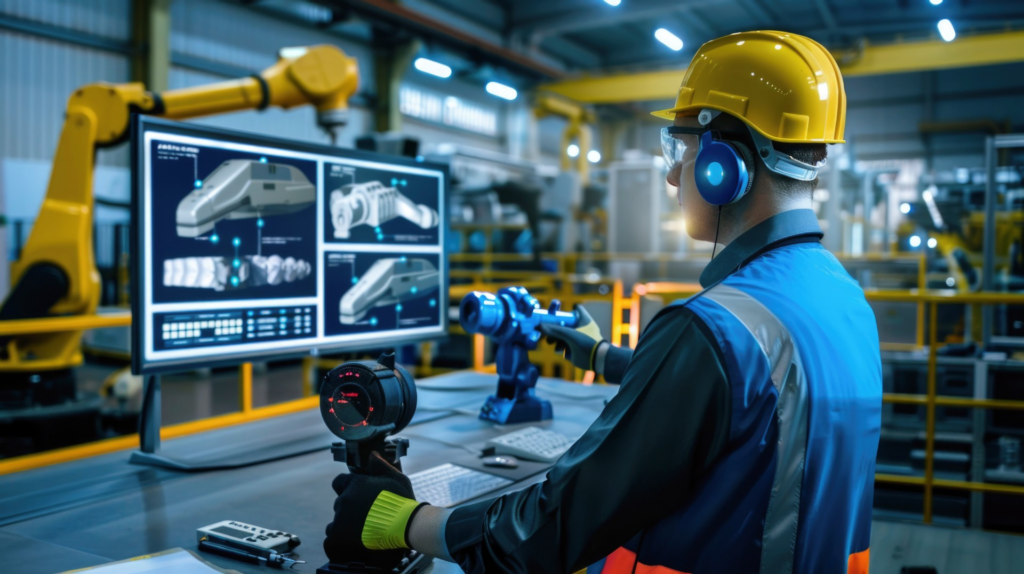
The manufacturing industry has always been driven by the need to increase efficiency, reduce costs, and ensure consistent product quality. Over the past few years, Artificial Intelligence (AI) has become a critical tool in helping manufacturers achieve these goals, particularly in the areas of predictive maintenance and quality control. By leveraging AI, manufacturers can predict machine failures before they happen, optimize production processes, and improve product quality—all of which contribute to a more streamlined and cost-effective operation.
In this blog, we will explore how AI is transforming the manufacturing sector, specifically focusing on predictive maintenance and quality control, and examine the benefits, challenges, and tools associated with these innovations.
1. Introduction: The Role of AI in Manufacturing
Manufacturing has long relied on traditional processes and machinery, but with the advent of Industry 4.0, the integration of smart technologies like AI is revolutionizing the sector. AI systems are capable of analyzing large volumes of data in real-time, identifying patterns, and making predictions—functions that were once reserved for highly trained human experts.
Predictive maintenance and quality control are two areas in manufacturing that benefit greatly from AI’s ability to analyze data and provide actionable insights. Predictive maintenance ensures that equipment operates smoothly without unexpected downtimes, while quality control ensures that products meet the required standards of performance and reliability.
2. AI in Predictive Maintenance
What is Predictive Maintenance?
Predictive maintenance (PdM) is a strategy that uses data-driven insights to predict when equipment or machinery will fail so that maintenance can be performed just in time to prevent breakdowns. Traditional maintenance schedules often follow a “run-to-failure” or “time-based” approach, which can lead to unnecessary downtime or excessive maintenance costs. Predictive maintenance, powered by AI and machine learning, moves beyond this reactive model and helps manufacturers identify potential issues before they cause disruptions.
AI models rely on data collected from sensors on machines, historical performance data, and external factors (e.g., temperature, humidity) to predict the likelihood of failure. The AI systems can detect anomalies in the performance of machinery, analyze trends, and predict when specific parts are likely to require maintenance.
How AI Powers Predictive Maintenance
- Data Collection: AI-powered predictive maintenance systems gather data from IoT sensors embedded in equipment and machinery. These sensors monitor variables such as vibration, temperature, pressure, and sound.
- Data Analysis: Machine learning algorithms analyze historical data, sensor inputs, and environmental factors to detect patterns that precede mechanical failures.
- Prediction: AI models forecast when equipment is likely to fail, helping businesses schedule maintenance activities ahead of time, avoiding unexpected breakdowns and reducing unnecessary maintenance costs.
Benefits of Predictive Maintenance
- Reduced Downtime: Predictive maintenance minimizes unplanned downtime by allowing companies to schedule maintenance before a breakdown occurs, thereby improving operational efficiency.
- Lower Maintenance Costs: By performing maintenance only when necessary, businesses can avoid over-maintenance and save on labor and parts.
- Extended Equipment Lifespan: Early identification of potential issues helps prevent severe damage, extending the life of critical machinery.
