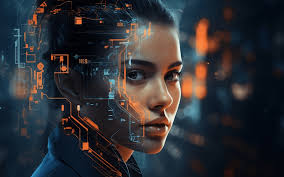
Artificial intelligence has witnessed transformative milestones over the past decade—from rule-based automation to neural networks, and from predictive analytics to generative models. But among these advancements, a new paradigm is emerging that may redefine our interaction with machines entirely: generative AI agents.
These aren’t just tools that respond to commands or generate text and images. Instead, generative AI agents are autonomous digital entities that understand goals, make decisions, and take action on behalf of users in dynamic environments. As the capabilities of AI models continue to evolve, so does their potential to act as independent, adaptable agents—effectively becoming digital collaborators rather than static assistants.
This blog delves into the concept of generative AI agents, their technological foundation, practical applications, potential challenges, and the transformative role they are likely to play across sectors.
What Are Generative AI Agents?
Generative AI agents are autonomous systems that combine the content creation abilities of generative models (like GPT, DALL·E, or MusicLM) with task-driven decision-making capabilities. These agents don’t simply generate text or media based on prompts—they interpret objectives, plan multi-step tasks, gather information, adapt to feedback, and execute actions to accomplish goals.
Imagine an AI that not only writes an email but also determines whom to contact, what time to send the message, and which attachments to include based on your calendar. Or a travel agent that finds your optimal itinerary, books your flights and accommodations, and reschedules meetings accordingly. These agents don’t wait for commands—they proactively deliver outcomes.
In essence, generative AI agents represent the fusion of generative intelligence with goal-oriented autonomy.
The Building Blocks of AI Agents
The rise of generative AI agents is made possible by advances across several areas of machine learning and software engineering. These components function like the organs and nervous system of a digital organism:
1. Language Models as Cognitive Engines
Large language models (LLMs) such as GPT-4, Claude, and LLaMA serve as the “brain” of many AI agents. These models interpret natural language instructions, summarize information, translate across contexts, and generate new content. When fine-tuned or prompted effectively, they enable agents to think through problems, generate options, and justify decisions.
2. Tools and Plugins
Agents become more useful when they can interact with external tools. This includes APIs, web browsers, databases, spreadsheets, messaging platforms, calendars, and more. Tool use enables agents to bridge the gap between text generation and real-world action.
3. Memory Systems
Unlike static bots, generative agents can recall previous conversations, preferences, goals, or mistakes. Persistent memory enables contextual continuity, making the agent feel more intelligent and human-aware over time.
4. Planning and Decision-Making Modules
Agents use planning frameworks to break down high-level goals into actionable steps. Some systems rely on techniques like tree-of-thought prompting, chain-of-reasoning, or behavior trees to determine the best course of action.
5. Feedback Loops
Agents learn by doing. Through feedback from users or the environment, they refine future decisions. Reinforcement learning and human-in-the-loop adjustments enhance their adaptability and accuracy.
Real-World Use Cases of Generative AI Agents
Generative agents are already finding real-world applications, albeit in their early stages. Here are some domains where these intelligent assistants are making an impact:
Personal Productivity
Calendar bots, email agents, and virtual secretaries are evolving from simple reminders to proactive planners. An agent might reschedule your appointments based on travel time, prepare daily briefings, or prioritize your inbox based on tone, urgency, and sender relationship.
Software Development
AI development agents can write code, debug issues, test modules, and even generate documentation. Tools like GitHub Copilot, Cody, or CodeWhisperer are precursors to fully autonomous dev assistants that operate across the software lifecycle.
Customer Support
Customer service agents are moving beyond chatbots. They now read support tickets, analyze product documentation, offer tailored responses, and even initiate follow-ups. These AI workers can reduce resolution time while increasing personalization.
E-commerce
Imagine an AI agent that curates outfits for you, compares prices across platforms, tracks your shipping, and processes returns—all autonomously. Retail companies are investing in agents that redefine online shopping from passive browsing to intelligent assistance.
