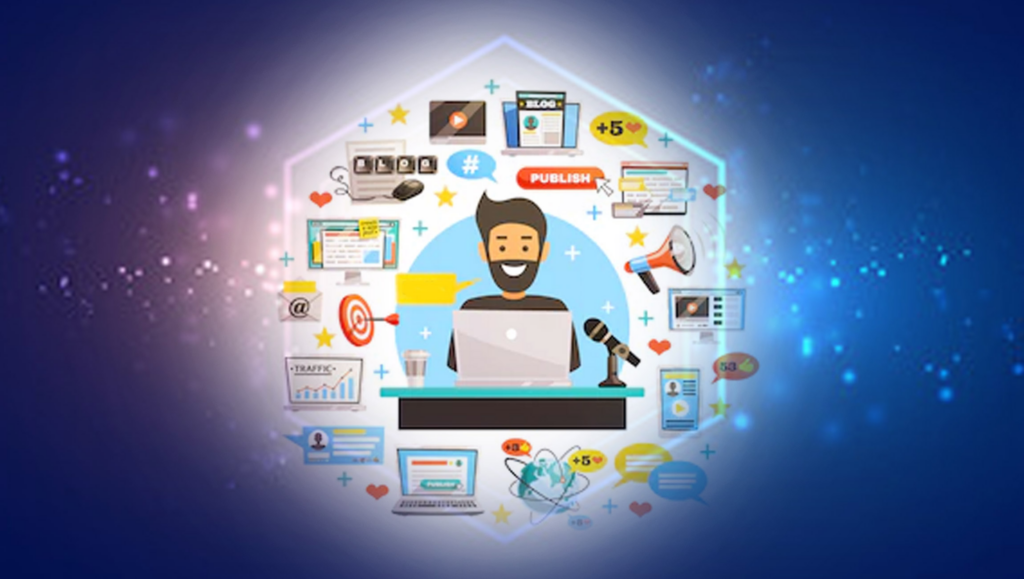
The explosion of digital content has transformed how we consume media—from streaming platforms and news aggregators to podcasts and social media feeds. While this abundance gives users unprecedented access to information and entertainment, it also creates a challenge: how to find content that is personally relevant and engaging amid overwhelming choice.
This is where Artificial Intelligence (AI) plays a transformative role. AI-powered personalization systems curate content uniquely tailored to each user’s preferences, habits, and behaviors, making media consumption more meaningful, efficient, and enjoyable. In this blog, we explore how AI enables personalized content curation, its underlying technologies, real-world applications, benefits, challenges, and future trends.
Understanding Personalized Content Curation
Personalized content curation refers to the process of selecting and organizing content for users based on their unique interests, behaviors, and consumption history. The goal is to deliver content that aligns with a user’s preferences, enhancing engagement and retention.
AI enhances this process by analyzing vast datasets, identifying patterns, and making intelligent recommendations in real time. It’s not just about suggesting similar content—it’s about understanding context, predicting preferences, and adapting over time.
Core AI Technologies Behind Content Personalization
1. Machine Learning (ML)
ML algorithms learn from user interactions—clicks, views, likes, shares, watch time—and use this data to recommend similar or relevant content. These systems continuously improve as more data is collected.
2. Natural Language Processing (NLP)
NLP allows machines to understand, analyze, and generate human language. It helps classify content, extract keywords, analyze sentiment, and match content with user intent.
3. Deep Learning
Deep learning models, particularly neural networks, power complex recommendation systems. These models can understand intricate relationships between user preferences and content attributes.
4. Collaborative Filtering
This technique predicts user preferences based on the preferences of similar users. If User A and User B share similar viewing patterns, content liked by User A may be recommended to User B.
5. Content-Based Filtering
This method recommends content based on features of the items themselves (e.g., genre, topic, length) and what a specific user has previously consumed.
Real-World Applications of AI in Content Curation
1. Streaming Platforms (Netflix, Spotify, YouTube)
AI powers personalized recommendations for videos, songs, and playlists. Netflix uses ML to suggest shows based on viewing history and preferences. Spotify’s “Discover Weekly” uses user behavior and collaborative filtering to suggest new music.
2. News Aggregators and Publishers
Apps like Google News and Flipboard use AI to present personalized news feeds. They consider reading habits, location, device usage, and user feedback to prioritize articles.
3. Social Media Feeds
Platforms like Facebook, Instagram, and TikTok employ AI to curate feeds that maximize user engagement. These systems rank content based on predicted interest, recency, and relationships.
4. E-learning Platforms
Online learning platforms such as Coursera or Duolingo personalize course recommendations and lesson plans using AI, adapting to each learner’s pace and knowledge gaps.
