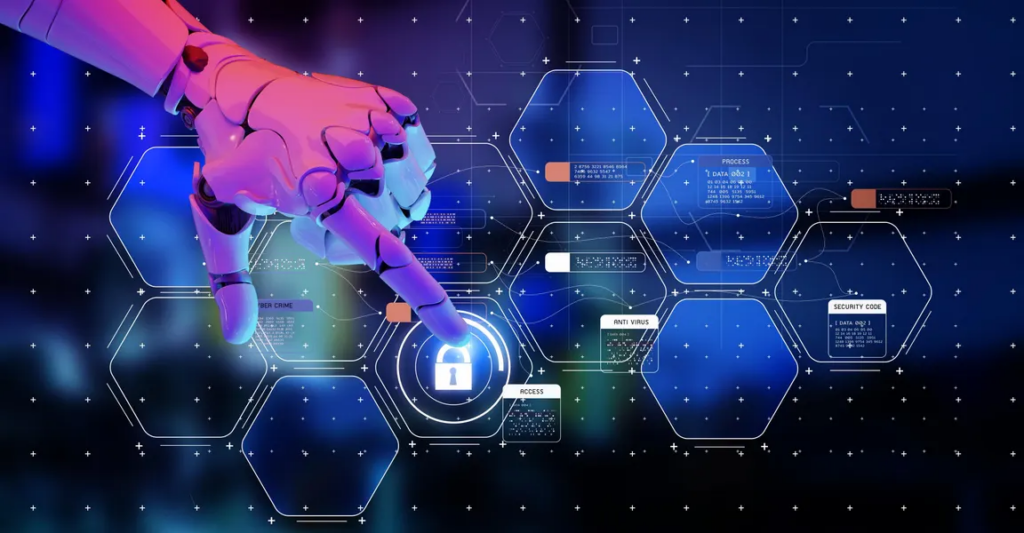
As artificial intelligence (AI) becomes increasingly integrated into modern business operations, it brings a dual-edged promise. On one side, AI offers the ability to automate complex tasks, analyze large volumes of data, and generate valuable insights. On the other, it introduces new vulnerabilities and challenges related to data security and privacy. In an era where data is a crucial asset, ensuring its protection is paramount.
AI systems thrive on data—personal, financial, behavioral, operational—and their effectiveness often hinges on access to large and varied datasets. This dependency on data raises important questions: Who controls the data? How is it stored, accessed, or shared? And what measures are in place to prevent misuse or breaches?
This blog explores the key data security and privacy concerns associated with AI adoption and provides actionable strategies to mitigate these risks effectively.
Why Data Security and Privacy Matter in AI
AI systems are only as trustworthy as the data they handle. From financial institutions and healthcare providers to marketing firms and government agencies, any organization using AI must address both regulatory and ethical considerations. Failing to do so can lead to:
- Loss of customer trust
- Legal and financial penalties
- Reputational damage
- Inaccurate or biased outcomes
As a result, building privacy and security into AI systems is not optional—it’s essential.
Common Data Security and Privacy Concerns in AI
1. Data Breaches and Unauthorized Access
AI systems often rely on centralized datasets, making them a target for cybercriminals. If not properly secured, these datasets can be compromised, exposing sensitive information such as customer identities, financial details, or proprietary business information.
2. Data Leakage through AI Models
Machine learning models can inadvertently “memorize” sensitive data during training. In some cases, attackers can extract portions of the original data by querying the model—a risk known as model inversion.
3. Bias and Discrimination
AI systems trained on historical data may unintentionally learn and replicate discriminatory patterns, leading to biased outcomes in areas like hiring, lending, or law enforcement. Such outcomes not only violate ethical principles but also privacy rights when individuals are profiled without consent.
4. Lack of Transparency (Black Box Models)
Many AI algorithms, particularly deep learning models, operate as “black boxes,” making it difficult to understand how decisions are made. This opacity complicates privacy assessments and regulatory compliance, especially when individuals have a legal right to explanation.
5. Data Aggregation and Re-identification
AI models often aggregate data from multiple sources to find patterns. Even when data is anonymized, combining datasets can lead to re-identification of individuals, especially when location, behavior, or other quasi-identifiers are involved.
6. Third-Party Risk
Many organizations rely on third-party AI vendors, which introduces additional risks. If vendors lack robust security practices, they can become entry points for attackers or misuse customer data.
Key Principles to Mitigate Privacy and Security Risks
Organizations must embed privacy and security into the AI lifecycle—from data collection to deployment and beyond. Below are foundational principles and strategies to guide responsible AI use.
1. Data Minimization and Purpose Limitation
Only collect and use the data necessary for the AI system to function. Avoid data hoarding, which increases the risk of breaches and non-compliance.
