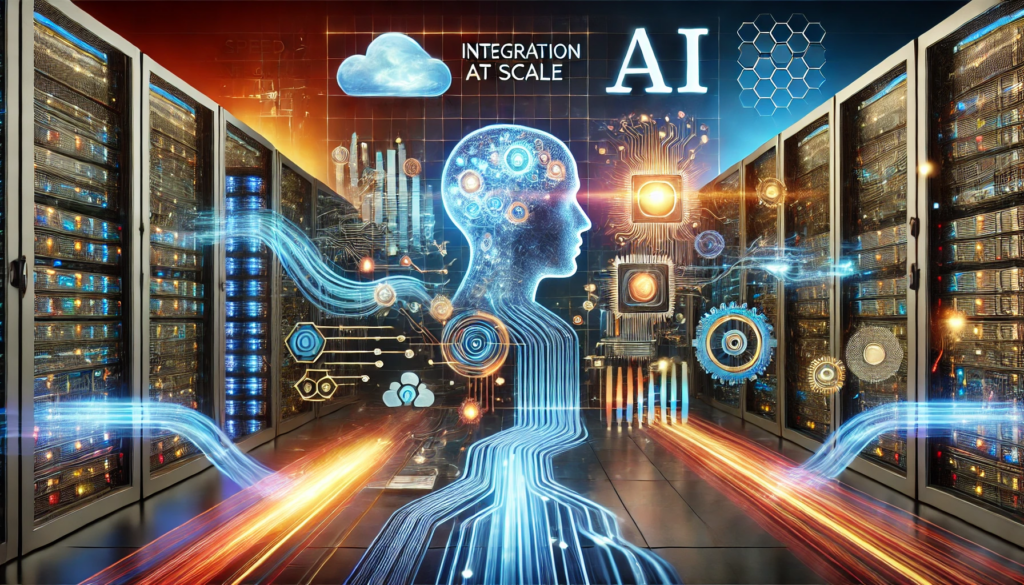
Artificial Intelligence (AI) is no longer a futuristic concept confined to research labs or tech giants. It is rapidly becoming an essential tool for businesses of all sizes and across all industries. From customer service automation and predictive analytics to intelligent manufacturing and personalized marketing, AI is reshaping how we work, make decisions, and deliver value. However, for many organizations, especially small to medium-sized enterprises, the idea of integrating AI can seem intimidating.
This guide provides a practical roadmap for organizations beginning their journey toward AI integration. It covers everything from initial considerations to implementation strategies, with a focus on making AI adoption both manageable and beneficial.
Why AI Integration Matters
Before diving into the practical steps, it’s essential to understand why AI integration is worth pursuing:
- Increased efficiency: Automating routine tasks allows employees to focus on higher-value work.
- Data-driven decisions: AI enables businesses to leverage data for more informed decisions.
- Competitive advantage: Early AI adopters often outperform competitors in innovation and customer experience.
- Cost savings: Intelligent systems can help reduce operational and labor costs over time.
Despite its potential, AI should not be viewed as a quick fix. Success requires strategic alignment, clear goals, and thoughtful execution.
Step 1: Define Your AI Objectives
The first step in AI integration is defining what you want to achieve. Start by identifying specific problems or inefficiencies within your organization where AI could add value.
Ask yourself:
- Are we spending too much time on repetitive tasks?
- Do we have large volumes of data that we’re not leveraging?
- Could we improve customer interactions or satisfaction using automation?
- Are there insights we’re missing in our business operations?
Clearly defined objectives will guide your AI journey. For example:
- Automate customer service inquiries using a chatbot.
- Use AI to forecast sales trends based on historical data.
- Improve quality control in manufacturing using computer vision.
These goals should be realistic and measurable, forming the basis of your AI strategy.
Step 2: Evaluate Your Readiness
Before diving into implementation, assess your organizational readiness. Successful AI integration depends on several factors:
a. Data Infrastructure
AI systems rely on data. Do you have:
- Access to quality, well-organized data?
- Systems in place to collect, store, and retrieve data?
- A plan to address data privacy and security?
If not, your first investment might need to be in data management systems, such as customer relationship management (CRM), enterprise resource planning (ERP), or cloud-based databases.
b. Skill Sets
Do your employees have the technical expertise to work with AI? You may need to:
- Upskill current staff through training programs.
- Hire AI specialists, data scientists, or machine learning engineers.
