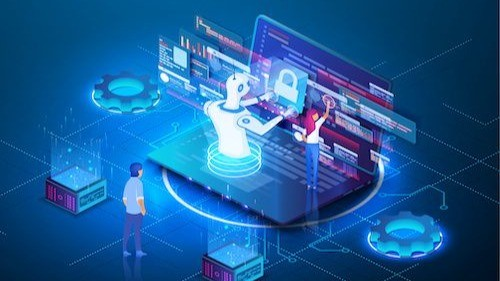
Artificial Intelligence (AI) has become an indispensable part of modern business operations and societal functions. From personalized recommendations and credit scoring to medical diagnostics and hiring, AI systems are being entrusted with decisions that significantly impact people’s lives. However, this growing reliance on AI also brings critical challenges—particularly in ensuring fairness and transparency.
When not designed or monitored carefully, AI can perpetuate existing inequalities, make opaque decisions, and ultimately erode trust. Ensuring fairness and transparency in AI-driven processes is not just a technical challenge but a societal imperative. In this blog, we will explore what fairness and transparency mean in AI, why they matter, the challenges involved, and actionable strategies for organizations to adopt responsible AI practices.
What Is Fairness in AI?
Fairness in AI refers to the absence of bias or discrimination in the outcomes produced by AI systems. It is about ensuring that individuals or groups are not unfairly disadvantaged or privileged based on race, gender, age, disability, or other protected attributes.
There are multiple interpretations of fairness in the AI context:
- Demographic Parity: Equal outcomes across different groups.
- Equal Opportunity: Equal true positive rates across groups.
- Individual Fairness: Similar individuals receive similar outcomes.
- Fair Treatment: Avoiding disparate impacts even if the model is accurate overall.
Each of these definitions highlights the complexity of fairness—what may seem fair in one context might not be in another. Thus, organizations need to carefully define fairness based on the specific use case and its societal implications.
What Is Transparency in AI?
Transparency in AI refers to the ability to understand, interpret, and explain how an AI system makes decisions. Transparency helps stakeholders—including users, regulators, and developers—gain insights into the system’s logic, data, and assumptions.
Transparency includes:
- Model Interpretability: Explaining how a model arrives at its decisions.
- Data Provenance: Understanding where the training data comes from and how it was collected.
- Decision Justification: Providing reasons behind a specific outcome or recommendation.
Without transparency, users and regulators cannot assess whether an AI system is acting fairly or ethically.
Why Fairness and Transparency Matter
1. Building Trust
Users are more likely to accept and adopt AI solutions if they understand how decisions are made and are confident the system treats them fairly.
2. Regulatory Compliance
Laws such as the EU’s General Data Protection Regulation (GDPR) and upcoming AI regulations require organizations to explain AI decisions and avoid discriminatory practices.
3. Avoiding Harm
AI-driven systems can amplify societal biases if not designed with fairness in mind, potentially harming vulnerable groups and widening inequality.
4. Reputation Management
An organization found to be using biased or opaque AI can suffer reputational damage, loss of customer trust, and legal penalties.
5. Improved Performance
Fair and transparent systems tend to generalize better, avoid hidden risks, and perform more robustly across diverse use cases.
Real-World Examples of Failures in Fairness and Transparency
- Hiring Tools
AI tools used by companies to screen resumes have, in some cases, favored male candidates over female ones due to biased historical data. - Facial Recognition
Studies have shown that facial recognition systems have higher error rates for people of color and women, leading to wrongful arrests and discrimination. - Loan Approval Algorithms
Credit scoring algorithms may deny loans disproportionately to certain racial groups if their training data reflects past discriminatory practices.
These examples show the potential consequences when AI systems lack fairness and transparency.
Challenges in Achieving Fairness and Transparency
Despite their importance, fairness and transparency in AI are difficult to achieve due to several inherent challenges:
- Ambiguity in Definitions: There is no single universally accepted definition of fairness.
