information, remains a critical concern.
The Future of AI Agents and Edge Computing
The combination of AI and edge computing is poised to transform countless industries, and as technology continues to evolve, we can expect even more advanced capabilities. With the growing demand for real-time decision-making and privacy-conscious applications, edge computing will become an essential component of AI agent systems.
In the future, we may see even smarter AI agents capable of running more sophisticated models locally, improving their ability to make complex decisions with minimal latency. As edge computing infrastructure becomes more robust and affordable, AI agents will become more autonomous, efficient, and reliable, ushering in a new era of intelligent, connected systems.