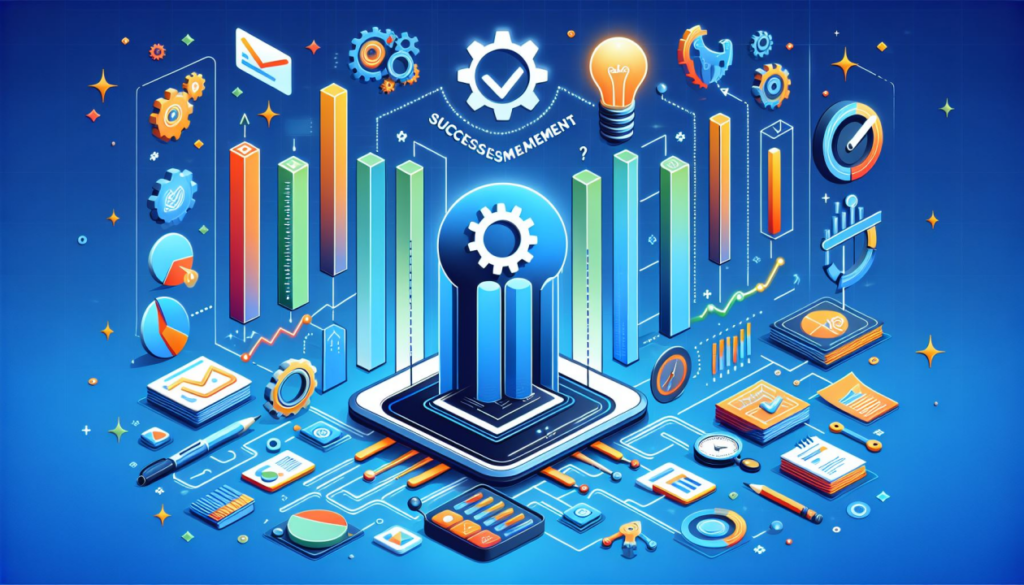
Integrating Artificial Intelligence (AI) into your organization’s workflows is a significant step toward digital transformation. However, simply launching an AI solution is not enough. To derive real business value and ensure sustainability, it’s essential to measure the success of your AI initiatives effectively.
Unlike traditional IT projects where outputs are often more predictable, AI deployments can yield varied results depending on data quality, model performance, user adoption, and business context. This makes defining and tracking success metrics a strategic necessity. This article will guide you through the key performance indicators (KPIs), evaluation methods, and best practices for assessing the impact of AI integration in your organization.
Why Measuring AI Success Is Different
AI systems learn, adapt, and often operate probabilistically. Traditional metrics such as uptime or error rate are still relevant but insufficient on their own. AI success must be assessed on both technical performance and business outcomes, making it more multidimensional.
Unique Challenges in Measuring AI:
- Outcomes may vary depending on input data.
- Accuracy may not always translate to business value.
- Deployment success requires user trust and adoption.
- Models may degrade over time due to data drift or changing environments.
A clear measurement framework helps stakeholders understand what’s working, what needs improvement, and whether AI investments are paying off.
Step 1: Define Success Before You Build
Success should be defined before the AI system is developed or deployed. Start by asking:
- What business problem are we solving?
- What does improvement look like?
- How will we know the AI solution is effective?
For example, if you’re using AI for customer support automation, your success might be a reduction in average handling time or an increase in customer satisfaction—not just a high model accuracy.
Step 2: Identify Key Performance Indicators (KPIs)
AI KPIs can be broadly categorized into two groups:
1. Technical Performance Metrics
These metrics measure the effectiveness of the AI models from a data science perspective:
- Accuracy: Percentage of correct predictions made by the model.
- Precision and Recall: Especially important in classification tasks, like fraud detection.
- F1 Score: Harmonic mean of precision and recall; a good all-around metric.
- Confusion Matrix: Useful for evaluating classification performance across classes.
- Latency: Time taken by the model to return predictions.
- Model Drift: A measure of how model performance changes over time.
2. Business Impact Metrics
These evaluate the real-world effectiveness of AI in delivering business value:
- Cost Reduction: Amount saved through process automation or operational efficiency.
- Revenue Growth: Additional revenue generated from better targeting, recommendations, etc.
- Time Savings: Reduction in hours spent on manual tasks.
