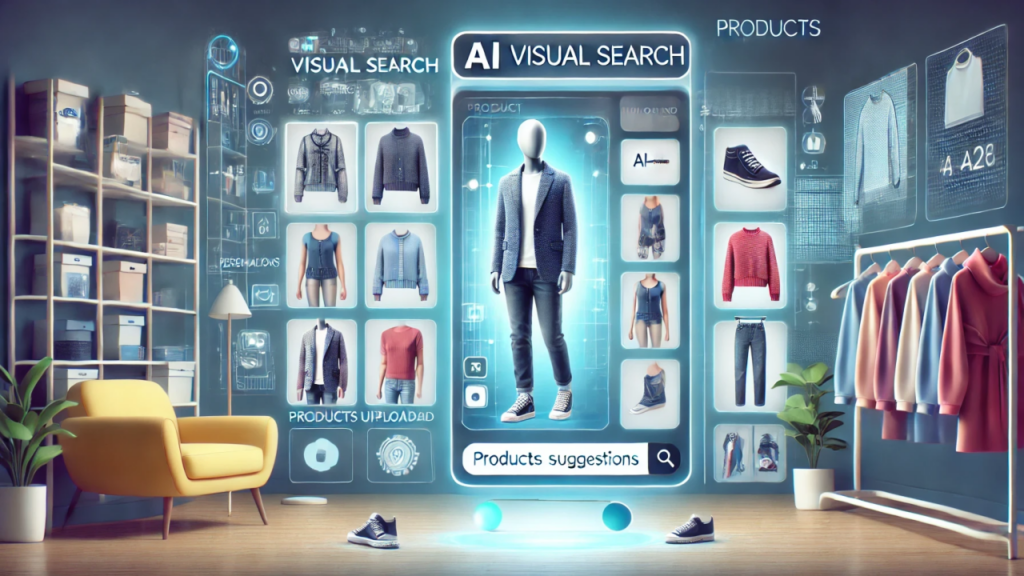
In the highly competitive world of e-commerce, customer attention is fleeting and brand loyalty is increasingly rare. Consumers are no longer satisfied with one-size-fits-all experiences—they expect retailers to anticipate their preferences, offer relevant suggestions, and provide seamless navigation across digital platforms. At the core of this transformation lies one of the most impactful technologies in retail today: Artificial Intelligence (AI) for personalized recommendations.
AI-powered recommendation systems have reshaped the way online retailers interact with customers, enabling them to tailor offerings and content to individual preferences. These systems not only enhance the customer experience but also significantly boost conversion rates, average order values, and long-term loyalty.
In this blog, we’ll explore how AI is revolutionizing personalized recommendations in e-commerce, the different techniques involved, the business benefits, implementation challenges, and examples from leading platforms.
Why Personalization Matters in E-commerce
Personalization is no longer a luxury—it’s a necessity.
According to McKinsey, 71% of consumers expect companies to deliver personalized interactions, and 76% get frustrated when this doesn’t happen. Personalization drives not only customer satisfaction but also purchasing behavior.
In e-commerce, personalized recommendations lead to:
- Higher engagement through relevant content and offers
- Increased conversions via cross-sell and upsell opportunities
- Improved customer retention through tailored experiences
- Stronger brand loyalty with consistent, curated communication
To achieve this level of customization at scale, businesses are increasingly turning to AI.
How AI Powers Personalized Recommendations
AI enables e-commerce businesses to analyze vast amounts of customer data in real-time and generate actionable insights. At the heart of AI-driven personalization are machine learning algorithms, data analytics, and natural language processing (NLP).
Let’s break down how AI recommendation engines work:
1. Data Collection
AI systems collect data from various sources, including:
- Browsing history
- Purchase behavior
- Wishlist and cart data
- Click-through rates
- Demographics and location
- Product ratings and reviews
This information feeds into AI models that recognize patterns and preferences.
2. Data Processing and Feature Extraction
Raw data is processed to extract meaningful features—such as a user’s affinity for certain brands, price ranges, or categories. AI models use these features to segment users or define behavioral clusters.
3. Recommendation Generation
AI algorithms analyze both user profiles and product attributes to generate real-time recommendations. These suggestions can be based on:
- Collaborative filtering
- Content-based filtering
- Hybrid systems
Let’s dive into these in more detail.
Types of AI Recommendation Systems
1. Collaborative Filtering
This method recommends products based on the preferences of similar users. If User A and User B both liked items X and Y, and User A also liked item Z, User B is likely to enjoy item Z as well.
Collaborative filtering is highly effective but has limitations when data is sparse—a problem known as the cold start problem.
