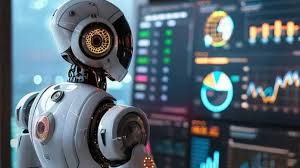
The digital age has introduced unprecedented volumes of data and an increasing need for rapid, accurate research. Traditional data analysis and research methods, though foundational, often struggle to keep pace with the exponential growth of information. Enter AI agents — intelligent, autonomous systems designed to analyze vast datasets, identify trends, generate insights, and support complex research tasks.
AI agents are redefining how individuals and organizations collect, interpret, and act on information. Their ability to automate tasks, detect patterns, and adapt to new data makes them indispensable in today’s research-driven world. Whether in scientific discovery, academic study, market intelligence, or social sciences, AI-powered tools are accelerating innovation and decision-making.
This blog delves into how AI agents are being applied in the realms of research and data analysis, highlighting their capabilities, advantages, use cases, challenges, and the future of intelligent data exploration.
Understanding AI Agents
AI agents are systems that observe environments, make decisions based on data, and perform tasks autonomously. Unlike simple scripts or rule-based automation, AI agents utilize advanced technologies such as:
- Machine learning
- Natural language processing (NLP)
- Knowledge graphs
- Data mining
- Deep learning
These tools allow AI agents to understand context, reason, learn from data, and refine their outputs over time. In research and analysis, this means they can sort through complex information far faster and more accurately than human researchers.
Key Functions of AI Agents in Research
1. Automated Literature Review
One of the most time-consuming tasks for researchers is sifting through existing literature. AI agents expedite this process by:
- Scanning thousands of articles, journals, and white papers
- Identifying relevant papers based on keywords, abstracts, and citations
- Extracting summaries and highlighting key findings
- Categorizing content by themes or research questions
This allows researchers to gain a comprehensive overview of a topic in a fraction of the time it would take manually.
2. Data Collection and Cleansing
AI agents can automatically gather data from diverse sources — surveys, social media, public datasets, and databases — and clean it for analysis. This includes:
- Removing duplicates
- Handling missing values
- Standardizing formats
- Identifying outliers
Accurate and clean data is foundational to reliable analysis, and AI excels at maintaining data quality across massive datasets.
3. Natural Language Processing for Textual Analysis
NLP enables AI agents to understand and analyze unstructured text data. In research, this is invaluable for:
- Sentiment analysis
- Topic modeling
- Entity recognition
- Opinion mining
Whether analyzing consumer feedback, news articles, academic papers, or interview transcripts, AI agents extract meaningful insights from text with minimal human intervention.
4. Statistical Analysis and Modeling
Beyond descriptive statistics, AI agents can perform complex predictive and prescriptive analyses. This includes:
- Regression modeling
- Time series forecasting
- Clustering and classification
- Anomaly detection
AI agents can identify hidden correlations, simulate outcomes, and even suggest hypotheses for further study, pushing the boundaries of what’s possible in research.
5. Visualization and Insight Delivery
AI doesn’t just crunch numbers; it also helps communicate findings effectively. With data visualization tools powered by AI, users can:
- Generate charts and dashboards automatically
- Highlight patterns and trends
- Create interactive reports
- Customize visual outputs based on user preferences
These capabilities make data insights more accessible and actionable for both researchers and stakeholders.
Applications Across Domains
Scientific Research
AI agents support drug discovery, climate modeling, genomics, and more by analyzing massive datasets at unprecedented speeds. For example, AI tools can analyze genetic sequences to identify mutations or model chemical interactions to predict drug efficacy.
Academic Research
Universities and scholars use AI to explore large bodies of literature, extract citations, and track academic trends. Tools like Semantic Scholar and Iris.ai assist with literature discovery, peer review analysis, and citation mapping.
Market Research
In the business world, AI agents monitor competitor activity, track consumer behavior, and analyze social trends. This helps companies make data-driven decisions about product development, marketing strategies, and customer engagement.
Social Science and Policy
AI helps researchers analyze societal behavior, study public opinion, and assess the effectiveness of policies. Tools can process survey data, social media activity, and public records to generate insights into social dynamics and human behavior.
Legal and Financial Research
AI agents rapidly scan legal documents, analyze case law, and identify regulatory trends. In finance, they process market data, predict economic shifts, and assist in fraud detection and investment strategy development.
Benefits of AI Agents in Research and Data Analysis
Speed and Efficiency
Tasks that once took weeks — such as reviewing literature or processing raw data — can now be completed in hours. AI agents operate 24/7, making continuous progress without fatigue or delay.
Accuracy and Consistency
AI eliminates human error in data entry, computation, and interpretation. It applies uniform standards across datasets, ensuring results are consistent and reproducible.
Scalability
AI agents can handle data from hundreds or even thousands of sources simultaneously. Whether analyzing tweets from millions of users or financial records across regions, AI scales effortlessly.
Cost Reduction
By automating repetitive and time-consuming tasks, AI reduces the need for large research teams and manual data processing, leading to significant cost savings.
Enhanced Insight Generation
AI doesn’t just analyze data — it identifies relationships, tests assumptions, and even suggests new avenues for exploration. It helps uncover insights that may be missed through traditional methods.
Challenges and Limitations
Data Bias
AI agents are only as good as the data they’re trained on. If the data is biased, the conclusions will be skewed. Ensuring data diversity and fairness is critical.
Interpretability
Some AI models, especially deep learning systems, act as “black boxes” — delivering results without explaining how they arrived there. In research, this lack of transparency can hinder trust and reproducibility.
Privacy and Ethics
Handling sensitive data, particularly in healthcare or social research, raises ethical concerns. AI systems must comply with regulations and respect participants’ rights and anonymity.
Over-Reliance on Automation
While AI enhances research, human judgment remains essential. Researchers must critically assess AI-generated insights and avoid blindly trusting algorithms.
Tools and Platforms Leveraging AI in Research
- IBM Watson: Offers AI tools for literature review, hypothesis generation, and data visualization.
- Scite.ai: Evaluates how scientific papers are supported or contradicted by other research.
- Tableau with AI Extensions: Combines data visualization with predictive analytics.
- Kensho: Used in finance to analyze markets and generate research reports.
- RapidMiner: Provides a platform for data mining and advanced analytics with user-friendly AI integration.
These platforms democratize AI by allowing researchers without coding expertise to leverage advanced analytics.
The Future of AI Agents in Research
As AI agents become more sophisticated, they will play increasingly proactive roles in research. Future developments may include:
- Autonomous Hypothesis Testing: AI that proposes, tests, and refines hypotheses with minimal human input.
- Cross-Disciplinary Insights: AI agents connecting findings from different domains to uncover new knowledge.
- Conversational Research Assistants: Voice or chat-based interfaces that help researchers navigate data and literature through natural conversation.
- Real-Time Global Research Networks: AI systems that monitor and synthesize worldwide research outputs in real time, keeping scholars constantly updated.
These advancements will transform the research landscape, fostering innovation, reducing barriers, and accelerating discovery.
Conclusion
AI agents are fundamentally reshaping how research and data analysis are conducted. By combining speed, scalability, and intelligence, these systems empower researchers to handle growing data complexity and derive meaningful insights more efficiently than ever before.
While challenges such as data bias and ethical concerns remain, the potential of AI in research is enormous. As tools continue to evolve and become more accessible, researchers from all fields will be better equipped to tackle complex questions, drive innovation, and contribute to global knowledge in transformative ways.