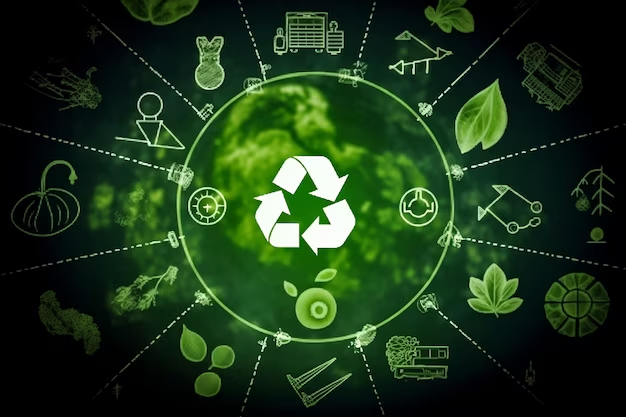
Generative AI is rapidly redefining the boundaries of creativity and automation. From producing realistic images, writing compelling stories, generating deepfake videos, and creating synthetic voices, generative AI tools have moved from labs to mainstream applications at an unprecedented pace. But with this exponential growth comes the need for careful reflection on how these technologies are developed and deployed.
Promoting the responsible development and use of generative AI is no longer a choice—it’s a necessity. This blog explores how developers, organizations, and society can collectively foster ethical practices, ensure transparency, and minimize the risks of misuse while still unlocking the transformative potential of these tools.
What Is Generative AI?
Generative AI refers to a class of machine learning models—like GANs (Generative Adversarial Networks), VAEs (Variational Autoencoders), and transformers—that can produce new content based on training data. These models learn patterns, styles, and structures to generate:
- Images (e.g., DALL·E, Midjourney)
- Videos (e.g., RunwayML, Pika)
- Music (e.g., Jukebox by OpenAI)
- Text (e.g., GPT, Claude)
- Code (e.g., GitHub Copilot)
- Voice (e.g., ElevenLabs, Resemble AI)
With such wide-ranging capabilities, generative AI poses both opportunities and ethical challenges that must be addressed proactively.
Why Responsible Development Matters
Unrestricted or poorly governed AI can result in:
- Misinformation and fake news
- Intellectual property violations
- Invasion of privacy
- Cultural and social bias
- Psychological manipulation
- Environmental impact due to high computational costs
The stakes are high. Failing to implement ethical guardrails today could lead to irreversible societal harm tomorrow.
Key Principles for Responsible Generative AI
1. Transparency
AI developers should clearly communicate:
- How their models are trained (data sources, biases).
- What capabilities and limitations the models have.
- When and where AI-generated content is used, especially in sensitive contexts (e.g., journalism, healthcare).
Transparency builds trust and allows for informed decision-making by users and regulators.
2. Accountability
Entities building or using generative AI must take responsibility for:
- Outputs produced by their systems
- Harms caused by the misuse of their models
- Providing redress mechanisms for affected individuals
This means putting systems in place for auditing, reporting, and responding to ethical concerns.
3. Fairness and Inclusivity
Bias in training data often leads to biased outputs. Developers should:
- Audit models for racial, gender, and cultural bias.
- Include diverse datasets that reflect global realities.
- Test outputs across demographics and use cases.
Inclusive AI reduces the risk of reinforcing stereotypes or excluding marginalized groups.
4. User Consent and Control
Responsible AI use should respect user autonomy:
- People should know when they’re interacting with AI, not humans.
- Individuals must consent to having their data used for training AI.
- Tools should allow users to opt-out, modify or delete content involving their likeness or intellectual property.
5. Safety and Risk Mitigation
Model developers should conduct thorough evaluations for misuse scenarios, including:
- Impersonation (deepfakes)
- Fraud (synthetic identity)
- Harassment or hate speech
- Intellectual property theft
Developers can also:
- Restrict certain prompt types
- Implement content filters or watermarking
- Use API-based access instead of open-source models to control deployment
6. Environmental Sustainability
Training large generative models consumes vast computing resources, contributing to carbon emissions. Responsible AI teams should:
- Report energy use and emissions
- Optimize model efficiency
- Use green energy sources where possible
- Consider model reuse and fine-tuning over training from scratch
Responsible Use by Businesses and Users
For Organizations:
- Establish internal AI ethics guidelines
- Appoint an AI ethics board or advisory group
- Provide training and education for staff on ethical AI usage
- Collaborate with external stakeholders (academia, policy makers)
For Creators and End Users:
- Avoid deceptive use of AI content (e.g., posing AI art as photography)
- Give credit to tools and models used in creation
- Be transparent with clients or audiences about the role of AI in the creative process
- Respect intellectual property and avoid generating close copies of copyrighted works
Responsible Model Deployment Lifecycle
Design Phase
- Define ethical goals and intended use.
- Consider misuse cases from the beginning.
Data Collection
- Use high-quality, diverse, and consented data.
- Avoid scraping copyrighted or sensitive materials without permission.
Training and Testing
- Monitor for harmful biases.
- Conduct adversarial testing to find abuse vulnerabilities.
Deployment
- Roll out gradually.
- Use API rate limits and approval workflows for risky use cases.
Monitoring and Feedback
- Track outputs and flag misuse.
- Allow users to report problems or provide feedback.
- Continuously update based on new threats and societal shifts.
The Role of Governments and Regulators
Governments have a crucial role to play in establishing guardrails for generative AI. Effective regulation should:
- Protect individuals from deepfake misuse, impersonation, and content fraud
- Mandate labeling of AI-generated content in certain sectors
- Ensure compliance with data privacy laws
- Penalize malicious or unethical use of AI tools
- Encourage international cooperation to avoid regulatory loopholes
Notable efforts include:
- EU’s AI Act: A tiered framework that imposes strict rules on high-risk AI systems
- China’s deepfake law: Requires synthetic media to carry clear disclosures
- US legislative proposals: Target deepfakes in election interference and sexual content
The Way Forward
Generative AI is not inherently good or bad—it is a tool, one of the most powerful ever created. The responsibility lies with us to guide its evolution with intention, empathy, and foresight.
Responsibility doesn’t stifle innovation—it ensures that innovation lasts.
By embedding ethical principles into development pipelines, empowering users with knowledge and control, and building strong regulatory frameworks, we can ensure that generative AI contributes meaningfully to society, rather than destabilizing it.
Cultivating a Responsible AI Mindset
Technological progress should be driven by human values. Promoting responsible AI requires a cultural shift as much as a technical one.
Encourage:
- Open dialogue between creators, ethicists, and users.
- Interdisciplinary teams—not just engineers, but artists, historians, psychologists, and policy experts.
- Human-centered design that values dignity, consent, and empowerment.
As we stand at the crossroads of creativity and computation, let us choose a path where generative AI enhances, not endangers, the human experience. The future of this technology doesn’t just depend on breakthroughs in machine learning—it depends on us.
Together, we must build, use, and govern generative AI responsibly.