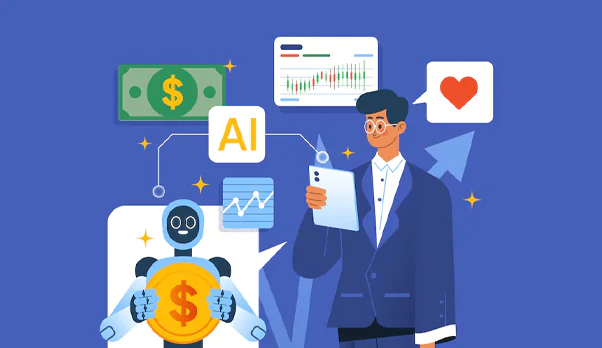
In the fast-paced world of finance, data is abundant, but deriving actionable insights remains a complex task. Traditional financial modeling relies heavily on historical data, deterministic equations, and human expertise. However, the integration of Artificial Intelligence (AI) is transforming this landscape—enabling more accurate forecasting, deeper risk assessments, and faster, automated financial decisions.
This blog explores how AI is revolutionizing financial modeling and forecasting, including use cases, technologies, benefits, challenges, and future prospects.
What Is Financial Modeling and Forecasting?
Financial Modeling
Financial modeling involves building abstract representations (models) of a real-world financial situation. These models help analyze performance, assess risks, or make strategic decisions. Common models include:
- Discounted Cash Flow (DCF)
- Budget forecasts
- Scenario analysis
- Valuation models
Financial Forecasting
Forecasting is the process of predicting future financial outcomes—like revenues, expenses, cash flow, or stock prices—using historical data and trends. Accurate forecasting is critical for budgeting, investment, and risk management.
The Role of AI in Finance
Artificial Intelligence—specifically Machine Learning (ML), Natural Language Processing (NLP), and Deep Learning—enhances traditional methods by learning from data patterns and continuously improving predictions. Rather than hard-coded formulas, AI models adapt dynamically to new data.
Example: While a traditional model might assume a linear revenue growth rate, an AI model can learn from seasonality, macroeconomic indicators, customer behavior, and news sentiment to predict fluctuations more accurately.
Key Applications of AI in Financial Modeling and Forecasting
1. Revenue and Sales Forecasting
AI can process vast amounts of historical sales data, market signals, promotions, and consumer behavior to predict future revenue more accurately than linear regression or moving averages.
- Retail: AI forecasts based on customer buying trends, competitor pricing, and weather data.
- B2B SaaS: Predicts churn, upsell potential, and recurring revenue.
2. Stock Price Prediction
Using deep learning, AI models can recognize complex patterns in historical price movements, volume, technical indicators, and even social sentiment.
AI Techniques Used in Financial ModelingTools Used: LSTM (Long Short-Term Memory) neural networks, Random Forest, XGBoost
Example: AI models analyze Elon Musk’s tweets and news headlines to predict Tesla stock volatility.
3. Credit Risk Assessment
Banks and lenders use AI to assess a borrower’s likelihood of default by analyzing:
- Financial history
- Transaction patterns
- Demographic data
- Behavioral signals (e.g., repayment consistency)
Example: Zest AI and Upstart use AI models that outperform traditional credit scoring systems like FICO.
4. Expense Forecasting and Budget Planning
AI helps CFOs and finance teams anticipate future expenses by analyzing vendor trends, inflation patterns, seasonality, and internal cost structures.
- Benefit: Allows for real-time scenario planning.
- Tool: Anodot and Planful for AI-driven financial planning and analysis (FP&A)
5. Market and Sentiment Analysis
Natural Language Processing (NLP) allows AI to digest news, analyst reports, and social media to evaluate market sentiment and assess risks in real time.
- Use Case: AI flags potential financial risks from geopolitical news or earnings call transcripts.
- Example: BloombergGPT or Thomson Reuters using NLP to assess earnings surprises.
6. Portfolio Optimization
AI enables dynamic, real-time portfolio management by continuously analyzing asset performance, economic indicators, and risk profiles.
Robo-advisors like Wealthfront and Betterment use AI to balance portfolios based on client goals and market shifts.
AI Techniques Used in Financial Modeling
AI Technique | Description | Financial Application |
---|---|---|
Regression (Linear, Logistic) | Predicts numeric or categorical outcomes | Revenue, credit scoring |
Time-Series Forecasting (ARIMA, LSTM) | Models sequential data with memory | Stock prices, cash flow |
Clustering (K-Means, DBSCAN) | Groups similar data points | Customer segmentation, fraud patterns |
Decision Trees / Random Forest | Ensemble methods for classification and prediction | Loan approvals, expense prediction |
Deep Learning | Neural networks for high-complexity tasks | Sentiment analysis, portfolio modeling |
NLP | Interprets human language from text | Market news analysis, earnings calls |
Popular Tools and Platforms
Tool/Platform | Use Case |
---|---|
DataRobot | AutoML platform for finance professionals |
Alteryx | Predictive analytics and data prep |
Microsoft Azure ML / Google Cloud Vertex AI | Scalable AI pipelines for enterprises |
Kensho (by S&P Global) | Market forecasting using NLP |
H2O.ai | Open-source ML for real-time analytics |
SAP Analytics Cloud / IBM Planning Analytics | Enterprise FP&A solutions |
Benefits of AI in Financial Forecasting
1. Increased Accuracy
AI models often outperform traditional methods due to their ability to identify nonlinear relationships and adapt to changing data patterns.
2. Real-Time Analysis
AI can analyze data streams in real time—enabling up-to-the-minute forecasting and anomaly detection.
3. Automation
Tasks like updating models, pulling data, running forecasts, and generating reports can be automated—saving time and reducing manual error.
4. Better Scenario Planning
AI models can simulate multiple “what-if” scenarios based on a range of input variables, helping companies prepare for different financial outcomes.
5. Insight Discovery
AI can unearth insights humans might miss—like subtle leading indicators or emerging patterns in unstructured data.
The Future of AI in Financial Modeling
As computing power increases and more data becomes accessible, AI’s role in finance will continue to grow:
- Self-learning models that improve over time without human input
- Explainable AI (XAI) to meet regulatory demands
- AI-Augmented Decision-Making where humans and AI collaborate in forecasting and planning
- Conversational AI for querying financial models using natural language (e.g., “What’s our cash flow projection for Q3 under a 15% cost increase?”)
AI won’t replace finance professionals—but those who understand how to leverage AI will replace those who don’t.
Generative and predictive AI are unlocking a new level of precision, speed, and flexibility in financial modeling and forecasting. From startups to Fortune 500s, organizations that embrace AI tools can outmaneuver competitors, reduce risk, and make smarter decisions backed by data-driven insights.
But success isn’t just about plugging in an AI model—it requires high-quality data, sound governance, and finance professionals who can interpret and act on AI-driven insights.
The future of finance is not just human or machine. It’s the human + AI collaboration that will drive smarter forecasting and better business outcomes.