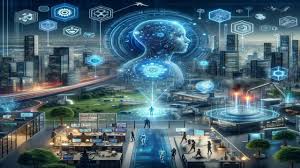
Generative AI has emerged as one of the most exciting technological advancements in recent years. From creating realistic images and videos to generating human-like text, the potential of generative AI is vast. However, the ability to run these complex models at scale requires significant computational power. This is where generative AI hardware plays a critical role. As the demand for AI models increases, there is a growing need for hardware that can handle the intense computational load required to train and run generative AI models efficiently.
In this blog, we will explore the future of generative AI hardware, focusing on the innovations and trends that are shaping the hardware landscape. From specialized processors to the integration of quantum computing, we will delve into the technologies that are set to transform the way generative AI models are built and deployed.
Current Generative AI Hardware
Before diving into the future, it’s essential to understand the current hardware landscape for generative AI. Most generative AI models, such as those used in deep learning and neural networks, rely on processors that are capable of handling massive parallel computation.
Graphics Processing Units (GPUs)
The backbone of current generative AI hardware is the Graphics Processing Unit (GPU). Originally designed for rendering graphics in video games, GPUs are well-suited for AI workloads due to their ability to perform many calculations simultaneously. The parallel processing power of GPUs allows them to train complex models faster than traditional CPUs.
NVIDIA has long been a leader in the AI hardware space, with its GPUs being widely used for deep learning tasks. The A100 and V100 GPUs, for example, have become standard for training AI models, including generative ones. GPUs have proven their value in running large-scale deep learning models such as GPT (Generative Pre-trained Transformer) and DALL·E.
Tensor Processing Units (TPUs)
Another significant development in AI hardware is Google’s Tensor Processing Unit (TPU). TPUs are specifically designed for machine learning tasks and are optimized for tensor operations, which are essential for neural network training. Google has leveraged TPUs for their cloud-based machine learning services, allowing developers to accelerate their models’ performance and scalability.
While GPUs are great for general-purpose deep learning, TPUs offer an edge in terms of specific optimizations for AI workloads, making them highly efficient for tasks like generative modeling, where matrix multiplications and tensor operations are central.
FPGAs and ASICs
Field-Programmable Gate Arrays (FPGAs) and Application-Specific Integrated Circuits (ASICs) are also important in the AI hardware ecosystem. These devices offer customizable solutions for specific AI workloads, including generative AI tasks. FPGAs are reprogrammable and can be tailored to accelerate specific algorithms, while ASICs are custom-built for optimal performance in specific use cases. While they are not as widely used as GPUs or TPUs, they offer unique benefits in terms of power efficiency and speed when properly implemented.
The Future of Generative AI Hardware
As generative AI models continue to grow in complexity, the hardware required to run these models must evolve. The future of generative AI hardware is likely to be characterized by several key trends and innovations that will change the landscape of AI computing.
1. Specialized AI Chips
One of the most significant trends in the future of generative AI hardware is the development of specialized AI chips. These chips will be optimized specifically for the unique needs of AI models, including generative models, and will be tailored to the computational requirements of deep learning algorithms.
AI Accelerators
AI accelerators are purpose-built processors designed to speed up machine learning workloads. These chips are expected to become more common as AI continues to push the boundaries of what is possible. Companies like Intel, AMD, and Google are investing heavily in the development of AI accelerators that will outperform traditional processors in specific machine learning tasks.
For instance, Google’s Tensor Processing Units (TPUs) have already shown that specialized hardware can dramatically increase the performance of generative AI models. Moving forward, we can expect more companies to develop custom AI accelerators that are capable of handling the unique requirements of generative AI, such as image generation, natural language processing, and video synthesis.
2. Quantum Computing and Generative AI
Quantum computing is a rapidly emerging field that holds the promise of revolutionizing how we approach computation, including AI workloads. Unlike traditional computers that use bits to represent data, quantum computers use quantum bits (qubits), which can represent multiple states simultaneously due to the principles of quantum mechanics.
This inherent parallelism in quantum computing makes it an exciting candidate for accelerating AI tasks, particularly generative AI. Quantum computers have the potential to process exponentially more data at once, which could drastically reduce the time it takes to train generative AI models. Moreover, quantum computing could enable more advanced models that are currently computationally impractical on classical hardware.
While quantum computing for generative AI is still in its infancy, companies like IBM, Google, and Microsoft are making significant strides in building quantum processors that could one day be used to power AI models. In the future, we may see quantum computing used to generate more realistic content, simulate complex systems, or even train AI models on vast datasets that are currently beyond the capabilities of classical computing.
3. Neuromorphic Computing
Neuromorphic computing is another exciting frontier for generative AI hardware. Neuromorphic chips are inspired by the human brain and are designed to mimic the way biological neurons process information. These chips are highly parallel and energy-efficient, making them ideal for running complex AI models, including generative ones.
The brain-like architecture of neuromorphic chips allows them to perform computations in a way that is more similar to how humans think, potentially leading to more efficient and faster AI models. Neuromorphic computing has the potential to accelerate generative AI workloads, especially in applications that require real-time decision-making and pattern recognition, such as autonomous vehicles and robotics.
4. Edge AI Hardware
Edge computing is the practice of processing data locally on devices rather than relying on cloud-based systems. This trend is expected to have a significant impact on generative AI, particularly in applications where low latency and real-time processing are critical, such as in autonomous vehicles, robotics, and augmented reality.
Edge AI hardware will play a key role in this shift. Devices like smartphones, drones, and IoT sensors are already being equipped with powerful AI chips that can run generative AI models locally. This enables faster processing and reduces the need for sending large amounts of data to the cloud for inference. In the future, we can expect to see more specialized AI chips for edge devices that are capable of running generative models, allowing for real-time content generation and on-the-fly AI decision-making.
5. Power Efficiency and Sustainability
As generative AI models grow in size and complexity, the demand for computational power will only increase. However, this increased computational demand also comes with a significant environmental cost. Training large AI models requires substantial energy, and running these models at scale can lead to higher carbon emissions.
To address these concerns, future generative AI hardware will need to focus on power efficiency and sustainability. Companies are already exploring ways to reduce the energy consumption of AI chips, including using more energy-efficient manufacturing processes, optimizing hardware designs, and incorporating renewable energy sources into AI data centers.
6. Collaborative and Open-Source AI Hardware Development
As AI hardware development becomes more specialized, there is an increasing trend toward collaboration and open-source initiatives. Companies are working together to create standardized hardware platforms that can be used across a variety of AI models. Open-source hardware and software ecosystems will enable a more inclusive and innovative approach to AI development.
In the future, we may see more collaborative efforts between hardware manufacturers, AI researchers, and cloud providers to create hardware platforms optimized for generative AI. This would make it easier for researchers and developers to access the necessary resources for building and deploying generative AI models.
Conclusion
The future of generative AI hardware is poised for significant innovation. As AI models grow in complexity, new specialized hardware, quantum computing, neuromorphic computing, and edge AI solutions will emerge to meet the demands of generative AI workloads. These advancements will enable faster, more efficient model training and inference, as well as make generative AI more accessible and scalable across various industries.
While we are still in the early stages of many of these developments, the future is bright for generative AI hardware. The combination of more powerful and specialized processors, the potential of quantum computing, and the focus on energy efficiency will help unlock the full potential of generative AI, bringing us closer to a world where machines can create, innovate, and generate content at an unprecedented scale.