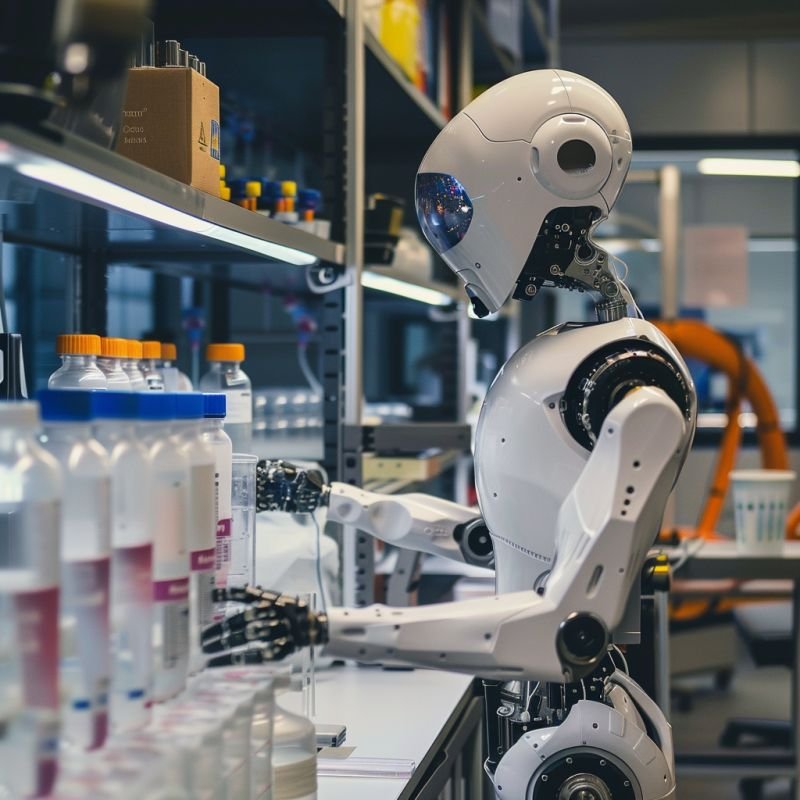
Scientific discovery has always been a human pursuit powered by logic, experimentation, and relentless curiosity. But in recent years, Generative AI has emerged as a powerful co-pilot in accelerating the pace of research and helping scientists break through previously insurmountable barriers.
From designing new molecules and materials to simulating complex systems and generating novel hypotheses, generative AI is transforming how science is done. In this article, we explore how generative AI is impacting scientific research, its key applications, benefits, limitations, and the future of discovery.
What is Generative AI?
Generative AI refers to algorithms that can create new data based on patterns learned from existing data. Unlike traditional AI systems that primarily classify or predict outcomes, generative models can:
- Generate new molecules
- Design new proteins
- Simulate environments or experiments
- Write research summaries or hypotheses
Key models powering generative AI include Generative Adversarial Networks (GANs), Variational Autoencoders (VAEs), and Transformer-based models like GPT and BERT.
The Shift in Scientific Research
Scientific discovery is no longer constrained by lab capacity or human trial-and-error. With AI’s ability to simulate outcomes, analyze massive datasets, and generate new ideas, researchers can:
- Reduce the time from hypothesis to testing
- Explore a vastly larger design space (e.g., millions of chemical compounds)
- Automate repetitive or tedious tasks
- Collaborate with AI as a creative partner
Applications of Generative AI in Scientific Research
1. Drug Discovery and Molecular Design
One of the most prominent use cases is in computational chemistry and pharmaceutical development.
- How it works: Generative AI learns from existing drug molecules and generates entirely new compounds that may bind to disease targets.
- Tools: DeepMind’s AlphaFold, Insilico Medicine, and Atomwise use generative models to predict protein structures, discover leads, and optimize drug candidates.
Example: In 2020, researchers used generative AI to propose potential inhibitors for SARS-CoV-2 (COVID-19) in just days—a process that traditionally takes months or years.
2. Materials Science
Generative models are used to design new materials with desired properties like conductivity, strength, or thermal resistance.
- AI learns from datasets of material compositions and can generate novel crystalline structures or polymers.
- Applications include batteries, semiconductors, and aerospace components.
Example: Researchers have used AI to propose new high-temperature superconductors with unique lattice structures.
