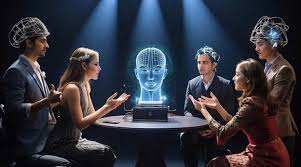
As artificial intelligence (AI) evolves from a supportive tool to an independent decision-making agent, ethical concerns are becoming increasingly complex and urgent. Autonomous AI agents—systems capable of acting without human input—are transforming sectors ranging from healthcare to finance, transportation to security. These agents can diagnose diseases, approve loans, manage inventory, and even operate military drones. With such responsibility comes an equally critical question: how should we govern the ethical behavior of AI agents?
Autonomous decision-making by machines introduces scenarios previously reserved for human judgment. This shift demands a thorough investigation into the moral, legal, and philosophical frameworks guiding AI behavior. Who is responsible when an AI makes a mistake? Can a machine be taught empathy or fairness? Should AI agents have constraints on autonomy? This blog explores these pressing questions and highlights the ethical dimensions involved in deploying autonomous AI systems.
Understanding Autonomous AI Agents
Autonomous AI agents are self-operating entities that sense their environment, make decisions based on learned rules or models, and act toward achieving goals. Unlike basic automation, these agents:
- Learn and adapt over time (via machine learning)
- Operate with minimal or no human oversight
- Make decisions in uncertain or dynamic environments
Such capabilities enable AI agents to control self-driving vehicles, analyze surveillance feeds, respond to cybersecurity threats, and handle customer queries independently.
While autonomy increases efficiency and scalability, it also means humans relinquish some control. This transition prompts us to rethink accountability, safety, and values in the digital age.
Core Ethical Challenges of Autonomous AI
1. Accountability and Responsibility
When an AI agent makes a harmful or incorrect decision, determining responsibility is difficult. For instance:
- If a self-driving car crashes, is the carmaker, software developer, or user at fault?
- When a medical AI recommends a wrong treatment, who answers for the consequence?
Currently, legal systems are designed to hold humans accountable. But autonomous agents blur the lines between tool and actor. Without clear regulations, society risks facing a gap in responsibility.
2. Bias and Discrimination
AI systems trained on historical data can reflect and amplify societal biases. This becomes especially dangerous when agents make decisions about:
- Hiring and employment
- Criminal sentencing or parole
- Loan approvals
- Access to healthcare
An AI agent might unknowingly favor one group over another, perpetuating systemic injustice. Ethical deployment requires not just technical accuracy but fairness, inclusivity, and ongoing bias detection.
3. Transparency and Explainability
AI systems—especially deep learning models—can be opaque or inscrutable, even to their creators. Known as the “black box” problem, this lack of clarity prevents users from understanding:
- How a decision was made
- What data influenced the outcome
- Whether the process was fair
Explainable AI (XAI) is a growing field aimed at making machine decisions more understandable. Transparency is essential for trust, especially in sensitive domains like law, medicine, and finance.
4. Consent and Autonomy
As AI systems make more decisions for humans, questions about user autonomy arise. For instance:
- Is it ethical for an AI to make healthcare decisions without patient input?
- Should an algorithm override human judgment in safety-critical scenarios?
Maintaining human agency is vital. AI should augment rather than replace decision-making where personal freedom, dignity, or values are at stake.
5. Moral Dilemmas and Value Alignment
AI agents may face ethical dilemmas similar to the classic “trolley problem,” where any action leads to harm. For example:
- Should a self-driving car prioritize passengers or pedestrians in an unavoidable crash?
- Can an AI military drone decide whether a target is justified?
Programming moral reasoning into machines is not straightforward. Cultural, contextual, and philosophical differences make universal ethics difficult to codify. Value alignment—ensuring AI shares human ethical standards—is still an unsolved challenge.
6. Manipulation and Surveillance
AI agents can be used to manipulate behavior through personalized content, nudging, and psychological targeting. Additionally, AI-driven surveillance systems can track individuals without their knowledge. These uses raise ethical questions about:
- Informed consent
- Privacy rights
- Free will
Striking a balance between utility and personal rights is essential to prevent abuse.
