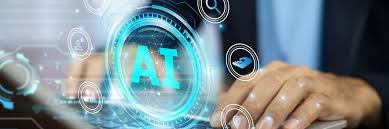
Artificial intelligence is no longer just a futuristic concept or the exclusive playground of tech giants. Across industries, companies—both large and small—are integrating AI into their operations to drive innovation, efficiency, and customer satisfaction. But while the success stories grab headlines, the road to effective AI implementation is paved with practical lessons that all businesses can learn from.
By examining companies that are leading the way in AI adoption, we can extract actionable insights to help others navigate their own AI transformation journeys. From overcoming cultural resistance to ensuring data quality, these lessons provide a roadmap for sustainable and responsible AI integration.
Start with a clear business problem, not the technology
One of the most common mistakes businesses make is adopting AI for the sake of appearing innovative, rather than solving a specific problem. Leading companies like Amazon and Netflix didn’t just deploy AI because it was trendy—they used it to solve operational challenges like personalized recommendations, logistics optimization, and customer service automation.
Companies that succeed with AI begin with a well-defined use case. For example, John Deere used AI not to experiment but to improve agricultural productivity by predicting machine failure and optimizing planting patterns. The value was clear, measurable, and aligned with their business model.
Invest in quality data before diving into AI
A powerful AI model is only as good as the data it learns from. Companies that lead in AI adoption often have one thing in common: they take data management seriously. From labeling and cleaning to storage and access, the data strategy is foundational.
Take the example of Procter & Gamble. Before integrating AI into their supply chain, they invested in unifying disparate data sources, standardizing formats, and implementing robust governance. As a result, their AI systems made more accurate demand forecasts and improved inventory control.
Lesson learned: prioritize data readiness. AI success is rarely about choosing the best algorithm—it’s about feeding the algorithm with high-quality, relevant, and timely data.
Create cross-functional AI teams
AI is not just an IT initiative. Successful implementation requires collaboration between data scientists, domain experts, engineers, and business leaders. This cross-functional approach ensures the technology solves the right problems and fits seamlessly into workflows.
At Airbnb, cross-functional teams consisting of product managers, software engineers, and data scientists work together to deploy AI solutions that personalize search results, detect fraud, and optimize pricing. This synergy between departments accelerates development and ensures user-centric outcomes.
For companies new to AI, this means breaking down silos. AI projects should not be handed off solely to tech teams—they need business context to be effective.
Don’t underestimate the importance of change management
Even the most advanced AI system can fail if employees are reluctant to adopt it. Leading companies recognize that change management is as critical as the technology itself. This includes educating teams about AI, addressing fears of job displacement, and ensuring transparency.
Microsoft, for instance, focused heavily on internal training as it integrated AI across its enterprise solutions. They launched upskilling programs, workshops, and communication campaigns to help employees understand how AI would augment—not replace—their roles.
The lesson is clear: a people-first approach helps mitigate resistance and builds a culture where AI is embraced, not feared.
Focus on ethical AI and transparency
With great power comes great responsibility. As AI systems begin to influence hiring, lending, policing, and other sensitive areas, companies must be mindful of ethical implications. Bias in training data, lack of explainability, and privacy concerns can quickly derail an AI initiative and harm brand trust.
IBM has made AI ethics a cornerstone of its AI strategy. The company publishes transparent reports on how its models are trained and encourages responsible AI use through internal audits and third-party reviews.
Building trust in AI systems requires a commitment to ethical development, clear documentation, and human oversight.
Build for scalability from the beginning
Many companies start with pilot AI projects that show promising results, but then struggle to scale. Leaders in the AI space consider scalability from the outset. This includes choosing flexible cloud infrastructure, modular architectures, and reusable models.
For example, Uber didn’t build a separate AI solution for every use case. Instead, they developed a centralized platform called Michelangelo that allows teams to build, deploy, and manage machine learning models at scale.
Even if your business is starting small, designing your AI initiatives with long-term scalability in mind will save time and cost in the future.
Adopt a test-and-learn mindset
AI is an evolving field. Even the best models need refinement, and not every use case will yield high returns immediately. Leading companies approach AI with a mindset of experimentation. They run pilots, A/B tests, and continuous iterations to improve outcomes over time.
Zara, the global fashion retailer, uses AI to track fashion trends and optimize inventory. Instead of overhauling its entire process at once, the company began by piloting AI in selected regions, measuring impact, and refining the models before a broader rollout.
In AI, perfection should not be the goal at launch. Focus on continuous learning and improvement.
Leverage third-party tools but retain strategic ownership
With the abundance of AI-as-a-service platforms available today, companies don’t need to build everything from scratch. Cloud providers like AWS, Azure, and Google Cloud offer plug-and-play AI services that can handle everything from speech recognition to fraud detection.
However, leading companies don’t outsource their AI strategy entirely. They use third-party tools for efficiency but retain ownership of their models, data, and long-term vision.
For example, fintech startups in India often use Google’s AutoML or AWS’s Rekognition to get started quickly but eventually build internal capabilities to ensure customizability and data privacy.
Balance automation with human input
AI thrives when it complements human intelligence rather than replacing it. The most effective AI systems are designed with a human-in-the-loop approach. This ensures that edge cases are handled correctly and users remain in control.
For instance, LinkedIn’s AI suggests job recommendations and candidate matches, but recruiters still make final decisions. This balance improves efficiency without compromising accuracy or fairness.
Companies should view AI as a collaborator, not a substitute.
Look beyond cost savings
While AI can certainly reduce operational costs, its true value lies in innovation and new revenue opportunities. Companies that lead in AI adoption don’t limit its use to automation—they also use it to create entirely new products, services, and business models.
Spotify’s AI-powered recommendation engine isn’t just a feature—it’s a core part of the platform that drives user engagement and subscription growth. Similarly, Tesla uses AI not just for driving but for transforming the entire transportation experience.
By thinking beyond savings and toward value creation, companies can maximize the ROI of AI investments.
Conclusion: AI leadership is a mindset
Adopting AI successfully is not about having the largest budget or the biggest tech team. It’s about clarity of purpose, a culture of innovation, and a willingness to learn from both successes and failures. The companies leading the way in AI adoption have demonstrated that strategic planning, strong leadership, and ethical execution are what truly set them apart.
As more businesses follow in their footsteps, these lessons will become increasingly valuable. Whether you’re a startup or an established enterprise, the path to effective AI integration begins with learning from those who’ve done it well—and applying those insights to your unique journey.