5. Podcast Platforms
Apps like Pocket Casts or Spotify Podcasts recommend shows based on listener preferences, browsing behavior, and topics of interest.
Benefits of AI-Driven Content Personalization
A. Enhanced User Engagement
Personalized content increases the time users spend on platforms by aligning with their interests, making media consumption more relevant and engaging.
B. Increased Retention and Loyalty
When users consistently find value in a platform’s recommendations, they’re more likely to return, subscribe, and remain loyal.
C. Efficient Discovery
AI helps users discover new content that they might not have otherwise encountered, broadening their interests while saving them time.
D. Real-Time Adaptability
AI adjusts recommendations dynamically based on real-time behavior, ensuring that suggestions stay current and contextually relevant.
E. Business Growth and Monetization
For content providers and media companies, personalized experiences lead to higher conversion rates, ad engagement, and customer satisfaction—translating into increased revenue.
Case Studies
Netflix: A Personalization Pioneer
Netflix’s recommendation engine is responsible for over 80% of the content watched on its platform. The company uses a combination of collaborative and content-based filtering, reinforced with deep learning models, to recommend shows and movies uniquely tailored to each user. It even customizes thumbnail images to increase click-through rates.
Spotify: Music to Your Taste
Spotify uses AI to analyze listening patterns, moods, and preferences to create personalized playlists like “Daily Mix” and “Release Radar.” Its algorithm tracks skip rates, playlist additions, and listening time to fine-tune recommendations.
The New York Times: Custom News Feeds
The Times employs machine learning to recommend articles based on reading behavior, geographic location, and previously consumed topics. This personalization has contributed to increased reader engagement and subscription retention.
Ethical and Technical Challenges
1. Privacy Concerns
AI personalization relies heavily on collecting and processing user data, raising concerns about how data is used, stored, and shared. Organizations must comply with regulations like GDPR and prioritize transparency and consent.
2. Filter Bubbles and Echo Chambers
Over-personalization can limit users to a narrow band of content, reinforcing existing beliefs and preventing exposure to diverse perspectives. This is especially problematic in news and social media.
3. Bias in Algorithms
If not carefully designed, AI models may reinforce societal or cultural biases present in training data. Ensuring fairness and inclusion in recommendation systems is an ongoing challenge.
4. Content Oversaturation
With AI constantly recommending new content, users may feel overwhelmed or develop decision fatigue. Platforms must strike a balance between variety and relevance.
5. Transparency and Explainability
Understanding how AI makes recommendations is essential for user trust. Many deep learning systems operate as “black boxes,” making it difficult to explain why a certain piece of content was suggested.
The Future of AI in Content Curation
A. Hyper-Personalization
Future systems will go beyond basic preferences, incorporating emotional state, time of day, device used, and even biometric data to create highly nuanced content recommendations.
B. Voice-Driven Personalization
With the rise of smart speakers and voice assistants, AI will curate audio content—music, news, or podcasts—based on conversational cues and voice tone.
C. Cross-Platform Personalization
AI will unify user preferences across platforms (mobile, desktop, smart TV) to deliver a consistent and personalized experience regardless of the device.
D. Real-Time Mood Detection
Emerging AI systems will detect user emotions through facial recognition or voice analysis and adjust content accordingly—for instance, suggesting uplifting music or calm meditations.
E. User-Controlled Personalization
Future platforms may empower users to fine-tune their recommendation algorithms, offering sliders or preferences to adjust content type, tone, or diversity.
Best Practices for Implementing AI in Content Curation
- Ensure Data Privacy
Collect only necessary data, store it securely, and communicate clearly how it will be used. Always comply with privacy laws. - Avoid Over-Personalization
Balance recommendations with diversity to prevent content stagnation and promote discovery. - Regularly Audit Algorithms
Review recommendation systems for bias, fairness, and effectiveness. Update models regularly with fresh, representative data. - Focus on User Experience
Keep the personalization process seamless and intuitive. Provide clear opt-in options and feedback mechanisms. - Combine Automation with Editorial Oversight
Human editors can complement AI to ensure content accuracy, cultural sensitivity, and alignment with brand values.
Conclusion
AI-powered personalized content curation is redefining how users interact with media. From entertainment and news to learning and social networking, AI helps surface the most relevant, engaging, and valuable content—enhancing user satisfaction while driving platform growth.
However, the power of AI must be wielded responsibly. Ethical considerations, user privacy, and algorithmic transparency are crucial to building trust and long-term value. As AI technologies evolve, the focus should not only be on what content to show, but also on how to empower users to shape their own media experiences.
Personalization is no longer a luxury—it’s the new standard. And AI is the engine making it possible.