- Customer Satisfaction (CSAT/NPS): Improvement in customer experience.
- Adoption Rate: Percentage of employees or customers using the AI system.
- Error Reduction: Drop in mistakes made in decision-making or process execution.
Step 3: Link AI Metrics to Business Goals
AI should never exist in isolation. Its success must align with broader business objectives. For instance:
- An AI-powered recommendation engine with 98% precision is impressive, but if it only increases sales by 1%, it may not be worth the investment.
- A model with slightly lower precision but integrated effectively into workflows may drive better business results due to ease of use or faster decision-making.
Tip:
Use a balanced scorecard approach that connects model performance with operational KPIs and strategic objectives.
Step 4: Monitor Short-Term vs. Long-Term Impact
AI initiatives often show value in phases. Track metrics in both the short and long term.
Short-Term Success Indicators:
- Successful integration into existing systems.
- Initial improvements in efficiency or prediction quality.
- Positive user feedback or adoption trends.
Long-Term Success Indicators:
- Sustained cost savings or revenue growth.
- Model robustness over time with minimal drift.
- Scalable benefits across departments or use cases.
Step 5: Gather Qualitative Feedback
Not everything that matters can be measured in numbers. Collect qualitative feedback from users, customers, and stakeholders to assess the usability and acceptance of the AI system.
Key feedback points:
- Is the AI easy to interact with?
- Do users trust its outputs?
- Are employees finding it helpful in decision-making?
- Has it improved customer interactions?
Surveys, interviews, and usability testing can offer deep insights that metrics alone may miss.
Step 6: Conduct A/B Testing and Benchmarking
Compare performance with and without the AI system to quantify its impact. This can be done through:
- A/B Testing: Randomly assign users to an AI-driven experience vs. a traditional one.
- Pilot Programs: Deploy AI in one business unit or region before a company-wide rollout.
- Historical Benchmarking: Compare metrics from before and after AI integration.
Be sure the tests are statistically significant and that external variables are accounted for.
Step 7: Set a Baseline and Measure Return on Investment (ROI)
To truly measure success, determine how much value the AI initiative has created compared to its cost.
Costs to Include:
- Development and deployment
- Data acquisition and storage
- Staff training and change management
- Ongoing maintenance and updates
Value Components:
- Cost savings through automation or efficiency
- Increased revenue from personalization or insights
- Time saved by employees
- Fewer errors or rework
ROI Formula:
textCopyEditROI (%) = [(Net Benefit from AI - Total Cost of AI) / Total Cost of AI] × 100
This financial measure helps gain executive buy-in and justifies future investments.
Step 8: Monitor Continuously and Iterate
AI systems are dynamic. Over time, user behavior, market trends, or regulations may change. What works today might not work tomorrow.
Continuous Monitoring Tips:
- Set automated alerts for key metrics (e.g., sudden drop in accuracy).
- Re-train models regularly to prevent performance decay.
- Revisit KPIs quarterly to reflect business evolution.
- Adjust models and integration strategies based on user feedback and performance data.
Use AI performance dashboards for real-time visibility and decision-making.
Step 9: Align with Compliance and Ethical Standards
Success isn’t only about performance and ROI. AI must also comply with ethical and legal standards.
Key compliance-related success factors:
- Are models free of bias?
- Is customer data handled ethically and lawfully?
- Can decisions be explained and justified?
- Are audit logs available for AI decisions?
A successful AI initiative is not just high-performing—it is fair, explainable, and trustworthy.
Step 10: Communicate Results to Stakeholders
Once you’ve measured success, share your findings with key stakeholders, including business leaders, IT teams, legal departments, and end-users.
Reporting Tips:
- Use non-technical language for business users.
- Highlight business impact more than technical details.
- Include visuals like graphs, trend lines, and comparison tables.
- Be transparent about challenges and areas of improvement.
Clear communication builds trust and sets the stage for scaling AI efforts.
Conclusion
Measuring the success of AI integration is critical to ensuring long-term value, guiding decision-making, and building confidence across the organization. It requires a thoughtful mix of technical rigor, business alignment, user feedback, and ethical awareness.
Rather than chasing high model accuracy or flashy results, focus on real-world impact, continuous improvement, and meaningful change. By establishing clear metrics and continuously refining your AI strategy, your organization can fully realize the transformative potential of AI—both now and into the future.