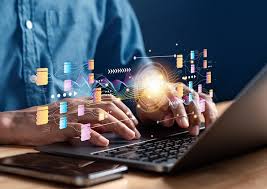
Artificial Intelligence (AI) has rapidly evolved from niche academic theory to an essential force in everyday technologies. Among the most transformative advances in recent years is multimodal generative AI—systems capable of understanding and producing multiple forms of data such as text, images, audio, and video simultaneously. This marks a significant shift from the early days of narrow, single-modal AI, pushing the boundaries of creativity, automation, and human-computer interaction.
Understanding Multimodal Generative AI
At its core, multimodal AI processes and combines different types of inputs—words, visuals, sounds, and more—mirroring the way humans perceive and interact with the world. Traditional AI systems typically specialize in one domain: natural language processing (NLP) systems generate or analyze text, while computer vision models handle images. Multimodal generative AI breaks these silos, enabling models to understand connections across modalities. For example, a user might input an image and ask for a poetic description, or provide a sound clip to generate an animated scene.
The generative aspect refers to the model’s capacity to create new content, not just classify or analyze it. Whether composing music, illustrating stories, or simulating conversations between fictional characters, these systems leverage their learned representations of multiple modalities to produce novel outputs.
A Brief History of Generative AI
The path to today’s multimodal models was paved by developments in generative AI, particularly with text and image data. Early rule-based systems attempted to generate language, but it wasn’t until neural networks—and later deep learning—that real progress began. The emergence of Generative Adversarial Networks (GANs) in 2014 allowed AI to generate photorealistic images by training two networks in opposition: one to generate content and another to critique it.
In parallel, transformers revolutionized NLP with the advent of models like BERT and GPT. These architectures allowed models to grasp context and semantics in language more effectively, laying the groundwork for models that could understand and generate fluent text.
Soon, researchers began experimenting with combining text and vision. OpenAI’s CLIP (Contrastive Language–Image Pretraining) and DALL·E demonstrated that a unified model could relate visual and textual concepts. Rather than training separate networks, these models used joint embedding spaces where text and images shared conceptual meaning.
Key Milestones in Multimodal Development
- CLIP (2021): OpenAI trained CLIP to understand images in the context of natural language, enabling zero-shot classification across diverse image types using just textual prompts.
- DALL·E and DALL·E 2 (2021–2022): These models generated creative images from natural language descriptions, opening up possibilities in design, advertising, and education.
- Imagen and Parti (Google, 2022): These models pushed the fidelity of text-to-image generation further, competing with DALL·E by producing more detailed and accurate visuals.
- Make-A-Video and Phenaki (Meta and Google, 2022–2023): These systems introduced video generation from text, blending frames with coherent motion and visual narrative.
- GPT-4 with vision (2023–2024): OpenAI extended its flagship language model to analyze and respond to images, paving the way for deeper integration between text and vision.
- Sora (2024): OpenAI’s video-generating model, capable of producing realistic, dynamic scenes from textual input, exemplified the peak of multimodal generation at the time.
How Multimodal AI Works
Multimodal AI models are trained on enormous datasets containing paired inputs—text with images, videos with captions, audio with transcripts, and so on. They use shared representation spaces, where concepts are not limited to one type of data. For example, the concept of a “cat” is represented similarly whether it appears as an image, is spoken aloud, or described in text.
This unified learning is possible through transformers—especially multimodal transformers—which attend to different inputs concurrently and establish relationships between them. This allows models to answer complex queries like, “What is happening in this image?” or “Generate a short story about the scene in this photo.”
Real-World Applications
The potential applications of multimodal generative AI span industries and disciplines:
- Healthcare: Analyzing medical images while considering patient history or clinical notes to suggest diagnoses.
- Education: Creating interactive textbooks where students can ask questions about images or diagrams.
- Entertainment: Producing movies, animations, or music videos from scripts or simple sketches.
- Accessibility: Assisting visually impaired users by describing scenes or reading signs using camera input.
- Retail: Designing fashion products from verbal descriptions or converting sketches into product prototypes.
Benefits of Multimodal Generative AI
- More Natural Interaction: It enables intuitive interfaces where users can speak, draw, type, or show something and receive intelligent, context-aware responses.
- Creative Empowerment: Artists, writers, and designers can collaborate with AI as co-creators, enhancing their productivity and exploring new styles or mediums.
- Improved Understanding: By synthesizing data from multiple sources, models can form a more holistic understanding of situations, reducing ambiguity and improving accuracy.
Ethical Considerations and Challenges
Despite its promise, multimodal AI introduces new ethical complexities:
- Bias and Representation: Training data may encode social biases, which can appear in generated content. Ensuring diversity and fairness in multimodal datasets is challenging.
- Deepfakes and Misinformation: Generating highly realistic images or videos can be exploited to spread false narratives or impersonate individuals.
- Data Privacy: Multimodal systems often require access to sensitive data, such as personal images or voice recordings, which raises serious privacy concerns.
- Energy and Compute Cost: Training these massive models consumes significant computational resources, contributing to environmental concerns.
Addressing these issues requires transparency in development, robust safety testing, and collaborative governance among developers, policymakers, and the public.
The Road Ahead
As the field matures, several trends are likely to define the next era of multimodal AI:
- Universal Multimodal Models: Future systems may seamlessly integrate text, image, audio, and video with minimal training or fine-tuning.
- Edge Deployment: Optimized models will run on mobile and wearable devices, enabling real-time interaction in offline or bandwidth-limited environments.
- Neurosymbolic Integration: Combining deep learning with symbolic reasoning could improve interpretability and reasoning in multimodal contexts.
- Personalization: Future generative systems may adapt to individual users’ preferences, styles, or contexts, offering highly customized experiences.
Conclusion
Multimodal generative AI represents a profound leap toward more human-like intelligence in machines. By enabling computers to understand and generate diverse forms of data, these systems are reshaping creativity, productivity, and communication. While the journey is still unfolding, the convergence of multiple modalities into a cohesive, generative intelligence marks one of the most exciting frontiers in technology today.
As with all powerful tools, the key lies not only in what they can do but in how we choose to use them—responsibly, inclusively, and imaginatively.