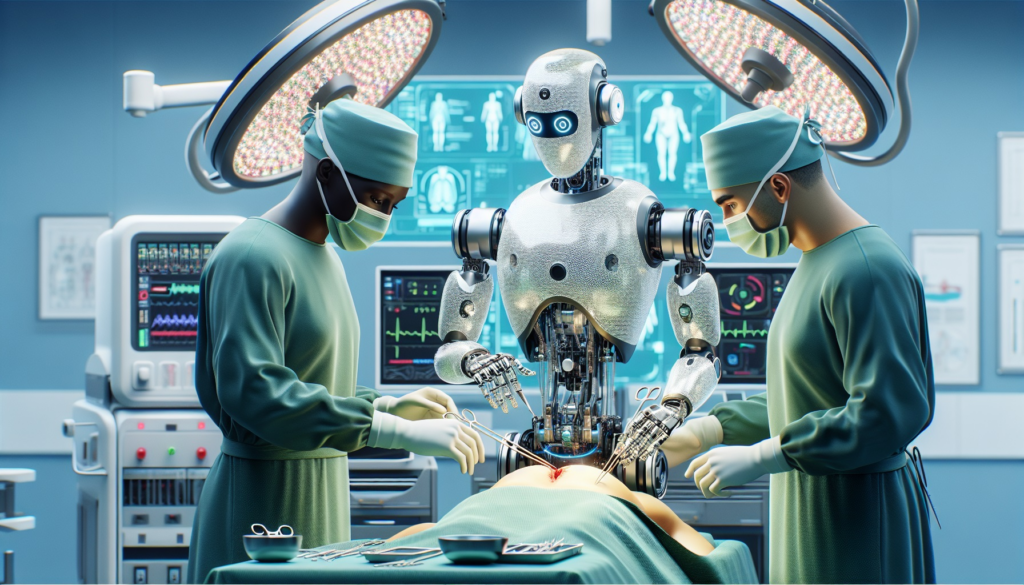
The healthcare industry is undergoing a significant transformation driven by the power of Artificial Intelligence (AI). As patient volumes grow, medical data proliferates, and demands for efficiency intensify, AI-powered tools are emerging as essential instruments to modernize healthcare delivery, improve patient outcomes, and reduce operational burdens.
From predictive diagnostics and robotic surgeries to AI-enabled administrative workflows and personalized treatments, artificial intelligence is no longer a futuristic concept in healthcare—it’s a reality reshaping how medical professionals operate and how patients receive care.
In this blog, we’ll explore how AI-powered tools are revolutionizing the healthcare sector, examine real-world use cases, discuss benefits and challenges, and offer strategies for successful adoption.
The Rise of AI in Healthcare
Healthcare generates massive amounts of data daily—from electronic health records (EHRs) and imaging scans to genetic profiles and wearable device outputs. Yet, much of this data remains underutilized. AI excels at analyzing large, complex datasets, identifying patterns, and making intelligent predictions, making it a perfect match for the needs of modern healthcare systems.
The global AI in healthcare market is expected to exceed $100 billion by 2030, driven by the increasing integration of machine learning (ML), natural language processing (NLP), computer vision, and robotics in medical processes.
Key Applications of AI in Healthcare
1. AI in Diagnostics and Imaging
AI algorithms are proving highly effective at detecting diseases from medical images such as X-rays, MRIs, and CT scans. These systems can identify patterns that may be difficult for human eyes to spot, aiding in early diagnosis and reducing diagnostic errors.
Use Case: Google Health’s AI model demonstrated high accuracy in detecting breast cancer from mammograms, sometimes outperforming radiologists. Similarly, AI tools like Aidoc and Zebra Medical Vision are being used in hospitals to analyze imaging data in real-time.
2. Predictive Analytics and Risk Assessment
AI models can analyze patient data to predict health risks and suggest preventive actions. This helps in managing chronic diseases and reducing hospital readmission rates.
Use Case: Predictive models can identify patients at high risk of developing diabetes or heart disease by analyzing lifestyle data, EHRs, and lab results. These insights help physicians intervene early with targeted care plans.
3. AI-Powered Virtual Assistants and Chatbots
AI chatbots and virtual health assistants can handle appointment scheduling, answer health queries, remind patients to take medications, and even offer preliminary medical advice, improving accessibility and patient engagement.
Use Case: Babylon Health’s AI chatbot offers symptom checking and medical triage, helping patients make informed decisions and reducing the burden on primary care providers.
4. Robotic Surgery and AI-Enhanced Procedures
Robotic surgical systems guided by AI provide improved precision, control, and flexibility during complex procedures. They can analyze real-time data and adjust surgical plans mid-operation.
Use Case: The da Vinci Surgical System, powered in part by AI, enables minimally invasive procedures with high precision, reducing recovery time and risk of complications.