Business Automation
Internal operations like HR onboarding, IT troubleshooting, and procurement can be delegated to agents. These entities don’t just follow static scripts—they adapt workflows based on company policies and user interactions.
Creative Collaboration
Writers, designers, and musicians can work alongside agents that generate drafts, refine style, suggest alternatives, and collaborate on ideation. The agent becomes a co-creator, enhancing human creativity rather than replacing it.
The Role of Multi-Agent Systems
One of the most exciting developments in this frontier is the emergence of multi-agent ecosystems. Rather than relying on a single AI to do everything, multiple specialized agents can collaborate, divide tasks, and synchronize efforts toward a common objective.
For instance, an AI project team could consist of:
- A research agent that gathers background materials
- A planner agent that outlines the strategy
- A writer agent that drafts content
- An editor agent that polishes the work
- A coordinator agent that ensures deadlines and communication flow
Each of these agents would have specific goals and capabilities, much like human departments in an organization. Together, they form a digital workforce capable of executing complex tasks at speed and scale.
Benefits of Generative AI Agents
The potential upside of generative agents is enormous. Some of the key benefits include:
- Autonomy: Agents can operate independently, freeing humans from micromanagement.
- Scalability: Businesses can deploy thousands of agents for support, logistics, or development without proportional increases in staff.
- Consistency: Unlike human workers, agents maintain focus, tone, and accuracy consistently over time.
- 24/7 Availability: These systems don’t need breaks, sleep, or vacations. They provide continuous output and support.
- Customization: Agents can be trained to reflect individual user preferences, corporate values, or branding guidelines, delivering a unique experience to each customer.
Challenges and Concerns
Despite the promise, generative AI agents bring significant concerns that must be addressed before widespread adoption.
Security and Trust
Giving AI the autonomy to act raises serious security questions. What happens if an agent misinterprets instructions or accesses sensitive information? Guardrails, sandboxing, and strict permission controls are critical.
Misuse and Malicious Agents
Agents can be weaponized. Autonomous systems could spread misinformation, conduct fraud, or be exploited for unethical purposes. Identifying and preventing bad actors is an emerging area of research.
Data Privacy
Agents that operate across emails, calendars, or databases require access to personal and organizational data. Without robust privacy safeguards, these systems pose a risk of leakage or abuse.
Loss of Human Control
As agents become more autonomous, the risk of unintended consequences grows. We need transparency into how agents make decisions, and override capabilities must remain in human hands.
Dependence and Skill Degradation
Relying too heavily on AI agents could erode human decision-making skills or reduce our ability to function without them. A healthy balance between human agency and automation is essential.
Looking Ahead: The Future of AI Agents
The evolution of generative AI agents is still in its infancy, but the trajectory is unmistakable. In the coming years, we can expect:
- More Personalized Agents: Agents trained on individual data profiles will deliver experiences as unique as the users they serve.
- Increased Emotional Intelligence: By integrating sentiment analysis and empathy modeling, agents will better understand human emotions and adjust responses accordingly.
- Voice-Driven Agents: Voice will become the primary interface for interacting with agents, reducing friction and making access more intuitive.
- Interoperability Across Platforms: Users will deploy agents that travel with them across devices, services, and environments.
- Regulatory Frameworks: Governments and organizations will develop standards and regulations to ensure ethical use and safety of autonomous agents.
Final Thoughts
Generative AI agents represent a bold leap forward in artificial intelligence. They mark a transition from passive tools to intelligent collaborators—entities capable of achieving goals, adapting to contexts, and interacting dynamically with the world around them.
While challenges remain around ethics, control, and safety, the benefits of intelligent agents are poised to transform how we live, work, and create. As the frontier continues to expand, generative AI agents will become not just assistants, but partners—redefining the relationship between humans and machines in the digital age.