Best practices:
- Define the specific purpose for data collection.
- Avoid using sensitive data unless absolutely necessary.
- Regularly audit data sets to remove outdated or irrelevant data.
2. Privacy by Design
Privacy should not be an afterthought. Incorporate privacy features at every stage of AI development, including design, data collection, model training, deployment, and maintenance.
Privacy by design involves:
- Embedding encryption, access controls, and anonymization techniques.
- Designing systems that allow users to control their data (e.g., opt-in/opt-out features).
- Incorporating explainability to clarify how data is used and decisions are made.
3. Data Anonymization and Differential Privacy
To reduce risks, data should be anonymized or de-identified before being used to train AI models. Advanced techniques like differential privacy ensure that individual data points cannot be traced back, even after analysis.
Differential privacy techniques:
- Add “noise” to datasets to prevent exact identification.
- Ensure that outputs from AI systems cannot reveal private information.
4. Federated Learning
Instead of centralizing sensitive data, federated learning enables AI models to be trained across multiple decentralized devices or servers. The model learns from the data locally and then shares updates with the central system.
Benefits:
- Raw data never leaves the local environment.
- Reduces the risk of exposure during data transmission.
- Ideal for applications involving personal devices like smartphones.
5. Robust Access Control and Encryption
Security controls such as encryption and access management are critical for protecting both data and AI models from unauthorized access.
Best practices:
- Encrypt data at rest and in transit using strong protocols.
- Implement multi-factor authentication (MFA) for system access.
- Use role-based access control (RBAC) to limit data exposure based on roles.
6. Regular Auditing and Monitoring
Conduct regular audits of AI systems to ensure compliance with privacy standards and detect any anomalies or vulnerabilities. Monitoring is crucial for spotting threats early.
Audit checkpoints include:
- Data access logs
- Model outputs and decision logs
- Third-party compliance reviews
- Bias and fairness testing
7. Explainable AI (XAI)
Explainability helps demystify AI decision-making processes, especially in high-stakes scenarios like healthcare or finance. This builds user trust and aids in compliance with privacy regulations.
Approaches to XAI:
- Use interpretable models (e.g., decision trees) where feasible.
- Apply techniques like LIME or SHAP to explain black-box models.
- Provide clear explanations to users about how their data influences decisions.
8. Ethical AI Governance
Establish a dedicated AI ethics or governance board to oversee responsible use of data and AI systems. Governance frameworks ensure that ethical and legal considerations are not overlooked.
Key responsibilities:
- Approve use cases and data sources.
- Review algorithmic fairness and bias.
- Define accountability for AI decisions.
9. Regulatory Compliance
AI systems must comply with data protection regulations such as:
- GDPR (General Data Protection Regulation) – EU
- CCPA (California Consumer Privacy Act) – US
- DPDP Act (Digital Personal Data Protection Act) – India
- HIPAA (Health Insurance Portability and Accountability Act) – US (Healthcare)
Compliance actions:
- Obtain explicit consent for data use.
- Allow users to request data deletion or correction.
- Be transparent about data usage and storage locations.
Building Trust through Transparency
One of the biggest challenges in AI adoption is building user trust. To overcome this, companies must be open about how data is collected, processed, and used. Transparency not only reassures users but also strengthens brand reputation.
Ways to build trust:
- Publish clear data policies and AI usage statements.
- Offer users control over their data through dashboards or settings.
- Disclose any partnerships with third-party AI vendors and their data practices.
The Role of AI in Enhancing Security
Interestingly, AI can also be used to enhance cybersecurity and privacy protections. Organizations are increasingly deploying AI to detect and respond to threats in real time.
Examples include:
- Anomaly Detection: AI monitors network traffic to identify unusual activity, such as unauthorized access or malware.
- Behavioral Analytics: AI assesses user behavior patterns to detect potential insider threats.
- Automated Threat Response: AI can automatically isolate affected systems or block malicious IPs, reducing response time.
In this way, AI becomes both a tool to protect data and a system that must itself be secured.
Conclusion
The integration of AI into business operations introduces undeniable advantages—but it also brings a heightened responsibility to protect data and preserve privacy. From securing datasets and managing access to ensuring algorithmic fairness and regulatory compliance, organizations must take a comprehensive, proactive approach.
By adopting best practices like data minimization, encryption, federated learning, and privacy-by-design principles, businesses can not only protect themselves from data breaches and legal penalties but also build lasting trust with users and stakeholders.
Ultimately, the future of AI hinges on its responsible use. Addressing data security and privacy concerns today ensures that AI technologies can be leveraged confidently and ethically tomorrow.