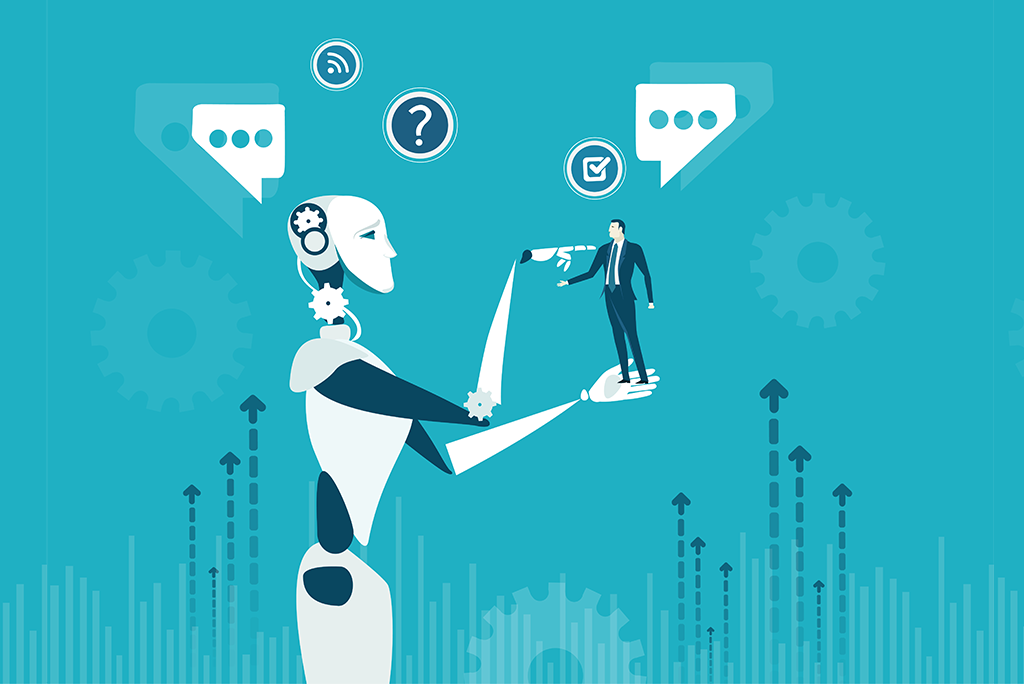
Artificial Intelligence (AI) has become a powerful force behind data-driven decision-making in organizations. From recommending candidates in recruitment processes to allocating medical resources, AI systems are being used to streamline workflows, reduce costs, and improve accuracy. However, as the influence of AI on decision-making increases, so do the ethical challenges associated with its deployment.
AI, by design, mimics human cognitive functions, yet it lacks consciousness, morality, and accountability. When left unchecked, it can perpetuate biases, infringe on privacy, and make decisions that raise serious ethical concerns. Managing these dilemmas is essential for businesses, governments, and society at large to ensure that AI contributes positively to human welfare.
This blog explores the key ethical dilemmas posed by AI in decision-making, their potential consequences, and practical strategies to manage them effectively.
Understanding Ethical Dilemmas in AI
An ethical dilemma arises when a decision leads to a conflict between competing values or principles. In the context of AI, such dilemmas typically involve issues such as:
- Fairness vs. efficiency
- Privacy vs. transparency
- Autonomy vs. control
- Accountability vs. delegation
For instance, an AI system may improve hiring efficiency but inadvertently discriminate against certain demographic groups. Or it may predict health outcomes accurately while processing highly sensitive personal data.
These dilemmas are particularly challenging because AI systems are often complex, opaque, and operate at scale, making the consequences far-reaching and sometimes difficult to trace.
Common Ethical Issues in AI Decision-Making
Let’s examine the most prevalent ethical dilemmas that arise when organizations use AI for decision-making.
1. Bias and Discrimination
AI systems learn from data, and if that data contains historical biases or reflects social inequalities, the system may replicate or even amplify these patterns. This can lead to discriminatory decisions in hiring, lending, insurance, or law enforcement.
Example: A resume screening tool trained on historical hiring data may favor male candidates over equally qualified female applicants if past data was skewed.
2. Lack of Transparency
Many AI models—especially deep learning algorithms—are considered “black boxes” because their decision-making logic is not easily interpretable. This lack of explainability raises concerns about trust and fairness.
Example: If an employee is denied a promotion based on an AI recommendation, how can they understand or contest the decision?
3. Invasion of Privacy
AI systems often rely on massive amounts of personal data to function effectively. However, collecting and using this data can violate privacy rights, especially if individuals are unaware their information is being used.
Example: AI-powered employee monitoring tools might track keyboard activity, facial expressions, or communications without clear consent.
4. Erosion of Human Autonomy
When organizations overly rely on AI systems, it can diminish human judgment and autonomy. This is particularly dangerous in high-stakes contexts like criminal sentencing or medical diagnosis.
