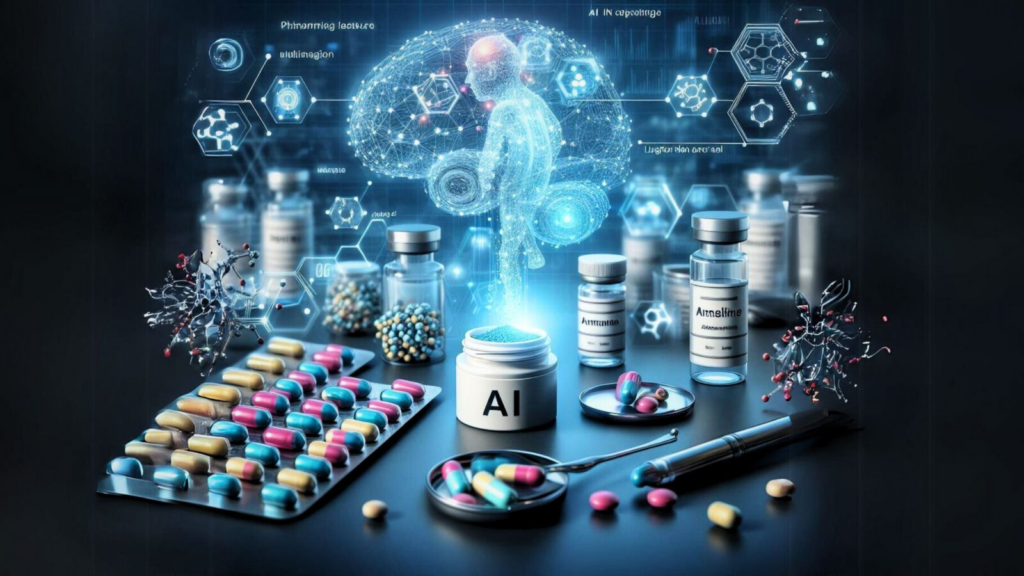
The pharmaceutical and biotechnology industries are among the most research-intensive sectors in the world, often requiring billions of dollars and over a decade to bring a single drug from discovery to market. In recent years, artificial intelligence (AI) has emerged as a transformative force in this domain—accelerating innovation, reducing costs, and unlocking new possibilities in drug development and personalized medicine.
AI is now being employed across nearly every stage of the pharmaceutical value chain, from molecule identification to post-market surveillance. As competition intensifies and the pressure to deliver effective treatments grows, companies are turning to AI not just as a tool, but as a strategic pillar of their R&D and operational ecosystems.
How AI is Transforming Pharma and Biotech
The integration of AI into pharmaceutical and biotech workflows enables researchers and organizations to process massive datasets, uncover complex patterns, and make data-driven decisions at a pace previously unachievable.
Below are the key areas where AI is making a significant impact:
1. Drug Discovery and Design
Traditional drug discovery is time-consuming and costly, involving trial-and-error experimentation on thousands of compounds. AI revolutionizes this process by using algorithms to predict how different molecules will interact with biological targets.
How AI helps:
- Screening millions of compounds in silico (via simulations) rather than in a lab
- Predicting drug-likeness, toxicity, and efficacy
- Identifying novel drug candidates based on existing databases and biological knowledge
Example: Companies like Insilico Medicine and Atomwise use AI platforms to design drug candidates in a fraction of the time required by traditional methods. In some cases, promising drug molecules have been generated within months instead of years.
2. Target Identification and Validation
Identifying the right biological target—such as a protein or gene linked to a disease—is critical in drug development. AI can analyze genomics, proteomics, and transcriptomics data to identify potential drug targets and validate their role in specific diseases.
Benefits:
- Increases likelihood of therapeutic success
- Reduces risk of failure in later development stages
- Helps understand disease mechanisms more deeply
AI tools can map out disease pathways and prioritize targets with the highest potential for intervention.
3. Clinical Trial Optimization
Clinical trials are essential but often inefficient, plagued by delays, high costs, and recruitment challenges. AI can improve various aspects of clinical trial design and execution:
Applications include:
- Patient stratification and recruitment based on genetic and demographic factors
- Adaptive trial designs using real-time data analysis
- Predictive modeling for patient dropout rates and adverse events
Example: IBM Watson has been used to match patients to clinical trials by parsing electronic health records and eligibility criteria, significantly speeding up the enrollment process.
4. Biomarker Discovery
Biomarkers are measurable indicators of disease state or treatment response. AI can sift through complex biological datasets to uncover potential biomarkers for early diagnosis, prognosis, and therapeutic response.
Impact:
- Enables development of companion diagnostics
- Supports precision medicine strategies
- Facilitates earlier and more accurate disease detection
Biotech companies are increasingly leveraging AI to build biomarker databases that support more targeted therapies.
