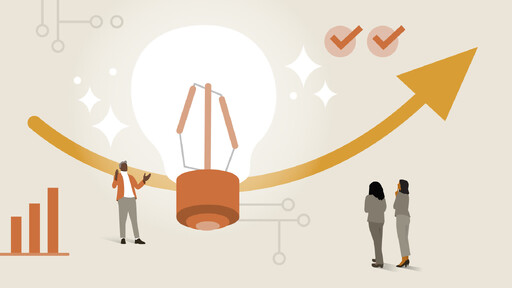
Artificial Intelligence (AI) is no longer a futuristic ideal—it’s a present-day force reshaping industries, operations, and customer experiences. However, for many businesses, especially those in the early or mid-stages of digital transformation, integrating AI into core functions can appear risky or uncertain. The key to overcoming hesitation and securing leadership buy-in is building a strong business case for AI integration.
A well-crafted business case answers critical questions: Why AI? Why now? What value will it deliver? What are the costs and risks? This blog serves as a practical guide for developing a compelling AI business case that aligns with strategic goals and delivers measurable outcomes.
Why Build a Business Case for AI?
Before diving into how, it’s important to understand the why. AI is a powerful tool, but like any enterprise technology, it requires thoughtful planning and resource commitment. A solid business case helps:
- Clarify strategic objectives
- Quantify expected benefits and ROI
- Identify costs, risks, and mitigation strategies
- Gain executive sponsorship and stakeholder support
- Establish a roadmap for implementation
Without a structured justification, AI initiatives risk being dismissed as experimental or non-essential.
Step 1: Identify the Business Problem or Opportunity
The foundation of any business case begins with a clear problem or opportunity that AI can address.
Ask:
- What operational inefficiencies are costing us time or money?
- Are there customer experience gaps we can close?
- Do we have processes that could benefit from automation?
- Are there strategic goals AI can accelerate?
Example: A retail company may face high cart abandonment rates. By implementing an AI-driven recommendation engine or chatbot support, they could reduce abandonment and increase sales.
Focus on use cases with tangible pain points or value potential. These serve as compelling entry points for AI experimentation.
Step 2: Align AI with Strategic Goals
For AI integration to gain traction, it must align with the organization’s broader objectives.
Strategic alignments might include:
- Enhancing customer satisfaction
- Reducing operational costs
- Increasing employee productivity
- Accelerating product development
- Improving data-driven decision-making
Ensure that your proposed AI initiative directly contributes to one or more of these high-priority goals. For instance, if a company’s objective is to improve customer response times, an AI chatbot integrated into the service desk aligns perfectly.
Step 3: Define the Scope of the AI Project
Clearly define what the AI solution will do, what it won’t do, and where it fits within the existing business structure.
Include:
- Functional boundaries (e.g., only automating invoice processing)
- Stakeholders involved
- Systems and processes affected
- Data sources required
By setting parameters, you avoid scope creep and make it easier to measure success. A narrowly scoped pilot project is often more effective than a broad, ambiguous initiative.
Step 4: Quantify the Benefits
Quantifiable outcomes help transform AI from an abstract concept into a concrete value proposition.
Types of benefits to calculate:
- Cost Savings – Reduced labor, fewer errors, lower overhead.
- Revenue Increases – Enhanced upselling, personalized marketing, better customer retention.
- Productivity Gains – Faster processing times, improved decision-making.
- Risk Reduction – Better fraud detection, improved compliance.
Example: Automating invoice data entry could save the finance team 10 hours per week, translating into thousands in annual cost savings.
Also consider qualitative benefits such as improved employee satisfaction or better customer engagement, even if they’re harder to assign a dollar value to.
Step 5: Assess the Costs
Your business case should account for both initial and ongoing costs, even if you’re using free or open-source AI tools.
Common cost elements:
- Technology infrastructure (cloud, APIs, software)
- Data preparation and cleaning
- Model development and training
- Integration with current systems
- Staff training and change management
- Maintenance and support
If you’re planning to use external vendors or consultants, include those fees as well. A clear understanding of costs prevents unrealistic expectations and budget overruns.
Step 6: Conduct a Risk and Feasibility Analysis
No business case is complete without addressing potential risks and how you plan to manage them.
Common AI risks:
- Data privacy and security concerns
- Bias in training data leading to unfair or inaccurate outcomes
- Resistance to change from staff
- Integration challenges with legacy systems
- Unclear accountability for model decisions
Mitigation strategies:
- Use anonymized or synthetic data for model training.
- Create cross-functional teams with IT, legal, and operations.
- Start with explainable AI tools that build trust.
- Provide ongoing training and involve end-users early.
Including a thoughtful risk analysis demonstrates maturity and foresight, building confidence among stakeholders.
Step 7: Estimate ROI and Payback Period
Senior decision-makers want to know: When will we see a return?
Use basic ROI calculations:
- ROI (%) = (Net Benefit / Cost of Investment) × 100
- Payback Period = Cost of Investment / Annual Benefit
Even if exact numbers are hard to predict, ranges or estimates can be effective.
Example: If an AI-driven inventory optimization tool costs $25,000 and is expected to reduce stockouts and overstocking by $15,000 per year, the payback period is less than two years.
Step 8: Define Success Metrics
Clear metrics help measure effectiveness post-deployment. They also provide accountability and guide future optimization.
Success metrics might include:
- Reduction in processing time or labor hours
- Increase in customer satisfaction (e.g., Net Promoter Score)
- Decrease in errors or rework
- Revenue or cost changes tied to AI adoption
Make sure metrics are:
- Specific
- Measurable
- Relevant to the business goal
- Time-bound
Step 9: Build the Implementation Plan
Outline how the AI solution will be deployed and integrated into operations. This instills confidence that the project is manageable and thought through.
Key components:
- Timeline (e.g., proof of concept, pilot, full rollout)
- Milestones and checkpoints
- Assigned roles and responsibilities
- Training and support plans
- Change management activities
A phased approach—starting with a small pilot—often works best to prove value and gather learnings before scaling.
Step 10: Present the Business Case
Once the case is built, it’s time to present it to decision-makers. Tailor your presentation to your audience:
- Executives want to see ROI, strategic value, and risk mitigation.
- IT Leaders focus on technical feasibility, security, and scalability.
- Business Managers care about process efficiency and usability.
- Finance Teams need clear budgeting and value justification.
Use visuals, summaries, and real-world examples where possible. Be prepared to answer questions about alternative options, failure contingencies, and long-term support.
Common Mistakes to Avoid
- Overpromising outcomes: Stay realistic about what AI can and cannot do.
- Ignoring stakeholders: Involve end-users and department heads early.
- Focusing only on technology: Business impact should lead the discussion.
- Skipping pilot validation: Always test before scaling.
- Underestimating change management: People matter as much as tech.
Conclusion
Building a compelling business case for AI integration is not just about showcasing technology—it’s about demonstrating strategic alignment, financial viability, and practical feasibility.
By clearly defining the problem, aligning with business goals, quantifying costs and benefits, mitigating risks, and outlining a realistic implementation plan, you position your AI initiative for approval and success.
AI adoption is not a one-size-fits-all journey. A well-developed business case is your roadmap, helping guide your organization toward smarter, more agile, and data-driven operations.