2. Content-Based Filtering
This technique focuses on product attributes. If a customer likes a particular type of item (e.g., wireless earbuds under $100), the algorithm recommends similar products based on these features.
It works well even for new users but may lack diversity in recommendations.
3. Hybrid Systems
Most e-commerce giants today use a combination of collaborative and content-based filtering to maximize recommendation accuracy and coverage. These hybrid models are further enhanced by reinforcement learning and real-time user feedback.
Advanced AI Techniques in Personalized Recommendations
Modern AI recommendation systems go beyond simple filtering. They use sophisticated approaches like:
A. Deep Learning
Neural networks process unstructured data—such as images and text descriptions—to deliver more nuanced suggestions. For example, recommending fashion items with similar visual aesthetics.
B. Natural Language Processing (NLP)
NLP helps AI systems understand customer reviews, search queries, and chatbot interactions. It can gauge sentiment and refine product recommendations accordingly.
C. Context-Aware Recommendations
These systems factor in contextual data like time of day, season, device type, and even current trends to generate highly relevant recommendations.
D. Real-Time Personalization
AI can respond to a user’s real-time behavior—what they just clicked or how long they hovered over a product—and adjust recommendations dynamically.
Business Benefits of AI-Powered Recommendations
1. Increased Conversion Rates
Personalized product suggestions boost the likelihood of purchases by showing customers exactly what they’re looking for—sometimes before they even search for it.
2. Higher Average Order Value (AOV)
AI recommends complementary or upgraded products (cross-selling and upselling), encouraging customers to spend more.
3. Customer Retention and Loyalty
A personalized experience makes customers feel understood and valued, increasing repeat visits and long-term loyalty.
4. Reduced Bounce Rates
Relevant product displays reduce the chance that customers will leave a site prematurely, enhancing engagement and session duration.
5. Operational Efficiency
AI automates the process of personalization, eliminating the need for manual curation and allowing marketing teams to focus on strategy.
Challenges in Implementing AI Recommendations
While the advantages are substantial, implementing AI recommendation systems isn’t without its challenges.
A. Data Quality and Integration
AI models require clean, comprehensive, and well-structured data. Fragmented or inaccurate customer data can reduce the effectiveness of recommendations.
B. Cold Start Problem
For new users or new products, there may not be enough data to generate accurate recommendations. Mitigating this requires combining AI with demographic insights or initial onboarding questions.
C. Privacy Concerns
Collecting and analyzing user data raises concerns around data privacy and compliance (e.g., GDPR, CCPA). Clear policies and opt-in mechanisms are essential.
D. Algorithmic Bias
If historical data reflects bias (e.g., gender or region-specific trends), AI may perpetuate or even amplify that bias in recommendations.
E. Technical Complexity
Building a custom AI recommendation engine requires significant technical expertise, infrastructure, and ongoing maintenance. Many businesses opt for third-party tools or platforms to reduce the burden.
Examples of AI in Action: Real-World Case Studies
Amazon
Amazon’s recommendation engine reportedly drives over 35% of its sales. The system uses hybrid models that factor in purchase history, browsing behavior, and even what’s in the customer’s cart to suggest products in real-time.
Netflix
Although not an e-commerce platform, Netflix’s content recommendation system is often cited as a gold standard. It uses viewing history, ratings, and genre preferences to personalize the home screen for each user.
Sephora
Sephora uses AI to recommend beauty products based on user quizzes, past purchases, and product reviews. Their “Color IQ” system matches foundation shades using computer vision and personalization algorithms.
Spotify
Spotify’s AI recommendation engine curates personalized playlists like “Discover Weekly” based on listening behavior, time of day, and user preferences.
Getting Started: Tools and Platforms
Businesses don’t always need to build their own AI systems from scratch. There are many platforms offering customizable recommendation engines, including:
- Google Recommendations AI
- Amazon Personalize
- Salesforce Einstein
- Dynamic Yield
- Algolia Recommend
These platforms offer APIs and user interfaces that make it easier for non-technical teams to deploy personalized experiences at scale.
Conclusion
In a digital economy defined by choice and convenience, delivering personalized customer experiences is critical for standing out. AI-powered recommendation engines enable e-commerce businesses to meet rising expectations, drive sales, and foster loyalty—all while scaling effortlessly.
From understanding user preferences to generating real-time suggestions, AI transforms raw data into meaningful action. However, successful implementation depends on high-quality data, ethical considerations, and the right mix of technology and strategy.
As customer demands continue to evolve, so too must the tools businesses use to engage them. Investing in AI for personalized recommendations isn’t just a competitive advantage—it’s fast becoming a requirement for long-term success in the e-commerce landscape.