- Trade-offs Between Accuracy and Fairness: Optimizing for fairness may lead to a reduction in model accuracy.
- Complexity of AI Models: Deep learning models can be so complex that their internal workings are difficult to interpret.
- Lack of Representative Data: Biased or incomplete data can skew model predictions.
- Dynamic Environments: Fairness requirements can change over time as societal norms evolve.
Strategies to Ensure Fairness in AI
1. Bias Detection and Mitigation
Regularly test AI models for disparate impact across demographic groups. Use statistical techniques to detect bias and apply mitigation strategies such as:
- Re-sampling or re-weighting the training data
- Fair representation learning
- Post-processing outcomes to balance metrics
2. Diverse and Inclusive Datasets
Ensure training data is representative of the population the model will serve. This includes collecting data across different ages, ethnicities, genders, socioeconomic statuses, and geographies.
3. Inclusive Development Teams
Include diverse perspectives during AI system development. Teams with varied backgrounds are more likely to identify potential fairness issues early in the design process.
4. Stakeholder Consultation
Engage with the communities and individuals who will be affected by AI decisions. Understand their concerns and incorporate their feedback into the design process.
5. Fairness-Aware Metrics
Use fairness-aware performance metrics in model evaluation, such as:
- Disparate Impact Ratio
- Equalized Odds
- Predictive Parity
These metrics help track fairness and guide improvements during model training.
Strategies to Ensure Transparency in AI
1. Use Interpretable Models
When possible, choose simpler models such as decision trees or linear regressions for high-stakes applications where interpretability is crucial.
2. Apply Explainable AI (XAI) Techniques
When using complex models, leverage explainability tools like:
- LIME (Local Interpretable Model-Agnostic Explanations): Generates interpretable models around individual predictions.
- SHAP (SHapley Additive exPlanations): Assigns importance to features contributing to model predictions.
These tools help users and stakeholders understand how decisions are made.
3. Maintain Documentation
Thoroughly document:
- Model assumptions and architecture
- Training data sources
- Performance metrics
- Decisions about feature selection
This documentation supports audits, troubleshooting, and compliance.
4. Transparent Communication
Clearly communicate the capabilities and limitations of AI systems to users. Avoid overstating the system’s abilities or misrepresenting how it works.
5. Provide Explanation Interfaces
Design user interfaces that offer clear explanations for AI decisions, particularly in sectors like healthcare, finance, and criminal justice.
Organizational Practices for Responsible AI
To institutionalize fairness and transparency, organizations should adopt robust governance frameworks:
- AI Ethics Guidelines: Define principles and policies for responsible AI use.
- AI Review Boards: Establish multidisciplinary teams to review AI systems for fairness, bias, and interpretability.
- Training Programs: Educate developers, data scientists, and decision-makers about fairness and transparency.
- Continuous Monitoring: Regularly evaluate AI systems post-deployment to ensure fairness and transparency are maintained over time.
Legal and Regulatory Landscape
Governments and regulatory bodies around the world are introducing laws to enforce fairness and transparency in AI. Key developments include:
- EU AI Act: Mandates transparency and accountability for high-risk AI systems.
- GDPR (Europe): Requires “meaningful information about the logic” of automated decisions.
- Algorithmic Accountability Act (USA, proposed): Calls for impact assessments of automated decision-making systems.
Staying ahead of regulatory changes can help organizations avoid legal risks and build consumer confidence.
Conclusion
AI offers immense potential to enhance productivity, improve decision-making, and solve complex problems. However, without fairness and transparency, these systems risk harming the very individuals they are meant to serve. Ensuring fairness means actively preventing bias and discrimination. Transparency involves making AI understandable and accountable.
Achieving both requires a holistic approach—combining technical solutions, diverse data, inclusive design, stakeholder engagement, and ethical governance. By prioritizing these values, organizations can build AI systems that not only perform well but also uphold social trust and equity.
As AI continues to shape our world, fairness and transparency must be foundational principles—not afterthoughts—in its development and use.