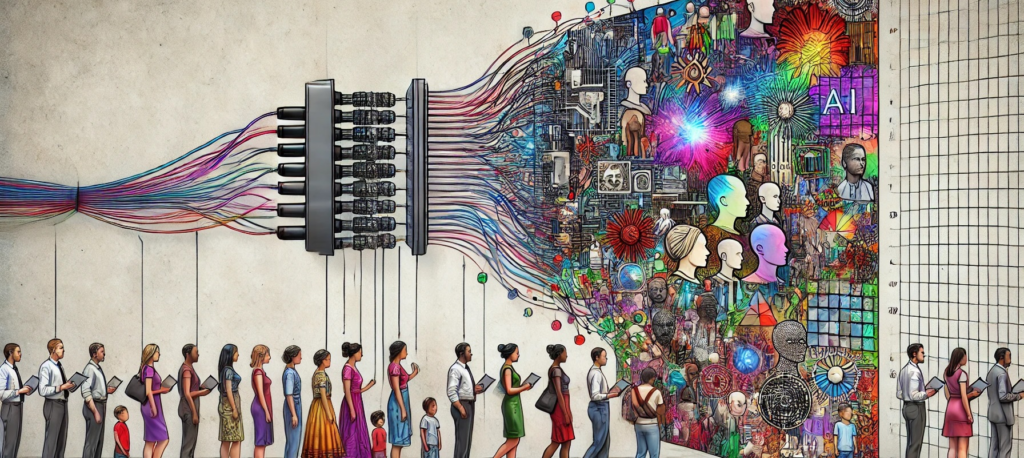
Generative AI has emerged as a revolutionary tool in content creation—producing everything from realistic images and videos to human-like text and speech. However, along with its vast potential comes a significant challenge: bias.
Bias in generative AI models can perpetuate and even amplify existing societal stereotypes and discrimination. From facial image generators producing racially skewed results to text generators reinforcing gender stereotypes, the problem is widespread and deeply embedded.
In this blog, we’ll explore:
- What bias in generative AI means
- Where it comes from
- The impact of biased outputs
- Real-world examples
- Practical steps toward mitigating bias
- The role of policy and ethics in shaping fairer AI systems
What Is Bias in Generative AI?
Bias in generative AI refers to systematic and unfair outcomes produced by AI systems due to imbalances or prejudices in the data or design of the model. It often reflects societal inequities—around race, gender, age, culture, religion, or economic status—and may lead to unfair treatment or representation of certain groups.
Types of Bias in Generative AI
- Data Bias: Occurs when training data underrepresents or misrepresents certain groups.
- Algorithmic Bias: Introduced during the model development process through flawed assumptions or design choices.
- Societal Bias: Embedded in the way people behave and how that behavior is recorded in the data.
- Labeling Bias: Arises when humans annotate data in ways that reflect personal or cultural biases.
Where Does Bias Come From?
Generative AI models are typically trained on massive datasets collected from the internet. These datasets include books, articles, forums, images, and videos. While vast, the web isn’t an unbiased space. It reflects the views, behaviors, and stereotypes prevalent in society.
Key sources of bias:
- Historical inequalities in literature, media, or visual data.
- Underrepresentation of marginalized groups in source datasets.
- Cultural dominance, where Western norms overpower others.
- Toxic online content, which includes hate speech, stereotypes, or misinformation.
Even a highly technical, neutral-sounding dataset can carry deep cultural assumptions. And since generative AI is probabilistic—it reproduces patterns—it can unintentionally reinforce harmful stereotypes.
Why AI Bias Is a Serious Problem
Unchecked bias in generative AI can lead to:
- Misinformation: Generating or spreading false narratives about people or cultures.
- Discrimination: Reinforcing societal prejudice or excluding marginalized groups.
- Erosion of trust: Reducing confidence in AI systems and the organizations that deploy them.
- Ethical violations: Failing to align with principles of fairness, justice, and inclusion.
- Legal consequences: Facing regulation or lawsuits for discriminatory outcomes.
How to Detect Bias in Generative AI
Before fixing bias, it must be identified. Here are some approaches:
1. Auditing Training Data
Analyze datasets for representation gaps across race, gender, geography, age, and ability. Are all communities fairly represented?
2. Fairness Testing
Use benchmarks or fairness evaluation tools to test outputs across diverse inputs. For instance:
- How does an image generator depict “a wedding” in different cultural contexts?
- Does the model associate particular professions with specific genders?
3. Human-in-the-Loop Evaluation
Involve diverse testers to evaluate AI outputs for fairness, inclusivity, and respectfulness. Human feedback is vital in assessing subtle forms of bias.
4. Explainability Tools
Tools like SHAP or LIME help explain why models make certain decisions, which can help identify discriminatory patterns.
Strategies to Mitigate Bias
Reducing bias in generative AI isn’t just a technical challenge—it also requires ethical commitment and social awareness.
1. Curating Diverse and Inclusive Datasets
- Balance datasets with images, texts, and voices from a wide range of demographics.
- Include non-Western cultures, languages, and social contexts.
- Remove or downweight harmful, toxic, or biased content.
2. Bias-Aware Model Training
- Use debiasing techniques, such as adversarial training or fairness constraints.
- Implement differential weighting, so minority examples are emphasized during learning.
- Incorporate counterfactual data augmentation to present alternate realities (e.g., female CEOs, male nurses).
3. Prompt Engineering with Equity in Mind
- Carefully craft prompts to reduce stereotypes or bias amplification.
- Use inclusive, neutral language when prompting models.
4. Post-Processing and Filtering
- Apply output filters to detect and block biased or offensive content.
- Allow users to flag problematic outputs for review and refinement.
5. Involving Diverse Teams
- AI development teams should include individuals from various backgrounds to ensure more inclusive perspectives.
- Diverse teams are better at spotting bias that may be invisible to others.
The Role of Policy and Regulation
While technology companies can take steps, governments and global institutions must also step in to set guardrails.
Current Developments:
- The EU AI Act includes requirements around non-discrimination and fairness in AI.
- The US Blueprint for an AI Bill of Rights advocates for algorithmic fairness and data protections.
- UNESCO and OECD have proposed frameworks for ethical AI development.
Key policy considerations:
- Transparency in data sources and model behavior
- Accountability mechanisms for harm
- Requirements for fairness audits
- Rights to human appeal or redress
Generative AI holds immense creative potential, but with great power comes great responsibility. Bias, if left unchecked, can undermine trust, reinforce inequality, and cause real harm to individuals and communities.
Solving this problem isn’t a one-time fix—it requires ongoing work from developers, researchers, policymakers, and society at large. By investing in transparent processes, inclusive datasets, ethical development, and strong regulatory frameworks, we can move toward an AI-powered future that is fairer and more just for everyone.
In a world shaped by AI, ensuring equity isn’t optional—it’s essential.