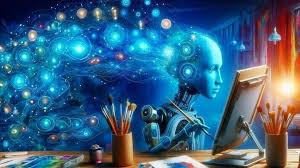
Generative AI has already made remarkable strides—from creating photorealistic images to composing original music and generating human-like conversations. Yet, these achievements may only represent the beginning of a much broader transformation. As researchers dive deeper into uncharted territories of AI development, the next generation of breakthroughs is likely to redefine creativity, problem-solving, and even consciousness as we understand it.
This blog explores the potential frontiers of generative AI research, outlining where innovations are likely to occur and what they could mean for society, science, and the future of intelligence.
Toward Truly Generalized Multimodal Models
One of the most anticipated advances in generative AI lies in the development of generalized multimodal models—systems that seamlessly understand and generate content across multiple data types like text, images, audio, and video.
While today’s multimodal models can generate an image from a prompt or describe a video using natural language, they still treat these tasks as loosely connected. The next wave of research is moving toward integrated sensory models capable of synthesizing inputs and outputs across domains with near-human fluidity.
Imagine an AI that can watch a live sports game, comment on it like a broadcaster, draw a highlight sketch, and generate a background soundtrack—all simultaneously. Achieving this will require not just larger datasets, but novel architectures that mirror the way human perception naturally blends sight, sound, language, and motion.
Zero-Shot Reasoning and Compositional Intelligence
Although generative AI is becoming increasingly skilled at replicating existing styles or patterns, its capacity for abstract reasoning remains limited. Upcoming breakthroughs will focus on enabling zero-shot generalization—where a model can solve completely new problems without specific training examples.
This involves compositional intelligence, or the ability to break complex problems into smaller parts and recombine known elements to reach novel conclusions. For example, a model that has learned to build chairs and tables might extrapolate how to design a stool without being explicitly trained on it.
To make this possible, researchers are exploring mechanisms like neuro-symbolic reasoning, hierarchical memory networks, and dynamic context windows that allow models to perform multi-step inference and logical deduction.
Energy-Efficient and Environmentally Responsible Training
Generative AI models are increasingly criticized for their high energy consumption and carbon footprint. Training large language models, for instance, can emit as much carbon as multiple transatlantic flights. The next breakthrough is likely to be not just in capability but in sustainability.
Research is advancing in low-rank adaptation, sparse training architectures, quantization, and model distillation—techniques that allow models to learn efficiently using fewer parameters and less computational power. We can expect to see AI models that are 10 to 100 times smaller, yet nearly as capable as today’s giants.
In tandem, efforts are underway to develop self-supervised training methods that minimize the need for curated datasets, further reducing the environmental and labor costs of development.
AI That Understands Time and Causality
Current generative models are largely pattern-based—they predict what comes next based on what they’ve seen, but lack a deep understanding of cause and effect. To push the boundaries of AI’s problem-solving capacity, researchers are working on models that understand temporal logic and causality.
This involves training models not just on static snapshots, but on longitudinal data streams—such as video timelines, sensor recordings, or behavioral sequences. These models will be able to infer the “why” behind the “what,” enabling breakthroughs in domains like predictive healthcare, financial modeling, and autonomous systems.
For example, a causally aware AI in healthcare could predict disease onset not just from symptoms but from subtle lifestyle patterns or genetic predispositions—something pattern-based models would miss.
Self-Evolving and Self-Healing Models
As generative AI is deployed in more critical environments, robustness becomes a key priority. One promising area of research is the creation of self-evolving or self-healing models—AI systems that can diagnose their own failures and retrain themselves on new data in real time.
These systems will incorporate feedback loops that continuously fine-tune their weights, optimize outputs based on live interactions, and prevent drift in performance over time. A future generative model embedded in a factory could not only detect its own predictive errors in equipment maintenance but also recalibrate its parameters without human intervention.
Such dynamic learning could lead to AI systems that behave more like biological organisms—learning, adapting, and evolving in response to environmental shifts.
AI with Emotional and Intentional Awareness
While current language models can mimic empathy or emotion in conversation, they do so without any real internal states. Breakthrough research is focused on affective computing—infusing generative AI with emotional understanding and intent modeling.
This involves training models to recognize emotional cues in text, speech, and imagery, and then tailor their responses to suit the emotional context. More advanced forms may involve theory-of-mind modeling, where AI begins to simulate the thoughts, beliefs, and intentions of the user.
In customer service, therapy, education, and social robotics, emotionally attuned AI will revolutionize engagement, making machines not just responsive, but seemingly relatable.
Quantum Generative AI
As quantum computing advances, it opens the door for a completely new generation of quantum-powered generative models. These would be capable of performing massive parallelism and solving probabilistic problems far beyond the reach of classical computing.
Quantum AI could simulate chemical reactions, biological systems, or financial markets with staggering precision, leading to breakthroughs in fields like materials science, drug discovery, and macroeconomic modeling.
We are just beginning to explore how quantum circuits can be used to generate and manipulate complex patterns. The first quantum GANs (Generative Adversarial Networks) and quantum diffusion models are already being tested in research labs. Over the next decade, these could completely redefine what generative AI is capable of.
Ethical Self-Governance in Generative Systems
As AI becomes more autonomous, there’s a growing need for embedded ethical frameworks within generative models. Future AI systems will be expected to not just follow external filters or guidelines, but to self-regulate based on moral reasoning.
This area of research involves encoding ethical principles—like fairness, transparency, and accountability—directly into model training and architecture. Reinforcement learning from human feedback (RLHF) is a current step in this direction, but the goal is to go further: to enable AI that can weigh competing values and make morally informed decisions.
In the future, we might see AI that can explain not just what it generated, but why it made certain decisions, complete with moral justifications and context awareness.
Generative AI and Lifelong Learning
Today’s AI models are static once trained. But the human brain learns continuously across a lifetime. The next breakthrough will focus on lifelong learning or continual learning in generative AI.
This would enable models to retain old knowledge while integrating new experiences, without succumbing to catastrophic forgetting. Such a capability would be transformative in fields like education, robotics, and dynamic content generation.
For instance, an AI tutor could continuously adapt its teaching style based on how a student learns over months or years, getting more effective with each interaction.
Open-Source Innovation and Collective Intelligence
An often-overlooked driver of breakthroughs in generative AI is the growing open-source movement. Platforms like Hugging Face, Stability AI, and OpenBioML are democratizing access to powerful models and datasets, enabling rapid experimentation and collaboration.
Future breakthroughs may not come from large corporations alone, but from decentralized research communities. These communities can train models on distributed infrastructure, share insights freely, and iterate more quickly than centralized labs.
As open-source generative AI becomes more advanced, it may evolve into a form of collective intelligence, where models trained in one context can rapidly adapt to others through modular learning and plug-and-play APIs.
Conclusion
The future of generative AI holds extraordinary promise—and an equal measure of complexity. The next wave of breakthroughs will go far beyond creating text or images. It will reshape how we interact with machines, how we understand intelligence, and how we solve the world’s most pressing challenges.
From emotionally aware companions to quantum-accelerated researchers, the upcoming generation of generative AI will be more than a tool—it will be a partner in discovery, innovation, and creativity.
By investing in responsible, inclusive, and interdisciplinary research today, we can ensure that tomorrow’s AI breakthroughs uplift humanity rather than undermine it. The journey ahead is not just about smarter machines—it’s about creating smarter futures.