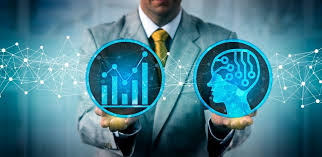
In the digital era, data is the new currency—and like any currency, its true value lies in how it’s used. The explosion of data from sensors, devices, transactions, and user behavior has created both a challenge and an opportunity for businesses. Organizations that can transform this data into meaningful insights gain a powerful competitive advantage. This is where analytics comes in, and more importantly, where artificial intelligence (AI) is redefining its capabilities.
The integration of AI into analytics is not merely a technological upgrade—it represents a profound shift in how decisions are made, problems are solved, and strategies are shaped. AI-powered analytics no longer just look at what happened. They predict what’s next, recommend actions, and in some cases, autonomously execute decisions. Over the past decade, we’ve witnessed a remarkable evolution from static reporting dashboards to dynamic, self-learning systems capable of delivering deep, real-time intelligence.
This blog explores the journey of AI-powered analytics, the transformation it brings to modern enterprises, the technologies fueling this shift, and how it will continue to shape business decisions in the years to come.
The Early Days: Descriptive Analytics
In the early stages of business intelligence, analytics was largely descriptive. Companies collected historical data and used it to create reports, charts, and summaries. These reports answered basic questions: What happened last quarter? How many units were sold? What were the revenues?
While useful for record-keeping and hindsight evaluations, descriptive analytics had limitations. It couldn’t explain why certain events occurred or suggest what actions to take next. The insights were reactive, and decisions often relied on intuition or further manual investigation.
Excel spreadsheets, structured SQL queries, and rigid dashboard tools dominated this era. Though helpful, they required significant effort, technical expertise, and time to yield results.
The Shift Toward Predictive and Prescriptive Analytics
As businesses began accumulating more data and computing power grew exponentially, analytics started evolving. Predictive models came into play, using statistical techniques and historical data to forecast future trends. This was the beginning of data science becoming a core business function.
Predictive analytics answered forward-looking questions: What will sales look like next month? Which customers are likely to churn? Which marketing campaigns are likely to succeed?
Soon after, prescriptive analytics entered the scene. These systems not only predicted outcomes but also suggested optimal courses of action. Should you launch the product in Market A or Market B? Which pricing strategy yields the highest profit?
This transition paved the way for more strategic, proactive decision-making, but it still relied heavily on human analysts and data scientists to develop models, clean data, and interpret results. The process was still time-consuming and prone to human bias.
Enter AI: The Analytics Game-Changer
The advent of AI brought a seismic shift in how analytics functions. Machine learning, a subset of AI, empowered computers to learn patterns from data without explicit programming. Instead of building models manually, machines could automatically identify correlations, outliers, and trends within massive datasets.
This evolution made analytics faster, smarter, and more scalable. AI could process unstructured data—like images, voice, or social media posts—alongside traditional structured data. It could continuously learn from new information, refine its predictions, and deliver insights in real-time.
Natural Language Processing (NLP) further democratized analytics. Users no longer needed technical skills to interact with data. They could ask questions in plain English—“What were last week’s top-selling products?”—and receive visual, data-driven answers instantly. AI made analytics more accessible, intuitive, and inclusive.
Key Components of AI-Powered Analytics
1. Machine Learning Models
AI systems use machine learning to detect hidden patterns, trends, and relationships in data. From classification algorithms that identify fraud to regression models predicting revenue, machine learning allows organizations to go beyond surface-level analysis.
These models continuously improve as they are fed new data, enabling businesses to adapt in real time to market dynamics, consumer behavior, and operational changes.
2. Natural Language Processing (NLP)
NLP allows users to interact with data conversationally, removing the need for specialized query languages. This innovation not only makes analytics more user-friendly but also enables voice-activated BI tools and smart search capabilities.
For example, a sales executive can simply ask, “Which region had the most returns last month?” and receive an immediate, visualized response.
3. Real-Time Data Processing
With IoT devices, social media feeds, and streaming platforms generating live data, traditional batch processing isn’t enough. AI-powered analytics platforms now leverage real-time analytics to provide immediate insights.
