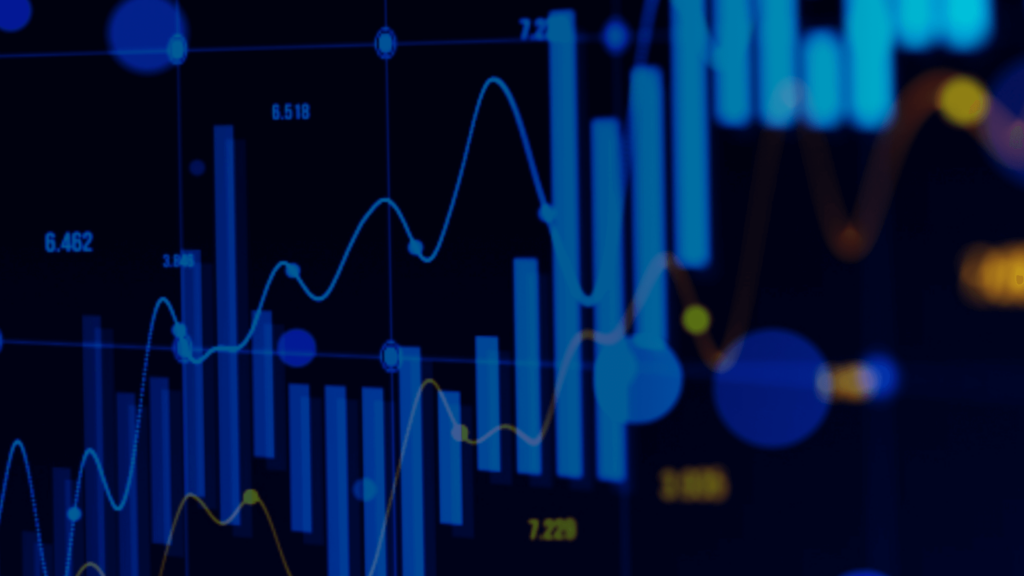
Artificial Intelligence (AI) is rapidly transforming business operations across every industry. From automating repetitive tasks to generating customer insights and predicting market trends, AI offers tremendous value. However, realizing this value requires more than enthusiasm—it demands a well-thought-out investment strategy.
For most businesses, one of the biggest questions is: how much does AI integration cost, and how do we measure the return on that investment (ROI)? This article dives deep into the financial side of AI, providing a detailed guide on budgeting, understanding cost components, and conducting ROI analysis.
Understanding the Financial Landscape of AI Integration
Unlike traditional software tools, AI systems involve unique complexities. These include data handling, model training, infrastructure provisioning, testing, deployment, and ongoing model maintenance. The costs are rarely limited to one department and can stretch across IT, operations, HR, and legal.
Why Cost Estimation Is Challenging:
- AI use cases vary significantly between businesses.
- Hidden costs (such as data preparation or user training) are often overlooked.
- ROI can be indirect or delayed.
To build a realistic budget, it’s essential to break down the cost components and understand where expenses accumulate over the AI lifecycle.
Major Cost Components of AI Integration
1. Data Acquisition and Preparation
AI needs vast and relevant data to function. Gathering, cleaning, and structuring this data is often the most resource-intensive phase.
Costs Include:
- Purchasing third-party datasets (if internal data is insufficient)
- Hiring data engineers or analysts
- Data labeling and annotation
- Implementing data governance and privacy protocols
Tip: Data quality directly influences model performance—cutting corners here often leads to failure later.
2. Infrastructure and Tools
You’ll need hardware and software to build, train, and deploy AI models.
Costs Include:
- Cloud computing (e.g., AWS, Azure, Google Cloud)
- On-premise servers or GPUs (if data privacy is a concern)
- Licenses for AI platforms, libraries, and tools
- API access fees from AI service providers
Tip: Consider starting with cloud-based AI services to minimize upfront capital expenditures.
3. Model Development and Testing
Whether you’re building custom AI models or customizing off-the-shelf solutions, development will require expert input.
Costs Include:
- Salaries for data scientists, ML engineers, and AI architects
- Consulting fees if working with AI vendors or agencies
- Sandbox environments for testing models
- A/B testing and validation
Tip: Use pre-trained models for common tasks like image classification or sentiment analysis to reduce development time.
