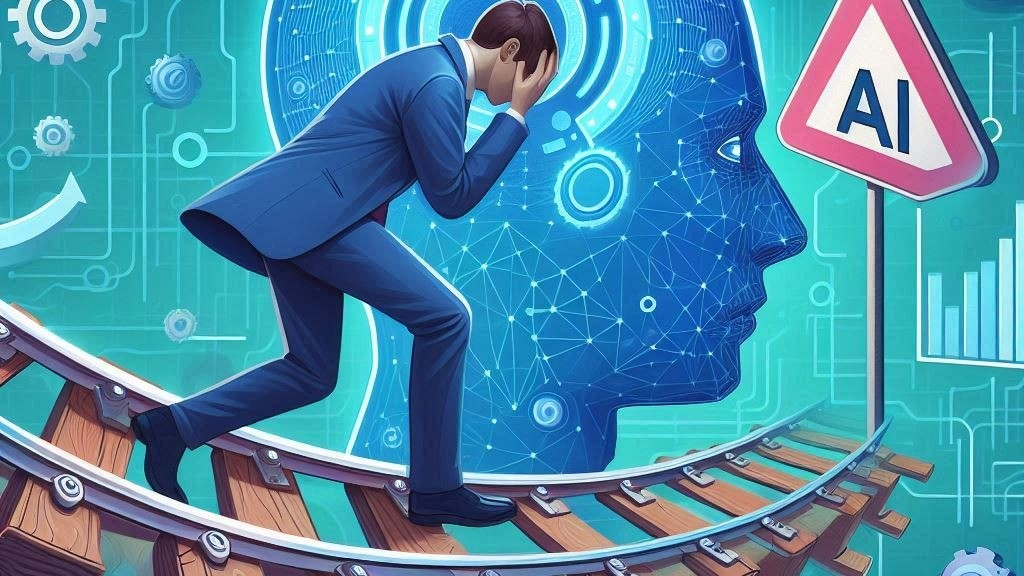
Artificial Intelligence (AI) is transforming industries—from customer service and logistics to finance and healthcare. Businesses increasingly see AI not as a futuristic concept, but as a practical tool for automation, decision-making, and personalization. However, integrating AI into the workplace is not as straightforward as adopting traditional software. It comes with unique complexities and potential risks that, if unaddressed, can lead to wasted investments, failed projects, or even reputational damage.
Whether you’re just beginning your AI journey or expanding existing initiatives, understanding the common pitfalls can help you avoid costly mistakes and increase the likelihood of success.
1. Lack of Clear Business Objectives
One of the most frequent mistakes is implementing AI without a clear understanding of why it’s needed. Organizations often jump into AI projects to keep up with trends or competitors without establishing specific, measurable business goals.
Why It’s a Problem:
- Leads to solutions in search of problems.
- Hard to define success or ROI.
- Results in poor stakeholder buy-in.
How to Avoid:
- Start with a clear problem statement.
- Define key performance indicators (KPIs) aligned with business goals.
- Ensure AI adds value beyond what traditional tools already provide.
2. Poor Data Quality and Availability
AI systems depend on data—the quality, quantity, and relevance of which directly affect outcomes. Organizations often underestimate how fragmented, incomplete, or biased their data is until they begin training models.
Why It’s a Problem:
- Leads to inaccurate or biased predictions.
- Requires excessive pre-processing and data cleaning.
- Can delay deployment or degrade model performance over time.
How to Avoid:
- Audit and clean data before launching AI projects.
- Ensure consistent data formats and centralized repositories.
- Establish robust data governance frameworks.
3. Overreliance on Out-of-the-Box Models
Many companies use pre-trained AI models or vendor platforms assuming they will work perfectly for their unique context. However, off-the-shelf models are often trained on generalized data and may not reflect your domain-specific needs.
Why It’s a Problem:
- Results in irrelevant or suboptimal predictions.
- Makes it difficult to fine-tune or scale solutions.
- Creates false confidence in AI capabilities.
How to Avoid:
- Customize or fine-tune models using your own data.
- Involve data scientists or AI engineers in evaluation.
- Test models thoroughly before going live.
4. Ignoring Change Management and User Adoption
AI doesn’t just impact technology—it changes workflows, decision-making, and job roles. Organizations often focus too heavily on building the system and neglect preparing employees for the transition.
