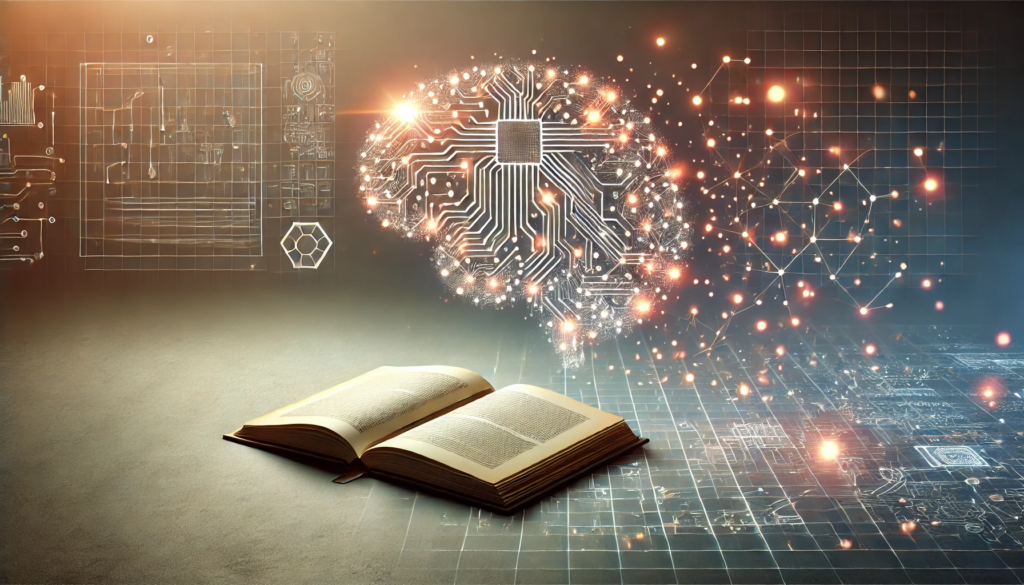
Artificial Intelligence (AI) is no longer a futuristic concept limited to science fiction. It has become a transformative force across industries, particularly in the domain of Research and Development (R&D). From accelerating discovery cycles to enabling intelligent data analysis, AI is reshaping how innovation occurs.
R&D is the lifeblood of progress in sectors such as pharmaceuticals, manufacturing, energy, healthcare, and technology. However, traditional research methods often involve lengthy trial-and-error processes, complex data interpretation, and significant resource allocation. AI offers a powerful solution to these challenges by automating repetitive tasks, uncovering hidden patterns in data, and enhancing the efficiency and accuracy of experimentation.
In this blog, we explore the multifaceted impact of AI on R&D, including its applications, benefits, limitations, and future potential.
Understanding the Role of AI in R&D
Artificial Intelligence refers to the use of machines and algorithms that can perform tasks typically requiring human intelligence. This includes learning from data (machine learning), understanding natural language, recognizing images, and making predictions.
In R&D, AI helps researchers move from hypothesis-based investigation to data-driven discovery. Instead of spending years formulating and testing theories manually, AI systems can simulate outcomes, analyze massive datasets, and recommend promising directions for exploration.
AI’s involvement in R&D spans multiple areas:
- Data processing and analysis
- Predictive modeling
- Simulation and prototyping
- Automated experimentation
- Knowledge extraction
Applications of AI in Research and Development
1. Accelerated Drug Discovery
In pharmaceutical research, AI is transforming drug discovery by predicting how different compounds interact with biological targets. Traditionally, this process takes years of laboratory testing. AI shortens this timeline by:
- Identifying potential drug candidates using machine learning models
- Simulating molecular interactions with AI-driven computational chemistry
- Repurposing existing drugs for new diseases based on pattern recognition
For example, during the COVID-19 pandemic, AI systems helped identify potential antiviral compounds much faster than traditional methods would have allowed.
2. Materials Science and Engineering
AI aids in the discovery of new materials by modeling atomic structures and simulating their properties. Instead of physically testing every possible combination, AI narrows down candidates likely to meet the desired criteria, such as strength, conductivity, or heat resistance.
This has been instrumental in fields like aerospace, renewable energy, and electronics, where material performance is critical.
3. Agricultural Research
In agritech R&D, AI helps optimize crop yields, improve soil management, and enhance plant breeding. Researchers use AI to analyze satellite imagery, monitor plant health, and model climate impacts.
AI-driven genetic analysis also supports the development of drought-resistant or pest-tolerant crop varieties, ensuring food security under changing environmental conditions.
