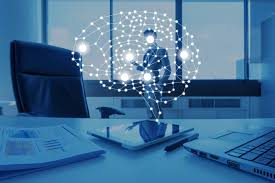
Artificial Intelligence (AI) is no longer a futuristic concept—it’s a practical, game-changing tool that’s already reshaping industries, transforming customer experiences, and enhancing decision-making. For business leaders, understanding AI is no longer optional. Regardless of your industry, having a solid grasp of what AI is and how it can be applied is essential for staying competitive.
This guide offers a beginner-friendly introduction to AI from a business leader’s perspective—what it is, how it works, its key types, practical use cases, and how to begin integrating AI into your organization.
What is Artificial Intelligence?
Artificial Intelligence refers to the ability of machines to perform tasks that typically require human intelligence. This includes learning from data, understanding natural language, recognizing patterns, making decisions, and even generating content.
AI can range from simple automation rules to complex machine learning algorithms that evolve over time. It’s not a single tool but an umbrella term that includes many technologies such as:
- Machine Learning (ML)
- Natural Language Processing (NLP)
- Computer Vision
- Generative AI
- Robotic Process Automation (RPA)
Why AI Matters to Business Leaders
AI is transforming how organizations operate. It enables companies to:
- Make faster, more accurate decisions using data
- Improve efficiency by automating repetitive tasks
- Enhance customer experience through personalization
- Identify trends and opportunities in real time
- Innovate new products and services
Companies that effectively adopt AI report higher productivity, better forecasting, improved customer satisfaction, and lower operational costs. Leaders who can harness AI are better positioned to drive transformation and long-term growth.
Key Types of AI Technologies in Business
1. Machine Learning (ML)
ML allows systems to learn from data and improve without being explicitly programmed. It’s used for:
- Predictive analytics (e.g., forecasting sales)
- Customer churn prediction
- Fraud detection
- Personalized product recommendations
2. Natural Language Processing (NLP)
NLP helps computers understand and respond to human language. Business uses include:
- Chatbots and virtual assistants
- Automated customer support
- Sentiment analysis of feedback
- Document summarization
3. Computer Vision
This involves analyzing and understanding images and videos. It’s useful for:
- Quality inspection in manufacturing
- Facial recognition for security
- Inventory tracking in retail
4. Robotic Process Automation (RPA)
RPA automates rule-based, repetitive tasks across software systems. Common uses include:
- Invoice processing
- Payroll management
- Data migration between systems
5. Generative AI
Generative AI models (like ChatGPT) can create human-like text, code, images, or designs. They are used for:
- Drafting emails or reports
- Generating marketing content
- Creating product designs or ideas
- Automating coding tasks
Common AI Use Cases Across Industries
AI isn’t confined to tech giants. Businesses across sectors are adopting AI to solve real-world problems:
Retail
- Personalizing shopping experiences
- Optimizing inventory management
- Chatbots for 24/7 customer support
Healthcare
- AI-assisted diagnostics
- Patient data analysis
- Virtual health assistants
Finance
- Fraud detection and prevention
- Algorithmic trading
- Risk modeling
Manufacturing
- Predictive maintenance
- Quality assurance with computer vision
- Supply chain optimization
Logistics
- Route optimization
- Delivery time predictions
- Warehouse automation
The AI Integration Roadmap for Business Leaders
Implementing AI can feel daunting, especially without a technical background. Here’s a simplified roadmap to guide you:
1. Identify Business Problems
Start by understanding your organization’s key challenges. Ask:
- What tasks are repetitive or rule-based?
- Where are decision-making delays hurting performance?
- Can customer experience be improved?
2. Assess Data Availability
AI needs data to work effectively. Check:
- Do you have quality, structured data?
- Are data collection and storage practices in place?
- Do you comply with data privacy regulations (like GDPR)?
3. Start Small and Focused
Choose one use case with a clear ROI. It’s better to succeed with a small pilot project than overextend too early. For example:
- Automate customer email classification
- Use ML for simple sales forecasting
- Implement an AI-powered chatbot on your website
4. Build the Right Team
Success with AI requires collaboration between business leaders and technical teams. You may need:
- Data scientists
- AI/ML engineers
- Domain experts
- IT support
Alternatively, you can work with external consultants or vendors.
5. Invest in Tools and Infrastructure
Consider whether you need:
- Cloud platforms (like AWS, Google Cloud, or Azure)
- Low-code AI platforms (e.g., DataRobot, Microsoft Power Platform)
- AI-as-a-service APIs (e.g., OpenAI, IBM Watson)
6. Measure and Iterate
Track metrics to evaluate the impact of your AI solution. Use KPIs like:
- Time saved
- Cost reduction
- Accuracy improvements
- Customer satisfaction
Use the feedback to improve and scale gradually.
Overcoming Common Challenges
AI implementation isn’t always smooth. Be ready to face and mitigate challenges such as:
– Lack of AI Talent
Hiring skilled AI professionals can be difficult. Upskill internal teams and consider partnerships.
– Poor Data Quality
AI is only as good as the data it’s trained on. Invest in data cleaning and governance.
– Change Resistance
Employees may fear automation. Ensure transparency, provide training, and communicate the goal is augmentation—not replacement.
– Ethical Concerns
Bias, privacy, and transparency are major concerns. Adopt ethical AI practices and ensure regulatory compliance.
Key Concepts Business Leaders Should Understand
You don’t need to become a data scientist, but knowing these concepts helps:
- Training Data: The examples used to teach the AI model.
- Model Accuracy: How well the AI performs the intended task.
- Bias: AI systems can reflect or amplify data biases.
- Explainability: Can the AI’s decision-making process be understood?
- Automation vs. Augmentation: AI should empower employees, not replace them.
The Future of AI in Business
AI is moving from experimental to essential. Emerging trends include:
- AI copilots for decision-making
- Autonomous business processes
- Multimodal AI (combining text, audio, images)
- AI-powered innovation and R&D
Organizations that embrace AI strategically and ethically will be best positioned to lead in the coming decade.
Final Thoughts
For business leaders, AI isn’t just a buzzword—it’s a strategic asset. By understanding the basics, identifying valuable use cases, and starting small, you can begin harnessing AI’s potential to drive innovation, efficiency, and growth.
Remember, the goal isn’t to turn every executive into a technologist. Instead, it’s to cultivate AI literacy—a mindset that allows you to recognize opportunities, make informed decisions, and lead with confidence in an AI-powered future.