5. Personalized and Precision Medicine
One-size-fits-all treatments often fail to address individual patient variations. AI can analyze patient-specific data—such as genomics, lifestyle, and environment—to recommend customized therapies.
How it works:
- Integrates multi-omic data (genomics, metabolomics, proteomics)
- Predicts individual treatment response and side effects
- Tailors drug dosages and combinations accordingly
Example: Foundation Medicine and Tempus use AI to analyze tumor profiles and guide personalized cancer treatments based on genetic mutations.
6. Drug Repurposing
Repurposing existing drugs for new indications is a cost-effective way to deliver treatments faster. AI can identify previously overlooked relationships between drugs and diseases by mining scientific literature, clinical data, and molecular databases.
Advantages:
- Shortens development time
- Reduces regulatory hurdles
- Enhances ROI on existing compounds
Example: During the COVID-19 pandemic, AI systems were employed to identify drugs like remdesivir and dexamethasone for potential treatment, accelerating the search for therapeutic options.
7. Manufacturing and Quality Control
AI-powered automation and monitoring tools improve the efficiency and consistency of pharmaceutical manufacturing processes.
Key benefits:
- Real-time process monitoring and control
- Early detection of deviations and defects
- Predictive maintenance of manufacturing equipment
AI enhances compliance with Good Manufacturing Practices (GMP) and ensures that drug quality remains consistent across batches.
8. Pharmacovigilance and Post-Market Surveillance
After a drug is launched, monitoring its safety and effectiveness is crucial. AI tools can scan adverse event reports, social media, and medical literature to identify emerging safety signals faster than traditional reporting systems.
Applications:
- Detecting rare side effects
- Analyzing unstructured text in health records and reports
- Automating signal detection workflows
This allows pharmaceutical companies and regulators to act quickly and prevent harm to patients.
The Role of AI in Biotechnology
In addition to drug development, AI is helping biotech firms innovate in areas such as gene editing, synthetic biology, and agricultural biotechnology.
Key uses include:
- Designing CRISPR guide RNAs for precise gene editing
- Optimizing cell and gene therapy protocols
- Simulating metabolic pathways to create engineered microbes
AI accelerates hypothesis generation and experimental design, making it a powerful tool for biotechnology startups and research organizations alike.
Benefits of AI in Pharma and Biotech
1. Reduced Time to Market
By automating and streamlining R&D processes, AI significantly shortens the drug development timeline.
2. Cost Savings
Fewer failed experiments and faster trials translate into substantial cost reductions over the course of development.
3. Higher Success Rates
AI improves decision-making in target selection, trial design, and patient selection, increasing the likelihood of successful outcomes.
4. Scalability
AI tools can handle vast datasets and workflows across multiple projects simultaneously, improving scalability.
5. Accelerated Innovation
AI drives faster hypothesis testing, iteration, and discovery, pushing the boundaries of what’s scientifically possible.
Challenges of Implementing AI in Pharma and Biotech
Despite its promise, the integration of AI into pharmaceutical and biotech companies is not without obstacles:
A. Data Quality and Availability
AI systems require large, high-quality datasets. Fragmented, inconsistent, or biased data can compromise model accuracy.
B. Regulatory and Ethical Hurdles
AI-driven decisions must be transparent, reproducible, and compliant with regulatory standards like those from the FDA or EMA.
C. Integration with Legacy Systems
Many firms rely on outdated IT infrastructures that hinder seamless AI adoption.
D. Talent Gap
There’s a growing need for professionals who understand both biomedical science and AI technologies.
E. Interpretability and Trust
Black-box AI models may not be acceptable in highly regulated environments where explainability is critical.
The Future of AI in Pharma and Biotech
As AI continues to evolve, its role in the pharmaceutical and biotech industries will become even more central. Future trends include:
- Explainable AI (XAI): Making AI models more interpretable and auditable for regulators and clinicians.
- Federated Learning: Enabling collaborative model training without sharing sensitive patient data.
- Quantum AI: Combining AI with quantum computing to solve complex biological problems at unprecedented speeds.
- AI + IoT in Bioprocessing: Real-time control of lab and manufacturing environments using sensor data and AI predictions.
Companies that invest now in responsible, scalable AI infrastructures will be better positioned to lead in the next era of biomedical innovation.
Conclusion
Artificial intelligence is redefining what’s possible in the pharmaceutical and biotechnology industries. By enhancing everything from drug discovery and clinical trials to personalized medicine and pharmacovigilance, AI is enabling smarter, faster, and more effective solutions to some of healthcare’s most pressing challenges.
As the industry continues to navigate complex regulatory landscapes, rising costs, and increasing demand for innovation, AI offers a powerful toolset for transforming ideas into therapies and improving patient outcomes.
To fully capitalize on AI’s potential, pharmaceutical and biotech companies must adopt a balanced approach—embracing innovation while upholding ethical standards, data integrity, and transparency. The future of medicine is intelligent, data-driven, and personalized—and AI is at the heart of it.