3. Protein Folding and Structural Biology
DeepMind’s AlphaFold 2 has revolutionized biology by predicting 3D protein structures from amino acid sequences with near-laboratory accuracy.
- This opens doors to understanding diseases at the molecular level, designing targeted therapies, and exploring synthetic biology.
Generative AI is now being applied to generate entirely new proteins—ones not found in nature—designed to perform specific biological tasks.
4. Physics and Climate Modeling
Generative AI can simulate physical systems—fluid dynamics, quantum states, or climate models—without solving computationally expensive equations.
- GANs and VAEs can produce realistic simulations of weather, particle collisions, or cosmic structures.
- Helps researchers test hypotheses faster and with fewer resources.
Example: NVIDIA’s FourCastNet uses AI to predict global weather patterns much faster than traditional physics-based models.
5. Astronomy and Cosmology
In astronomy, generative AI can simulate galactic formations, classify galaxies, and even propose models of the universe’s evolution.
- AI-generated simulations allow scientists to test dark matter and dark energy theories by creating synthetic datasets for comparison.
Example: The CAMELS project uses AI to generate virtual universes, helping researchers explore fundamental physics.
6. Hypothesis Generation and Literature Review
Large Language Models (LLMs) like GPT-4 and BioMedLM can:
- Generate plausible hypotheses based on scientific literature
- Summarize long research papers
- Suggest potential experiment paths
Example: Semantic Scholar uses generative AI to recommend relevant papers and auto-generate concise summaries.
7. Genomics and Personalized Medicine
In genomics, generative AI helps design synthetic DNA sequences, simulate gene-editing outcomes, and predict protein-DNA interactions.
- AI-generated synthetic biology designs could lead to engineered organisms for drug synthesis or environmental remediation.
Startups like Synthego and Evonetix use AI to design and simulate genetic interventions.
8. Synthetic Data Generation
In many scientific domains, real data is scarce or expensive. Generative AI helps by:
- Creating synthetic but realistic datasets for model training
- Augmenting underrepresented categories
- Maintaining privacy in sensitive data (e.g., medical records)
Tools and Platforms for Scientific Generative AI
Tool/Platform | Application | Notes |
---|---|---|
AlphaFold 2 | Protein folding | Open-source by DeepMind |
ChemGAN | Molecule generation | GAN-based drug discovery |
BioGPT | Biomedical literature | NLP for biosciences |
FourCastNet | Weather prediction | Fast, accurate simulations |
Generative Chemistry Toolkit (GCT) | Molecular design | Used by pharma companies |
RosettaFold | Protein structure prediction | Alternative to AlphaFold |
IBM RXN for Chemistry | Reaction prediction | Automates retrosynthesis |
Benefits of Generative AI in Science
1. Accelerated Discovery
AI drastically shortens the cycle of hypothesis → experiment → result → refinement. What once took years can now be done in weeks.
2. Exploration of Vast Design Spaces
AI can search through millions of possibilities—molecules, materials, genes—far beyond what any human could explore manually.
3. Cost Reduction
Virtual simulations and AI-generated designs reduce the need for expensive lab testing, high-performance computing, or physical prototyping.
4. Enhanced Collaboration
AI provides tools that allow researchers from various disciplines to co-create, visualize, and test ideas together.
5. Democratization of Research
Open-source models and datasets empower smaller labs or institutions to access cutting-edge tools without requiring massive infrastructure.
The Future of Generative AI in Research
Looking ahead, generative AI will likely become a foundational part of scientific practice, just like lab notebooks and simulation software once did.
Emerging Trends:
- AI-first research labs that use autonomous agents for continuous experimentation
- AI-driven knowledge graphs that connect disparate findings across fields
- Digital twins of organisms for testing gene therapies or metabolic effects virtually
- Closed-loop science: AI suggests an experiment, runs it virtually, analyzes results, and refines the hypothesis automatically
As MIT Technology Review put it, “Generative AI may one day be the scientist’s lab assistant, literature reviewer, and co-inventor all in one.”
Generative AI holds immense promise for scientific discovery. It amplifies the creative and analytical capacities of researchers, speeds up innovation, and makes knowledge more accessible. But it’s not a replacement for scientific intuition, ethical scrutiny, or empirical rigor.
Rather, it’s a partner in the pursuit of truth—helping us explore the unknown with unprecedented speed and intelligence.
In the age of generative AI, the next great scientific breakthrough may come not just from the lab, but from the lab + code.