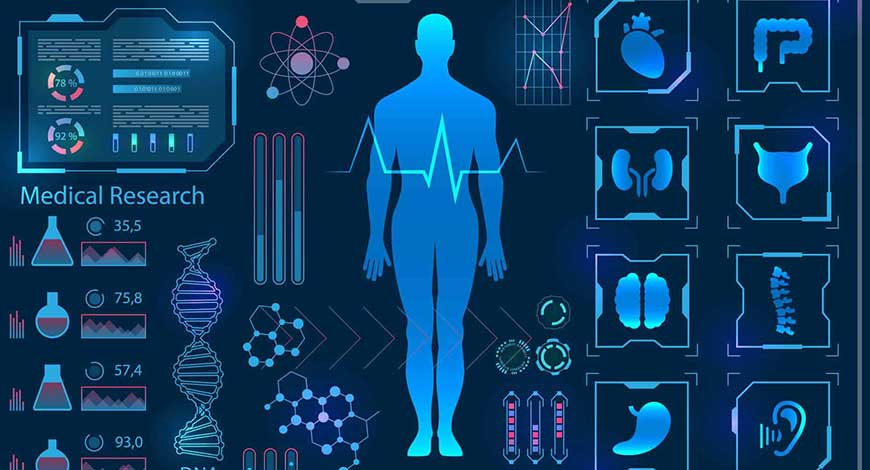
The healthcare industry stands at the forefront of technological transformation. Among the most groundbreaking innovations shaping this sector is Generative AI, a subset of artificial intelligence that doesn’t just analyze data—it creates entirely new outputs. In healthcare, this capability is being harnessed to accelerate drug discovery, improve diagnostics, and unlock novel possibilities in personalized medicine.
This blog explores how generative AI is revolutionizing two critical areas of healthcare—drug discovery and diagnostics—and why this matters for patients, researchers, and healthcare providers alike.
What is Generative AI in Healthcare?
Generative AI uses deep learning models like Generative Adversarial Networks (GANs), transformers, and variational autoencoders (VAEs) to generate new, data-like content—be it text, images, molecular structures, or medical diagnoses.
In healthcare, this means:
- Designing new drug molecules that could become future treatments
- Creating synthetic medical images to train diagnostic systems
- Simulating how diseases develop and how patients respond to treatments
Unlike traditional AI, which mostly classifies or predicts based on past data, generative AI creates data, offering unparalleled opportunities in areas where real-world data is limited or expensive to collect.
Generative AI in Drug Discovery
1. The Challenge of Drug Development
Drug discovery is a time-consuming and costly process:
- Average time: 10–15 years
- Average cost: $2.6 billion+
- Failure rate: Over 90%
Many potential treatments never make it past the lab because of poor efficacy, toxicity, or bioavailability. This is where generative AI steps in.
2. How Generative AI Transforms Drug Discovery
Generative AI accelerates the early-stage discovery pipeline by:
a) Generating Novel Molecules
AI models can learn chemical patterns and generate:
- Entirely new molecular structures
- Variants of known compounds with improved properties
These models use datasets of known drug-like molecules and can produce novel candidates in seconds—a process that previously took months or years.
b) Optimizing Drug Properties
Generative AI fine-tunes molecules for:
- Better binding to target proteins
- Lower toxicity
- Enhanced solubility or stability
c) Predicting Drug-Target Interactions
Models can simulate how well a drug will interact with a biological target (like a protein or receptor), minimizing costly trial-and-error experiments.
d) Accelerating Preclinical Screening
Generative models can simulate thousands of interactions in silico, vastly reducing the need for expensive wet-lab testing.
3. Real-World Examples of AI-Driven Drug Discovery
Insilico Medicine
- Developed a drug candidate for fibrosis in under 18 months using AI.
- Traditional methods would have taken over 3–4 years.
Atomwise
- Uses deep learning to predict binding affinities of molecules to target proteins.
- Helps pharmaceutical companies screen millions of compounds virtually.
BenevolentAI
- During the COVID-19 pandemic, their AI suggested baricitinib as a potential treatment, which later gained FDA approval.
4. Benefits of Generative AI in Drug Development
- Speed: Reduces discovery time from years to months
- Cost Efficiency: Limits expensive lab tests and failures
- Novelty: Finds molecular structures not yet discovered by humans
- Precision Medicine: Enables design of targeted therapies based on individual genetics
Generative AI in Diagnostics
While drug discovery is about finding treatments, diagnostics is about identifying diseases early and accurately. Generative AI is making significant strides here too.
1. Synthetic Medical Imaging for Training AI
A major challenge in training medical diagnostic systems is data scarcity. Privacy laws, ethical concerns, and limited datasets can restrict model development.
Generative AI helps by:
- Creating synthetic X-rays, MRIs, and CT scans that mimic real images
- Allowing AI models to train on larger, more diverse datasets
- Reducing bias by representing different ages, ethnicities, and conditions
For example, GANs can produce chest X-rays with realistic features of pneumonia, enabling better model training even in low-data environments.
2. Enhancing Image Quality and Resolution
Generative AI can:
- Upscale low-resolution scans
- Remove noise from blurry images
- Reconstruct damaged or partial data
This leads to clearer diagnostics, especially in early disease detection such as tumors or micro-fractures.
3. AI-Assisted Diagnosis
Generative AI isn’t just about data synthesis. It can assist in:
- Generating probable diagnoses from symptoms and test results
- Suggesting next steps or treatment options
- Personalizing risk scores based on genetic and lifestyle data
Models like OpenAI’s GPT-based tools and Google’s Med-PaLM are being trained to understand complex medical questions and provide clinical reasoning.
4. Multi-modal Diagnostics
Generative AI can combine multiple data sources—such as:
- Genomic data
- Imaging data
- Lab results
- Patient history
This holistic analysis helps identify patterns and conditions that may go unnoticed when analyzing each data type in isolation.
Ethical Considerations and Challenges
While the potential of generative AI is immense, several ethical and technical concerns must be addressed:
1. Data Bias and Representation
If training data lacks diversity (e.g., underrepresented populations), the AI may produce biased or ineffective results.
2. Explainability and Trust
Generative AI often operates as a “black box.” In medicine, doctors must understand why a diagnosis or molecule was generated.
3. Regulation and Approval
How do we verify the safety of AI-designed drugs or diagnoses? Regulatory bodies like the FDA are still developing frameworks to evaluate AI-generated health solutions.
4. Intellectual Property
Who owns a molecule designed by AI—the company, the developer, or the algorithm’s creator?
5. Privacy Concerns
Synthetic data generation must still comply with regulations like HIPAA and GDPR, especially when real patient data is used to train models.
The Future of Generative AI in Healthcare
The possibilities of generative AI in healthcare are only just beginning. Over the next decade, we may see:
- Digital twins: AI-generated simulations of patients to test treatments virtually
- On-demand drug creation: Tailored drugs generated for individuals based on genetic profiles
- Real-time diagnostics: Wearables and AI working together for continuous monitoring and early alerts
- AI-pharmacist bots: Generating and explaining treatment options to patients in understandable language
Generative AI is not just enhancing healthcare—it’s redefining it. From designing next-generation drugs in record time to enabling precision diagnostics that save lives, the impact of AI is undeniable. Still, its success depends on responsible use, interdisciplinary collaboration, and a commitment to equity and transparency.
As AI continues to learn and evolve, it holds the potential to democratize innovation, accelerate cures, and bring us closer to a future where healthcare is more predictive, personalized, and precise.