4. Product Design and Prototyping
AI-driven generative design software can automatically create thousands of product design iterations based on specified parameters. These tools use machine learning to refine designs and simulate performance, significantly reducing the time needed to prototype and test products.
This is particularly useful in industries like automotive, consumer electronics, and industrial manufacturing.
5. Scientific Research and Literature Review
AI can process vast quantities of scientific literature to identify relevant studies, summarize findings, and recommend unexplored areas of research. Natural language processing (NLP) tools help researchers stay current with the ever-growing body of scientific knowledge.
This accelerates hypothesis formulation and ensures that R&D efforts are not duplicated unnecessarily.
6. Energy Sector Innovation
In energy research, AI is used to model consumption patterns, optimize grid operations, and simulate the behavior of renewable energy systems. AI can also help design more efficient batteries and energy storage solutions by predicting chemical behaviors under various conditions.
Key Benefits of AI in R&D
1. Faster Time to Innovation
AI automates complex tasks and simulations that would take humans months or even years to complete. This drastically reduces development timelines and allows organizations to bring new products to market more quickly.
2. Cost Efficiency
AI reduces the need for physical prototypes, excessive trials, and redundant research. It allows companies to allocate resources more strategically, making innovation more affordable.
3. Enhanced Accuracy and Precision
AI systems excel at detecting patterns and correlations in data that may be imperceptible to humans. This leads to more accurate predictions and reduces the risk of human error.
4. Scalability
AI allows researchers to conduct experiments and simulations at scale. What once required a full laboratory team can now be done by a few experts supported by AI-driven platforms.
5. Cross-Disciplinary Innovation
AI promotes interdisciplinary research by integrating knowledge across biology, chemistry, physics, and data science. This synergy fuels breakthroughs that may not be possible through siloed approaches.
Challenges and Limitations
Despite its advantages, integrating AI into R&D is not without challenges.
1. Data Availability and Quality
AI models require large, high-quality datasets to function effectively. In many research areas, especially emerging ones, such data may be limited or unstructured.
2. Interpretability and Trust
AI models, particularly deep learning systems, can be difficult to interpret. Researchers and regulators may be hesitant to trust results that cannot be easily explained or validated.
3. Ethical and Regulatory Concerns
In fields such as biotechnology or medicine, using AI raises ethical questions about bias, consent, and accountability. Regulatory frameworks often lag behind the rapid pace of technological change.
4. High Implementation Costs
While AI can reduce long-term costs, the initial investment in infrastructure, training, and integration can be substantial, particularly for smaller organizations.
5. Dependence on Human Expertise
AI is a tool, not a replacement for researchers. Human oversight is crucial to guide AI systems, verify results, and ensure ethical research practices.
The Future of AI in R&D
As AI continues to evolve, its impact on R&D will deepen. Future developments may include:
- Autonomous Research Labs: Facilities where AI systems run experiments, adjust parameters, and analyze results with minimal human intervention.
- Collaborative AI: Tools that work alongside researchers in real-time, suggesting hypotheses, designing experiments, and interpreting outcomes.
- AI-Driven Innovation Platforms: Centralized systems where cross-functional teams from different companies or institutions collaborate using shared AI resources.
- Democratization of R&D: Cloud-based AI tools may make sophisticated research capabilities accessible to startups and academic institutions without extensive funding.
- Human-AI Co-Creation: AI systems that can partner with researchers to ideate, design, and refine products in a creative and iterative way.
Industry Case Studies
IBM’s Project Debater
IBM has developed an AI system capable of forming arguments, counterarguments, and conclusions based on vast amounts of scientific text. Such systems are used to support research teams in policy-making and academic institutions.
Google DeepMind’s AlphaFold
AlphaFold made a breakthrough in biology by accurately predicting protein structures, a long-standing challenge in science. This has immense implications for drug development, genetics, and molecular biology.
Siemens’ Generative Design
Siemens uses AI to automate the design of industrial components, drastically improving efficiency and performance. This has helped reduce material costs and accelerate the development cycle in manufacturing.
Conclusion
Artificial Intelligence is not just enhancing Research and Development—it is redefining it. By enabling faster discovery, smarter experimentation, and deeper insights, AI is accelerating progress across industries. While there are challenges to overcome, the potential rewards of AI-driven R&D are enormous.
Organizations that embrace AI in their research initiatives are better positioned to innovate, adapt, and lead in the evolving global economy. As tools become more accessible and integrated, AI will play a central role in shaping the future of innovation.