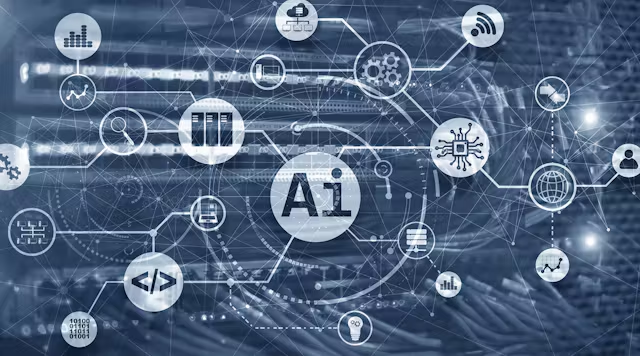
Artificial Intelligence (AI) is rapidly reshaping industries by improving decision-making, automating routine tasks, and uncovering actionable insights from data. However, integrating AI into your existing workflows isn’t just about installing new tools—it requires strategic planning, collaboration, and a shift in how your business operates.
This blog will walk you through the key steps to successfully integrate AI into your workflow, ensuring a smooth transition and maximum return on investment.
1. Identify Clear Business Objectives
Before diving into AI, it’s essential to define what you want to achieve. Whether your goal is to increase productivity, reduce operational costs, enhance customer experience, or uncover new market opportunities, having a clear vision will help you choose the right AI solutions.
Questions to ask:
- What problem are we trying to solve?
- Which metrics define success?
- Is the goal efficiency, accuracy, speed, or insight?
Example: A logistics company might aim to reduce delivery times by 15% using route optimization powered by AI.
2. Audit Your Existing Workflow and Data
AI thrives on clean, high-quality data and well-defined processes. Analyze your current workflow to identify inefficiencies, data silos, and manual tasks that could benefit from automation or intelligent decision-making.
Checklist:
- Map out end-to-end processes.
- Identify bottlenecks or repetitive tasks.
- Evaluate the quantity, quality, and accessibility of your data.
- Check for structured and unstructured data sources.
Example: In HR, repetitive tasks like resume screening or scheduling interviews can be streamlined with AI tools, assuming you have enough historical hiring data.
3. Choose the Right AI Use Case
Instead of trying to transform your entire operation overnight, start with a focused, high-impact use case. Look for areas that are:
- Data-rich
- Repetitive
- Costly or time-consuming
- Closely aligned with your business goals
Examples:
- Chatbots for customer support
- Predictive analytics for sales forecasting
- Document automation in legal teams
- Personalized marketing recommendations
4. Select the Appropriate AI Tools and Technology
Depending on your use case and technical capabilities, you can choose between:
- Off-the-shelf AI tools (e.g., Salesforce Einstein, Grammarly, ChatGPT)
- Custom-built AI models developed in-house or via AI consultants
- APIs and SaaS platforms that integrate AI into existing systems
Factors to consider:
- Integration capabilities with your current systems
- Scalability and flexibility
- Vendor reputation and support
- Security and compliance requirements
5. Ensure Data Readiness
AI is only as good as the data it learns from. Invest in data cleaning, labeling, and structuring before deploying any AI model. You may also need to set up data pipelines and cloud infrastructure to support real-time analytics and automation.
Tips:
- Standardize your data formats
- Remove duplicates and errors
- Implement data governance policies
- Use tools like ETL (Extract, Transform, Load) pipelines
6. Build a Cross-Functional Team
Successful AI integration requires collaboration between business, technical, and operational teams. Your implementation team should ideally include:
- AI/ML engineers or data scientists
- Software developers
- Domain experts (e.g., sales, HR, supply chain)
- Change management leaders
Bonus Tip: Having a dedicated AI project manager helps keep the initiative aligned with business goals and timelines.
7. Pilot the AI Solution and Validate Results
Before full deployment, run a pilot program. Test the AI in a controlled environment, evaluate performance, and gather feedback from real users.
Measure key outcomes such as:
- Time saved
- Accuracy improvements
- User adoption rates
- ROI and cost-effectiveness
Example: A finance team might pilot an AI tool for automating expense approvals, measuring whether it reduces processing time by 50%.
8. Train Employees and Foster Adoption
AI adoption often fails due to resistance or lack of understanding. Invest in employee training and change management. Help teams see AI as a productivity enhancer, not a threat.
Ways to build adoption:
- Offer hands-on workshops
- Share pilot success stories internally
- Highlight how AI helps rather than replaces roles
- Include users in the feedback loop
9. Integrate AI into Daily Workflows
Once the pilot is successful, gradually scale the AI solution and integrate it into day-to-day operations. This could involve:
- Embedding AI in CRM, ERP, or custom dashboards
- Automating alerts, decisions, or approvals
- Updating standard operating procedures
Important: Ensure minimal disruption and provide technical support during rollout.
10. Monitor, Iterate, and Optimize
AI systems are not static—they learn and evolve. Continuously monitor performance, retrain models with new data, and collect user feedback.
What to track:
- Model accuracy and drift
- User satisfaction
- Cost and productivity metrics
- System uptime and response times
Schedule regular reviews to refine algorithms, improve predictions, and explore new use cases.
11. Address Ethical, Legal, and Security Concerns
Ensure your AI integration follows ethical guidelines, industry regulations, and data privacy laws like GDPR or HIPAA.
Checklist:
- Protect customer and employee data
- Eliminate bias in training data and algorithms
- Provide transparency in AI decisions
- Obtain necessary consent for data use
Building trust in AI systems is just as important as functionality.
12. Scale Across Departments and Functions
Once AI proves its value in one area, expand into others. Build a roadmap for enterprise-wide AI transformation by:
- Sharing success metrics and lessons learned
- Reusing components (data pipelines, APIs, etc.)
- Encouraging departments to propose new use cases
- Aligning future AI projects with strategic business goals
Conclusion: AI Integration is a Journey, Not a One-Time Event
Successfully integrating AI into your workflow is a strategic, multi-step process that requires clear goals, collaboration, data preparation, and continuous learning. When done right, it can transform your operations, drive innovation, and give you a competitive edge.
Whether you’re starting with automation or predictive analytics, the key is to think big, start small, and scale smart.