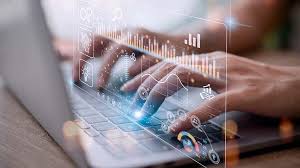
In today’s digital world, consumers are accustomed to personalized experiences across various platforms—whether it’s the recommendations they receive on Netflix, the targeted ads on Facebook, or the personalized shopping suggestions on Amazon. Behind these personalized interactions lies a powerful force: artificial intelligence (AI). By leveraging AI, businesses can tailor their services, products, and content to meet the unique preferences and behaviors of each consumer. But how exactly does AI collect and analyze the data necessary for such personalization? In this blog, we’ll explore the intricacies of how AI gathers data, processes it, and delivers customized experiences.
Understanding AI-Driven Personalization
At its core, AI-driven personalization aims to deliver the right content or product to the right individual at the right time. This involves using sophisticated algorithms and data models to tailor experiences based on a customer’s preferences, actions, and even predicted needs. However, this personalization requires data. Without data, AI would be unable to make informed decisions about what content or products are relevant to an individual.
The AI-powered personalization process involves two key stages: data collection and data analysis. Let’s dive into each of these stages and understand how they contribute to creating personalized experiences.
How AI Collects Data
AI relies on a variety of methods to collect data. The more data it has, the more accurate its predictions and recommendations can be. Here are the primary ways AI collects data for personalization:
1. User Interactions with Websites and Apps
When users visit websites or use mobile apps, they leave behind traces of their activities. These interactions can include:
- Pages viewed
- Time spent on each page
- Items clicked
- Searches performed
- Products added to cart or purchased
- Content shared or liked
All of this information is recorded and analyzed by AI systems to build a profile of the user’s preferences. For example, if a user spends a significant amount of time looking at running shoes, AI can infer that they have an interest in sports or fitness-related products. This insight allows businesses to recommend similar products or display targeted ads related to running gear.
2. Social Media Activity
Social media platforms like Facebook, Twitter, Instagram, and LinkedIn generate vast amounts of data that AI can leverage for personalization. Through user-generated content, AI can gather valuable information such as:
- Likes and shares
- Comments and reactions
- Posts and hashtags followed
- Connections and interactions with brands
For instance, AI can track the types of posts a user engages with most frequently and use that data to recommend more content in line with those interests. Similarly, businesses can use AI to monitor social media discussions about their brand to better understand consumer sentiment and create personalized marketing campaigns.
3. Customer Feedback and Reviews
Customer feedback, including ratings, reviews, and surveys, provides important insights into how users perceive a product or service. AI systems can analyze this unstructured data to detect patterns or sentiments that indicate customer satisfaction, preferences, or pain points.
For example, if customers frequently mention a specific feature of a product in their reviews (e.g., durability of a watch), AI can factor this information into its personalization algorithms to promote similar products or highlight that feature in future recommendations.
4. Transactional Data
For businesses, transactional data is one of the richest sources of insights. Every time a customer makes a purchase or interacts with a business’s system, valuable data is generated. This can include:
- Purchase history
- Frequency of purchases
- Payment methods
- Amount spent
- Customer loyalty (e.g., points or rewards)
By analyzing transactional data, AI can offer personalized discounts, upsell complementary products, or predict what the user is likely to purchase next. For instance, if a customer regularly buys a specific brand of coffee, AI might suggest related accessories like a coffee maker or special coffee blends.
5. Location Data
Geolocation is another critical data point that AI uses to personalize experiences. By tracking a user’s physical location through their mobile device or IP address, AI can offer:
- Location-based product recommendations (e.g., local restaurants, events, or services)
- Real-time discounts or promotions when the user is near a store
- Weather-based suggestions (e.g., recommending an umbrella when it’s raining)
For businesses with brick-and-mortar stores, location data is invaluable in sending personalized offers or alerts to users as they enter the vicinity.
6. Third-Party Data Integration
In addition to data collected directly from users, businesses often use third-party data sources to enhance their AI-driven personalization. This might include:
- Demographic data (age, gender, income, etc.)
- Behavioral data from other online platforms
- Data from industry reports or research
By integrating this third-party data, businesses can create a more comprehensive profile of their customers, leading to better-tailored experiences. For example, a fashion retailer might use third-party data on trends or consumer preferences to suggest the latest styles to users.
How AI Analyzes Data for Personalization
Once AI has collected enough data, the next step is analysis. This is where machine learning (ML) and data modeling come into play. The collected data is processed using various algorithms to derive insights and make predictions. Let’s explore some key methods AI uses to analyze this data:
1. Segmentation
One of the first steps in data analysis is segmentation. AI categorizes users into different groups based on similar characteristics or behaviors. These segments might include:
- Demographics (age, gender, location)
- Interests (fitness, gaming, fashion)
- Buying behaviors (high spenders, frequent shoppers)
By segmenting customers, businesses can tailor marketing strategies and product offerings to fit each group. For example, AI might target younger consumers with trendy fashion items, while promoting more classic styles to older consumers.
2. Pattern Recognition
AI uses pattern recognition to detect trends and correlations within the data. By studying past behaviors, AI can predict future actions. For instance, if a user frequently buys items related to fitness, AI may predict that they will likely purchase a gym membership or supplements in the near future.
This prediction capability enables businesses to recommend products or services that customers are likely to need before they even realize it. For example, e-commerce sites use pattern recognition to suggest products that complement a user’s previous purchases.
3. Natural Language Processing (NLP)
NLP enables AI to understand and interpret human language, whether written or spoken. This technology is used to analyze text-based data, such as customer reviews, support tickets, social media posts, and website chats.
By analyzing the sentiment and meaning behind text data, AI can better understand user preferences, needs, and feelings. This helps businesses refine their personalization efforts, ensuring that they speak to customers in the most relevant way.
For example, if a customer expresses frustration in a review, AI can recognize the negative sentiment and prioritize addressing their concerns. Conversely, positive reviews can be used to highlight aspects of a product that resonate with other users.
4. Predictive Analytics
AI uses predictive analytics to forecast future consumer behavior based on historical data. By analyzing patterns in a customer’s interactions, transactions, and preferences, AI can predict what the customer is most likely to do next.
For example, if a customer has repeatedly bought skincare products, AI may predict that they will likely purchase a new moisturizer when their current one runs out. This enables businesses to send timely and personalized product recommendations that increase the chances of a sale.
5. A/B Testing
A/B testing is a technique that involves testing two variations of a webpage, email, or advertisement to see which one performs better. AI automates this process, constantly testing different versions of personalized content, messaging, or offers. By analyzing the results, AI determines what works best for specific segments and fine-tunes future recommendations.
For example, a retailer might test different product recommendations on their homepage and use AI to determine which version leads to more clicks or conversions.
Conclusion
AI-driven personalization is transforming the way businesses interact with consumers. Through the collection and analysis of vast amounts of data, AI enables businesses to offer highly personalized experiences that resonate with individual customers. From transactional data and social media activity to predictive analytics and machine learning algorithms, AI has the power to make every customer interaction more relevant, engaging, and impactful.
As AI continues to evolve, its ability to collect and analyze data will only improve, allowing businesses to create even more tailored experiences. In the future, we can expect even deeper insights and more precise personalization that will continue to delight consumers and drive business success. The journey toward a fully personalized digital experience is only just beginning, and AI is leading the way.