- Improved Safety: Predicting and addressing machine failures before they occur reduces the risk of accidents and injuries in the workplace.
AI Tools for Predictive Maintenance
- Uptake: A platform that uses AI to provide actionable insights into equipment health and maintenance schedules, improving operational efficiency.
- IBM Maximo: AI-powered asset management solution that uses predictive analytics to monitor equipment performance and predict failures.
- Plex: Cloud-based software that integrates predictive maintenance with manufacturing operations to enhance overall machine performance.
3. AI in Quality Control
What is AI-Driven Quality Control?
In manufacturing, quality control (QC) is the process of ensuring that products meet specific standards of quality before they reach the customer. Traditionally, QC involved manual inspection of products or sampling methods, which were both time-consuming and prone to human error. Today, AI plays a critical role in improving the accuracy, efficiency, and consistency of quality control by automating the inspection process and detecting defects in real-time.
AI-based quality control systems rely on computer vision, machine learning, and advanced analytics to analyze product characteristics, identify defects, and ensure that the production process adheres to quality standards.
How AI Powers Quality Control
- Computer Vision: AI-powered cameras and sensors capture high-resolution images of products as they move through production. Computer vision algorithms then analyze these images to detect defects, such as scratches, cracks, or missing components.
- Machine Learning: Machine learning models are trained on large datasets of defective and non-defective products. These models can learn to differentiate between acceptable and defective products, even identifying subtle defects that may be difficult for human inspectors to spot.
- Real-Time Analysis: AI systems process data in real-time, allowing manufacturers to identify defects and issues instantly. This allows for quick corrective actions, reducing waste and improving product quality.
- Predictive Analytics for Quality Trends: AI can identify trends in defects over time, enabling manufacturers to understand the root causes of quality issues. Predictive analytics also helps to anticipate potential future defects before they occur.
Benefits of AI in Quality Control
- Higher Accuracy: AI-driven quality control systems significantly reduce human errors in inspections, ensuring that products meet the highest standards.
- Increased Efficiency: AI automates the inspection process, reducing the time required for quality checks and speeding up production.
- Cost Reduction: By identifying defective products early, manufacturers can reduce waste, rework, and scrap costs.
- Consistent Quality: AI systems ensure consistent quality checks across production lines, reducing variability and maintaining uniform product quality.
- Better Product Traceability: AI-driven quality control systems can track defects back to their source, enabling manufacturers to identify and resolve the root cause of quality issues.
AI Tools for Quality Control
- Veo Robotics: Uses AI and computer vision to provide real-time quality inspection and defect detection on production lines.
- Landing AI: An AI platform focused on vision-based quality control, offering solutions for defect detection in manufacturing processes.
- Slyce: A visual AI platform that automates quality inspections, helping manufacturers identify defective products early in the production process.
4. Challenges of Implementing AI in Manufacturing
While AI offers numerous benefits for predictive maintenance and quality control, manufacturers must also be aware of the challenges involved in implementation:
- Data Quality and Availability: AI models require high-quality, structured data for training. Incomplete or inaccurate data can lead to unreliable predictions and incorrect quality assessments.
- Integration with Legacy Systems: Many manufacturing plants still rely on legacy systems that may not be compatible with modern AI tools. Integrating AI with older equipment and software can be a complex and costly process.
- Cost of Implementation: While AI promises long-term cost savings, the initial investment required to deploy AI technologies, including sensors, software, and infrastructure, can be high.
- Skilled Workforce: Implementing AI in manufacturing requires skilled data scientists, machine learning engineers, and operators who can manage and interpret AI-driven insights. There may be a need for additional training or hiring to ensure success.
- Privacy and Security Concerns: As manufacturing systems become more connected and data-driven, concerns about cybersecurity and data privacy become more pronounced. Ensuring the security of proprietary data and AI systems is critical.
5. The Future of AI in Manufacturing
The potential of AI in manufacturing is vast, and its impact is expected to grow in the coming years. As AI technologies continue to advance, we can expect even more sophisticated predictive maintenance and quality control systems that leverage new techniques such as deep learning, reinforcement learning, and edge computing. These advancements will further optimize manufacturing processes, reduce costs, and improve product quality.
In the future, AI-driven systems will also integrate with other Industry 4.0 technologies such as the Internet of Things (IoT), augmented reality (AR), and blockchain, creating a more connected and intelligent manufacturing ecosystem.
6. Conclusion
AI is significantly transforming the manufacturing industry, particularly in the areas of predictive maintenance and quality control. By leveraging AI to predict equipment failures and automate quality inspections, manufacturers can reduce downtime, lower maintenance costs, improve product quality, and increase overall operational efficiency. While the initial investment and integration may present challenges, the long-term benefits of AI adoption in manufacturing are undeniable. As AI technology continues to evolve, it will undoubtedly play an even more significant role in shaping the future of manufacturing.