5. Natural Language Processing for Clinical Documentation
NLP algorithms can process unstructured data such as physician notes and convert them into structured records, streamlining clinical documentation and enhancing decision support.
Use Case: Nuance’s Dragon Medical One uses AI to transcribe physician dictation accurately into EHRs, saving time and reducing documentation fatigue.
6. AI in Drug Discovery and Development
AI speeds up the drug discovery process by predicting how different compounds interact with biological targets, reducing the time and cost associated with bringing new drugs to market.
Use Case: DeepMind’s AlphaFold solved one of biology’s grand challenges—predicting protein structures—accelerating research in drug development and disease understanding.
7. Remote Monitoring and Wearable Integration
AI algorithms interpret data from wearables and remote monitoring devices to provide real-time health insights and alerts. This is especially useful for managing chronic conditions like hypertension or diabetes.
Use Case: Apple Watch and Fitbit devices use AI to monitor heart rhythms and alert users to irregularities like atrial fibrillation, potentially saving lives through early detection.
Benefits of AI in the Healthcare Sector
1. Improved Accuracy and Early Detection
AI systems can process vast amounts of data with greater speed and accuracy than humans, leading to earlier and more accurate diagnoses.
2. Operational Efficiency
By automating repetitive administrative tasks such as billing, claims processing, and scheduling, AI allows healthcare providers to focus more on patient care.
3. Personalized Medicine
AI enables tailored treatment plans based on an individual’s genetic makeup, lifestyle, and medical history, improving the effectiveness of interventions.
4. Enhanced Patient Engagement
AI chatbots and health assistants empower patients with information, reminders, and virtual consultations, improving adherence and satisfaction.
5. Cost Reduction
Early diagnosis, better resource utilization, and automation reduce healthcare costs for both providers and patients.
6. Accessibility to Care
AI tools can bridge the healthcare access gap by offering remote consultations, health education, and virtual diagnostics—especially beneficial in rural and underserved areas.
Challenges of AI Adoption in Healthcare
Despite its potential, AI adoption in healthcare comes with hurdles that must be addressed to ensure safe and ethical use.
A. Data Privacy and Security
Healthcare data is highly sensitive. AI systems must comply with data protection regulations such as HIPAA (in the U.S.) and GDPR (in the EU). Ensuring secure data storage and handling is crucial.
B. Bias and Fairness
AI models trained on biased datasets can result in discriminatory outcomes. For example, a diagnostic model trained primarily on data from one demographic may underperform for others.
C. Lack of Interpretability
Many AI models, especially deep learning systems, are often seen as “black boxes” with limited explainability, which can be problematic in clinical settings where trust and accountability are vital.
D. Integration with Existing Systems
Healthcare IT systems are often complex and fragmented. Integrating AI tools seamlessly with EHRs and other platforms can be technically challenging.
E. Regulatory and Ethical Concerns
AI systems used for diagnostics or treatment recommendations must undergo rigorous validation and regulatory approval. Additionally, ethical frameworks for AI use in healthcare are still evolving.
Best Practices for Implementing AI in Healthcare
1. Start with High-Impact Use Cases
Begin with applications where AI can demonstrate clear value, such as diagnostic imaging analysis or administrative task automation.
2. Ensure High-Quality, Diverse Data
Diverse and well-labeled datasets help in building robust AI models that generalize well across populations.
3. Involve Clinicians and Stakeholders
Collaborate closely with medical professionals to ensure AI tools are aligned with clinical needs and workflows.
4. Prioritize Transparency and Ethics
Use explainable AI models where possible, and implement strong governance practices to ensure ethical and equitable outcomes.
5. Invest in Training and Change Management
Educate healthcare staff about AI capabilities and limitations. Foster a culture of innovation and openness to change.
Future Trends: The Next Frontier of AI in Healthcare
The future of AI in healthcare holds exciting possibilities:
- Real-Time AI Diagnostics at the Point of Care: Tools that provide instant analysis during patient visits.
- AI-Driven Genomic Medicine: Precision treatments based on deep genetic analysis.
- Digital Twins for Patients: AI-generated virtual models of patients for personalized treatment simulation.
- Federated Learning: Collaborative model training without sharing sensitive patient data, enhancing privacy.
- AI-Augmented Mental Health Support: Tools that detect mood and mental health changes through speech or text analysis.
Conclusion
AI-powered tools are revolutionizing the healthcare sector by making care more predictive, personalized, and efficient. From diagnostics to drug discovery, AI is enhancing how medicine is practiced and experienced. However, to fully harness AI’s potential, healthcare organizations must overcome challenges related to data quality, integration, ethics, and trust.
By taking a strategic, patient-centric approach to AI implementation—starting with well-defined use cases, involving clinical stakeholders, and ensuring regulatory compliance—healthcare providers can unlock immense value and usher in a new era of intelligent, compassionate care.