4. Integration and Deployment
AI models must be integrated into your existing systems and workflows—this step is often underestimated.
Costs Include:
- Custom APIs and middleware development
- Modifying legacy systems
- DevOps and containerization
- Testing across different environments
Tip: Collaborate with IT early to ensure compatibility and reduce integration issues.
5. Training and Change Management
AI adoption is not only technical but also cultural. Employees must learn to work with AI tools effectively.
Costs Include:
- Employee training and upskilling programs
- Internal workshops and onboarding resources
- Change management consultants
- Stakeholder engagement and communication
Tip: Budget for both technical training and change management to maximize adoption.
6. Maintenance and Monitoring
AI systems need constant monitoring and updates to maintain accuracy and compliance.
Costs Include:
- Continuous model retraining
- Drift detection systems
- Data storage and refresh
- Compliance audits (especially in regulated industries)
Tip: Set aside at least 15–20% of the initial implementation cost for annual maintenance.
Sample AI Budget Breakdown (for a mid-sized project)
Cost Component | Estimated Range (USD) |
---|---|
Data Collection & Prep | $25,000 – $50,000 |
Tools & Infrastructure | $20,000 – $100,000 |
Development & Testing | $50,000 – $150,000 |
Integration & Deployment | $30,000 – $70,000 |
Training & Change Mgmt | $10,000 – $30,000 |
Ongoing Maintenance | $15,000 – $40,000/year |
Total Initial Budget | $150,000 – $400,000 |
Costs vary based on project scope, data needs, and industry requirements.
Conducting ROI Analysis for AI Projects
While budgeting is about controlling costs, ROI analysis ensures that the investment delivers value. ROI measurement in AI can be challenging due to its mix of tangible and intangible benefits.
1. Define What Success Looks Like
Start by aligning your AI initiative with specific business goals. Examples include:
- Reducing customer churn
- Increasing operational efficiency
- Improving lead conversion
- Decreasing manual processing time
2. Estimate Financial Benefits
Translate business goals into measurable financial outcomes.
Examples:
- Automating data entry saves 500 employee hours/month = ~$25,000/year
- AI-driven upselling increases sales conversion by 10% = ~$150,000 in extra revenue
- Predictive maintenance reduces downtime = $100,000/year saved
3. Calculate ROI
Use this formula:
ROI (%) = [(Total Benefits – Total Costs) / Total Costs] × 100
Example:
- Total Benefits: $250,000/year
- Total Costs: $150,000 (initial) + $30,000 (annual maintenance)
- Year 1 ROI = [($250,000 – $180,000) / $180,000] × 100 = 38.8%
Note: ROI typically improves in later years as the initial investment amortizes.
4. Factor in Intangible and Strategic Benefits
Not all returns are purely financial. Consider:
- Competitive advantage
- Faster decision-making
- Enhanced customer experience
- Improved data culture and digital maturity
These may not show up on balance sheets but still deliver long-term value.
5. Set a Time Frame for ROI
AI projects may take 6–18 months to break even, depending on their scope. Ensure stakeholders are aligned on when to expect ROI.
Short-term ROI Examples:
- Customer service chatbots reducing support tickets
- Lead scoring models boosting sales conversions
Long-term ROI Examples:
- AI-based forecasting systems
- Predictive analytics in supply chain management
Avoiding Cost-Related Pitfalls
Underestimating Hidden Costs
Failing to account for change management, data labeling, or cloud usage can derail your budget.
Solution: Build a contingency buffer of 10–20%.
Misjudging Scope
Starting with overly ambitious, complex AI use cases can inflate costs and delay benefits.
Solution: Begin with a pilot project or MVP (Minimum Viable Product).
Not Measuring Continuously
ROI isn’t static—ongoing measurement is key to understanding value over time.
Solution: Set KPIs and track quarterly or bi-annually.
Final Thoughts
AI integration is a significant but worthwhile investment. The key to success lies not just in developing high-performance models but in budgeting effectively, aligning AI with business goals, and rigorously tracking returns.
By understanding the cost structure—from data and development to deployment and maintenance—you can build a financially sound AI strategy. More importantly, a disciplined approach to ROI analysis ensures that AI doesn’t remain a buzzword, but becomes a measurable contributor to your organization’s success.