Example: A judge might rely too heavily on a risk assessment tool to determine bail eligibility without questioning its output.
5. Accountability and Liability
Who is responsible when an AI system makes a harmful or unethical decision? The developer, the user, or the organization? Assigning accountability in AI-driven decisions is a complex and unresolved issue.
Example: If a self-driving car causes an accident due to a software glitch, is the manufacturer liable?
The Importance of Ethical AI Governance
As organizations integrate AI into decision-making processes, they must adopt strong governance frameworks that prioritize ethics, transparency, and accountability. Ethical AI is not just a compliance checkbox—it is fundamental to building trust with employees, customers, and society.
Governance must ensure that AI aligns with human values, legal requirements, and social norms. It should involve cross-functional collaboration between technologists, ethicists, legal teams, and domain experts.
Strategies for Managing AI Ethical Dilemmas
Organizations can take proactive steps to manage ethical dilemmas related to AI in decision-making. Below are key strategies to consider:
1. Adopt Ethical AI Principles
Establish a clear set of principles that guide AI development and use within the organization. These principles should address fairness, transparency, privacy, accountability, and safety.
Examples of widely accepted principles:
- Do no harm
- Ensure fairness and non-discrimination
- Be transparent and explainable
- Respect user privacy
- Maintain human oversight
Codifying these principles provides a foundation for ethical decision-making at every stage of AI development.
2. Use Explainable AI (XAI)
Prioritize models that offer transparency in how decisions are made. Explainable AI tools help users understand and trust the system, especially in sensitive applications.
Techniques include:
- LIME (Local Interpretable Model-Agnostic Explanations)
- SHAP (SHapley Additive exPlanations)
- Decision trees or rule-based systems
These methods provide insights into why a model produced a particular outcome, allowing stakeholders to evaluate its fairness and relevance.
3. Conduct Regular Ethics Audits
AI systems should be regularly audited for bias, privacy risks, and unintended consequences. Ethics audits can reveal hidden problems before they escalate into major issues.
Audit components may include:
- Data source analysis
- Fairness testing across demographics
- Model performance reviews
- Stakeholder impact assessments
These audits should be documented and include input from independent reviewers when possible.
4. Implement Bias Mitigation Techniques
Use statistical and algorithmic methods to detect and reduce bias in training data and model outcomes.
Common techniques include:
- Data re-sampling to balance class distributions
- Fairness constraints during model training
- Adversarial debiasing methods
Additionally, it’s essential to include diverse perspectives during the development process to identify and address biases early.
5. Ensure Informed Consent and Data Privacy
Clearly inform individuals when their data is being collected or used in AI systems, especially for decision-making purposes.
Best practices:
- Use opt-in mechanisms for data collection
- Provide clear privacy notices
- Anonymize and encrypt sensitive data
- Adhere to data protection regulations (like GDPR or CCPA)
Respecting privacy builds trust and reduces legal risk.
6. Maintain Human Oversight
AI should support—not replace—human decision-makers. Especially in high-impact areas, humans should have the final say and the ability to override AI outputs when necessary.
Implementation tips:
- Design AI tools as decision support systems
- Train employees to critically assess AI outputs
- Use AI recommendations as one of multiple decision factors
This hybrid approach ensures accountability and enhances the quality of decisions.
7. Create an AI Ethics Committee
Establish a cross-functional team responsible for reviewing the ethical implications of AI projects. This committee can evaluate risk, approve sensitive use cases, and provide ongoing governance.
Committee members may include:
- Data scientists
- Ethicists
- Legal experts
- HR representatives
- External stakeholders (when applicable)
Their insights can guide the responsible use of AI within the organization.
8. Educate Employees and Developers
Building ethical AI requires awareness and knowledge. Organizations should offer training programs that teach developers, managers, and end-users about the ethical implications of AI.
Topics to include:
- AI bias and fairness
- Data ethics
- Responsible AI design
- Legal and regulatory standards
A well-informed workforce is essential to maintaining ethical AI practices.
The Role of Policy and Regulation
While organizations play a key role, governments and regulators are also stepping in to ensure AI is used ethically. Legislation such as the EU AI Act, GDPR, and emerging frameworks from countries like the U.S., Canada, and India aim to set boundaries and promote transparency in AI use.
Organizations must stay informed and compliant with these evolving regulations to avoid legal consequences and stay competitive.
Conclusion
As AI continues to reshape decision-making across industries, the ethical challenges it brings must not be overlooked. Bias, privacy violations, and accountability issues are not just theoretical concerns—they have real-world implications for individuals, organizations, and society.
Managing these ethical dilemmas requires a balanced and proactive approach. By adopting principles of fairness, transparency, and accountability, and embedding them into AI design and deployment, organizations can unlock the benefits of AI while minimizing harm.
The future of AI will not be determined by how smart our algorithms become, but by how responsibly we use them. Ethical AI is not just about avoiding harm—it’s about building systems that align with our values, serve humanity, and create a more equitable and trustworthy digital world.