Why It’s a Problem:
- Resistance from staff leads to poor adoption.
- AI tools are underutilized or misused.
- Cultural friction stalls innovation.
How to Avoid:
- Communicate clearly about the role and value of AI.
- Offer training and upskilling opportunities.
- Involve end users early in the development process.
5. Lack of Explainability and Transparency
Many AI systems—especially deep learning models—are seen as “black boxes,” producing outputs that are difficult to interpret. In sensitive domains like healthcare, finance, or hiring, a lack of transparency can raise ethical and legal concerns.
Why It’s a Problem:
- Erodes user trust in AI decisions.
- Raises compliance risks under laws like GDPR.
- Makes it hard to debug or improve models.
How to Avoid:
- Use interpretable models where possible.
- Implement explainability tools like LIME or SHAP.
- Document how data is used and how predictions are made.
6. Neglecting Security and Privacy Requirements
AI systems often process sensitive data. If security protocols are not tightly integrated, organizations can expose themselves to data breaches, IP theft, and regulatory penalties.
Why It’s a Problem:
- Increases vulnerability to cyberattacks.
- May violate data protection laws.
- Damages brand reputation.
How to Avoid:
- Encrypt data in transit and at rest.
- Anonymize personal information where possible.
- Conduct privacy impact assessments before deployment.
7. Underestimating Model Maintenance and Lifecycle Management
AI systems are not one-time deployments. They need ongoing tuning, re-training, and monitoring to remain effective as business conditions and data evolve.
Why It’s a Problem:
- Models degrade over time due to concept or data drift.
- Performance metrics decline without warning.
- Maintenance becomes resource-intensive if not planned.
How to Avoid:
- Build AI lifecycle management into your strategy.
- Automate performance monitoring and drift detection.
- Schedule periodic reviews and updates.
8. Overhyped Expectations
AI is powerful, but it’s not magic. Many teams enter AI projects expecting instant results, full automation, or dramatic ROI within weeks. This can lead to disappointment and project abandonment.
Why It’s a Problem:
- Causes loss of executive and team support.
- Skips crucial development and validation stages.
- Undermines credibility of future AI initiatives.
How to Avoid:
- Set realistic milestones and timelines.
- Communicate limitations and required iterations.
- Emphasize incremental value delivery.
9. Not Involving the Right Stakeholders
AI implementation requires collaboration across departments—including IT, data science, operations, legal, and HR. Leaving any of these stakeholders out of the loop can create misalignment or project delays.
Why It’s a Problem:
- Creates friction between business and technical teams.
- Leads to gaps in compliance or policy enforcement.
- Makes adoption and integration harder.
How to Avoid:
- Create cross-functional AI project teams.
- Hold regular stakeholder meetings and check-ins.
- Ensure business and technical goals are aligned.
10. Failure to Measure and Prove Impact
Even if your AI solution works as intended, it won’t be considered a success unless you can show measurable business impact. Failing to define or track ROI can prevent further investment in AI initiatives.
Why It’s a Problem:
- Projects are seen as cost centers, not value drivers.
- It’s harder to get buy-in for future AI work.
- You can’t identify which models or tools to scale.
How to Avoid:
- Define success metrics at the start (e.g., cost savings, time saved).
- Use A/B testing or pilot rollouts to quantify impact.
- Report results clearly to stakeholders.
11. Overlooking Ethics and Bias
Bias in AI models can lead to discriminatory outcomes, especially in hiring, lending, or law enforcement applications. Ethical missteps can lead to public backlash and legal issues.
Why It’s a Problem:
- Violates fairness principles and potentially the law.
- Causes reputational damage.
- Erodes user trust.
How to Avoid:
- Audit training data for representativeness and bias.
- Establish ethical AI guidelines and review boards.
- Implement fairness and accountability checks.
Conclusion
AI integration offers tremendous potential, but it’s not without risk. Many of the pitfalls organizations encounter are avoidable with the right planning, cross-functional collaboration, and governance.
Avoiding common mistakes such as poor data quality, lack of stakeholder involvement, ignoring compliance, or unrealistic expectations can drastically improve your chances of success. Instead of rushing to adopt the latest AI trends, take a strategic approach: define clear goals, prepare your organization, and treat AI as a journey, not a one-time event.
Ultimately, successful AI integration is not just about technology—it’s about aligning tools with people, processes, and values to create sustainable impact.