- Collaborate with external consultants or vendors.
c. Technological Ecosystem
Evaluate your existing IT infrastructure. Is it compatible with AI tools? Can it scale with future needs? Legacy systems might limit your ability to adopt advanced AI tools without upgrades or integration layers.
Step 3: Start Small with Pilot Projects
Many companies make the mistake of trying to implement large-scale AI systems right away. A better approach is to start small, testing AI in a low-risk area through a pilot project.
Examples of good pilot projects include:
- Implementing a customer support chatbot for common queries.
- Using machine learning to analyze historical sales data for demand forecasting.
- Applying natural language processing (NLP) to extract insights from customer reviews.
When designing a pilot project:
- Choose a clearly defined problem.
- Set success criteria (e.g., reduce response time by 30%).
- Use a limited dataset or scope.
- Ensure stakeholders are on board with realistic expectations.
Once the pilot shows promising results, it can be scaled or adapted for broader use.
Step 4: Choose the Right Tools and Partners
The AI landscape is filled with tools, platforms, and service providers. Your choices depend on your goals, budget, and technical expertise.
a. Off-the-shelf AI Solutions
These are pre-built AI applications that are easy to deploy and require minimal customization:
- Chatbots: e.g., Dialogflow, Zendesk AI
- Predictive analytics: e.g., Salesforce Einstein, Microsoft Azure ML
- Computer vision: e.g., Amazon Rekognition, Google Vision AI
They are ideal for businesses without large IT teams and offer quick wins.
b. Custom AI Development
For specialized needs, developing custom AI models may be necessary. This requires:
- Data scientists and ML engineers
- Cloud computing resources
- Longer development time
You can also partner with AI consultancies or universities for help with custom models and integration strategies.
Step 5: Address Ethical and Legal Considerations
AI systems, while powerful, also raise concerns about bias, fairness, and transparency. Before deploying any system:
- Ensure your data is unbiased and representative.
- Use explainable AI models wherever possible.
- Comply with data protection laws like GDPR, HIPAA, or relevant national regulations.
Transparency about AI use is also important. Let users know when they are interacting with an AI system, and provide them with human escalation options if needed.
Step 6: Prepare Your Workforce
AI adoption often causes concerns among employees about job displacement. Managing this transition with transparency and training is crucial.
Actions to consider:
- Communicate clearly about how AI will support—not replace—workers.
- Provide training in AI literacy and related technologies.
- Identify new roles AI may create, such as data analysts, AI trainers, or automation supervisors.
An inclusive strategy that empowers employees can turn AI integration into a workforce advantage.
Step 7: Monitor and Iterate
AI implementation is not a one-time event. It requires continuous monitoring, evaluation, and improvement.
Key metrics to track:
- Operational efficiency improvements
- Cost reductions
- User satisfaction
- Error or failure rates in AI outputs
Gather feedback from users, identify issues, and iterate your models and processes regularly. Over time, AI systems can become more accurate, reliable, and valuable to your business.
Real-World Example: AI in Retail
A mid-sized retail company wanted to improve customer engagement on its e-commerce site. After evaluating its needs, the team started with a chatbot pilot project using an off-the-shelf NLP platform. The chatbot handled 60% of customer inquiries within three months, freeing up human agents to focus on complex queries.
The company then scaled AI use to predict inventory needs using past sales data, reducing overstocking by 25%. Employees were trained to monitor AI recommendations and adjust campaigns accordingly. The result was improved customer satisfaction, reduced operational costs, and a data-driven culture across departments.
Common Challenges and How to Overcome Them
- Lack of data or poor data quality
- Start by improving your data collection and cleaning practices.
- High costs of AI adoption
- Use cloud-based solutions and open-source tools to reduce initial investment.
- Fear of automation among staff
- Offer reskilling programs and clearly communicate the human-AI collaboration model.
- Undefined success metrics
- Set measurable goals before deployment to evaluate effectiveness.
Conclusion
AI is no longer just for tech giants. With the right strategy, tools, and mindset, organizations of all sizes can start reaping the benefits of AI. The key is to begin with clear objectives, start small, and focus on building the right infrastructure and culture for long-term success.
Integrating AI into your business is not about replacing people—it’s about augmenting human capabilities, unlocking new insights, and enabling smarter, faster decisions. By following the practical steps outlined in this guide, you can confidently take your first steps toward AI integration and position your organization for a more intelligent future.