Ethical Frameworks for AI Agents
To guide ethical decision-making, several frameworks have been proposed and adopted in part by policymakers and organizations.
1. Utilitarianism
This approach suggests that AI should maximize overall happiness or minimize harm. While efficient in theory, it can justify morally questionable outcomes if they benefit the majority.
2. Deontology
Deontological ethics emphasizes duties and rules over outcomes. For instance, an AI system should always tell the truth or avoid causing harm, regardless of the benefits. This ensures consistency but can be rigid.
3. Virtue Ethics
Here, the focus is on cultivating moral character and ethical virtues like honesty, compassion, and courage. While difficult to program, this model encourages human-like ethical reasoning in AI.
4. Rights-Based Ethics
This approach centers on protecting individual rights, such as privacy, freedom, and equality. AI systems guided by this principle prioritize user dignity and legal protections.
5. Ethical AI Principles (Proposed by Institutions)
Organizations like the EU Commission, IEEE, and OECD have outlined ethical principles, including:
- Accountability
- Transparency
- Non-maleficence (do no harm)
- Justice and fairness
- Human oversight
Though these principles are widely accepted, implementation varies across countries and industries.
Case Studies and Real-World Implications
Self-Driving Cars
Autonomous vehicles are a prime example of AI making life-or-death decisions. In 2018, a self-driving Uber vehicle struck and killed a pedestrian. The incident sparked debate about software safety, human supervision, and moral judgment.
Should an AI prioritize vehicle occupants, pedestrians, or the “greater good”? Without global consensus, different companies adopt different policies, creating inconsistency and confusion.
Facial Recognition and Law Enforcement
AI-driven facial recognition systems have led to wrongful arrests and privacy violations. In some cases, the technology has misidentified individuals—especially people of color—due to biased training data. Ethical concerns include:
- Consent and awareness
- Racial profiling
- Mass surveillance
Many cities have since banned or restricted the use of such systems, citing ethical risks.
AI in Hiring
Firms use AI agents to screen resumes, conduct interviews, and assess personality traits. However, studies have shown that these systems can inherit gender and racial biases. Ethical AI in recruitment requires transparency, fairness audits, and the right to human review.
The Role of Governance and Regulation
To ensure ethical deployment of AI agents, regulation is essential. Governments, companies, and international bodies must collaborate to:
- Create accountability frameworks
- Mandate bias testing and reporting
- Enforce transparency standards
- Protect data privacy
- Establish liability laws for autonomous actions
The EU’s AI Act, for instance, categorizes AI systems by risk level and imposes stricter rules on high-risk applications. Countries like Canada, Japan, and the U.S. are also developing their own ethical guidelines and legislation.
Designing Ethical AI from the Ground Up
The most effective way to ensure ethical AI behavior is to embed values directly into system design. This requires:
- Diverse teams of developers, ethicists, and users
- Ethical risk assessments during development
- Continuous monitoring and updates
- Robust feedback loops with stakeholders
Rather than treating ethics as an afterthought, it must become integral to the AI development lifecycle.
The Human Element
Despite their growing capabilities, AI agents should never replace human ethical judgment. Ethical challenges often require empathy, context, and cultural sensitivity—traits that AI has yet to replicate. Instead of creating autonomous decision-makers in isolation, humans and machines should collaborate. Hybrid systems that combine AI efficiency with human conscience may offer the best path forward.
Conclusion
The ethics of autonomous AI agents and decision-making is not a theoretical concern—it is a practical necessity as machines increasingly influence human lives. From driverless cars to healthcare algorithms, AI decisions must be fair, transparent, and accountable.
By embedding ethics into design, regulation, and implementation, we can harness the power of AI without compromising human values. While no system can be perfectly ethical, deliberate and inclusive efforts can ensure that AI serves society, not the other way around.
The road ahead is complex, but through collaboration and thoughtful governance, we can build a future where intelligent systems act not just smartly, but rightly.