Retailers can adjust pricing dynamically based on inventory levels. Financial institutions can detect fraudulent transactions as they occur. Manufacturers can monitor equipment health and initiate maintenance before failures happen.
4. Automated Insights
Instead of waiting for an analyst to spot trends, AI systems automatically surface significant changes, anomalies, or opportunities. These systems alert users to critical metrics without needing to be prompted.
For instance, if sales drop unexpectedly in a specific region, AI can flag the issue, identify the cause—like competitor discounts or supply chain delays—and recommend countermeasures.
5. Augmented Decision-Making
AI doesn’t just present insights; it recommends actions. This is where prescriptive analytics merges with automation. Whether it’s suggesting which customers to target with a loyalty campaign or which inventory to restock, AI augments human decision-making with data-backed reasoning.
Industry Applications: Transforming Business Practices
Retail
AI-powered analytics helps retailers personalize customer experiences, optimize inventory, and forecast demand. Dynamic pricing models respond to real-time factors like competitor pricing, customer location, and buying behavior.
Customer churn models help retention teams intervene before customers leave, while sentiment analysis from reviews provides deeper insight into product reception.
Healthcare
Hospitals use AI to analyze patient data, predict disease outbreaks, and optimize resource allocation. Predictive models assist in early diagnoses, while AI-driven image analysis supports radiologists in identifying anomalies in scans.
AI also aids in managing patient flow, reducing wait times, and improving treatment outcomes by suggesting tailored care plans based on historical data.
Finance
Financial institutions use AI for fraud detection, credit scoring, and portfolio management. Real-time analytics tracks transaction behavior and flags suspicious activity.
Investment firms leverage AI to build smart portfolios, using real-time data to rebalance assets, assess risk, and recommend diversification strategies.
Manufacturing
Predictive maintenance models analyze sensor data from machinery to forecast failures before they occur. AI-driven supply chain optimization ensures that production aligns with demand, reducing waste and enhancing operational efficiency.
Quality control systems use image recognition to detect defects in real time on the assembly line, ensuring consistent product quality.
Challenges and Ethical Considerations
Despite its promise, AI-powered analytics is not without challenges.
Data Quality: AI models are only as good as the data they’re trained on. Inaccurate, incomplete, or biased data can lead to flawed insights.
Interpretability: Complex AI models, particularly deep learning networks, can be “black boxes,” making it hard to explain why a certain prediction was made. This lack of transparency is especially concerning in regulated industries like healthcare and finance.
Privacy and Security: AI systems often process sensitive information. Ensuring that data is anonymized, encrypted, and compliant with regulations (such as GDPR) is essential.
Over-Reliance on Automation: While AI enhances decision-making, complete reliance on automated insights can be risky. Human judgment and domain expertise remain crucial to validate findings and make ethical decisions.
The Future of AI-Powered Analytics
The future of analytics will be even more intelligent, contextual, and embedded into daily workflows.
Hyperautomation will integrate AI-powered analytics into every facet of business operations—from marketing and HR to logistics and customer service. Systems will proactively identify opportunities and automate responses with minimal human intervention.
Edge AI will bring analytics closer to data sources, enabling faster insights in industries like autonomous vehicles, remote healthcare, and smart infrastructure.
Explainable AI (XAI) will gain prominence, as businesses and regulators demand more transparency in AI decision-making. Users will not only get answers but also understand the logic behind them.
Personalized Analytics will tailor insights based on the user’s role, preferences, and goals, creating bespoke dashboards and recommendations that adapt in real-time.
Conclusion
The journey from basic dashboards to intelligent, self-learning analytical systems marks one of the most profound shifts in business technology. AI-powered analytics is more than a tool—it is a strategic enabler that empowers organizations to harness the full potential of their data.
As AI continues to evolve, its integration into analytics will deepen, creating a future where insights are instant, decisions are data-driven, and businesses are more agile than ever before.
The companies that embrace this evolution will not only thrive in today’s competitive landscape but also shape the intelligent enterprises of tomorrow.