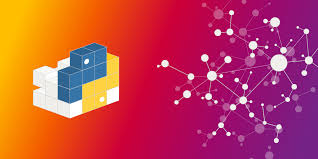
In the modern digital age, personalized recommendations have become a cornerstone of customer engagement. Whether you’re browsing an e-commerce website, watching videos on a streaming platform, or reading articles on a news site, personalized content recommendations aim to enhance your experience by tailoring suggestions based on your preferences. Traditionally, recommendation systems have relied on collaborative filtering and content-based filtering techniques. However, as the amount of data grows and the need for more accurate, meaningful recommendations increases, businesses are turning to more advanced technologies to improve personalization. One such technology is the knowledge graph.
Knowledge graphs have emerged as a powerful tool for enhancing personalized recommendations. By organizing and structuring data in a way that allows for more meaningful connections and inferences, knowledge graphs provide a more holistic understanding of users, products, and content. This approach enables businesses to create more contextually aware and insightful recommendations that cater to users’ specific needs.
In this blog, we will explore how knowledge graphs work, how they enhance personalized recommendations, and the benefits they bring to both businesses and customers.
What is a Knowledge Graph?
A knowledge graph is a data structure that represents relationships between entities in a graph format. Entities can be anything of interest, such as products, users, brands, locations, or topics. The key feature of a knowledge graph is its ability to capture not only the individual entities but also the relationships between them.
For example, in an e-commerce context, a knowledge graph could include nodes representing products, categories, brands, and user preferences. The relationships between these entities could indicate that a user likes a particular brand or has purchased items in a specific category. These relationships allow the system to reason about how different entities are connected, which can lead to more accurate and insightful recommendations.
Knowledge graphs often leverage ontologies, which are formal representations of knowledge within a specific domain. Ontologies help define the types of entities and the types of relationships between them, enabling the graph to capture more detailed and structured information.
How Knowledge Graphs Enhance Personalized Recommendations
Knowledge graphs enhance personalized recommendations in several ways by providing a deeper, more contextual understanding of users, products, and content. Here are some of the key benefits of using knowledge graphs for recommendation systems:
1. Rich Contextual Understanding
Traditional recommendation systems typically rely on surface-level data such as product categories or user behavior (clicks, purchases, etc.). While this data is useful, it doesn’t capture the full complexity of a user’s interests or the relationships between different items.
Knowledge graphs, on the other hand, provide a rich, interconnected view of entities and their relationships. For instance, a knowledge graph can understand that a user who likes action movies may also be interested in adventure movies, because the two genres share certain thematic elements. Similarly, it can recommend related products based on the relationships between brands, features, or usage contexts. This ability to understand context and relationships enables knowledge graphs to provide more sophisticated and personalized recommendations.
2. Handling Complex User Preferences
Users often have multifaceted preferences that may not be immediately obvious from their past actions. For example, a customer who frequently buys sports equipment might also enjoy fitness-related content, even though they’ve never explicitly interacted with it. Traditional recommendation systems might miss these nuances because they are limited to analyzing direct interactions.
With a knowledge graph, these more subtle preferences can be inferred by analyzing the connections between entities. If the graph identifies that a user follows fitness influencers and has purchased items related to both sports and health, it can recommend fitness-related content or products, even if the user hasn’t explicitly searched for them. This leads to a deeper level of personalization, helping businesses provide recommendations that better align with users’ hidden interests.
3. Improved Cross-Domain Recommendations
Another advantage of knowledge graphs is their ability to generate cross-domain recommendations. In many industries, users interact with multiple types of content or products across different domains. For example, a customer might shop for clothing on an e-commerce site, but also listen to music or watch movies. Knowledge graphs can seamlessly connect these diverse domains and provide holistic recommendations that span multiple areas of interest.
For instance, an e-commerce platform might use a knowledge graph to recommend a playlist based on the user’s clothing preferences, or suggest a movie related to the user’s interest in a particular product category. This type of cross-domain recommendation is difficult for traditional recommendation systems to achieve because they typically focus on one domain at a time.
4. Enhanced Content Discovery
One of the challenges in digital platforms is ensuring that users are exposed to relevant content that they might not have actively searched for. With the vast amount of content available on the internet, it’s easy for users to miss out on valuable recommendations simply because they don’t know to search for them.
A knowledge graph can help surface content that a user may not have discovered otherwise by identifying hidden connections between entities. For instance, a user who has bought a certain book may be introduced to related authors, topics, or even events. By analyzing the relationships between users, content, and other entities, the knowledge graph can proactively suggest content that the user is likely to enjoy, leading to greater content discovery.
5. Personalization at Scale
As businesses grow and accumulate larger user bases, delivering personalized recommendations at scale becomes increasingly challenging. Traditional systems may struggle to handle vast amounts of data and provide relevant recommendations to each individual user. Knowledge graphs, however, are inherently scalable and capable of processing complex relationships in real-time.
By continuously updating the relationships between entities and incorporating new data, knowledge graphs can adapt to changes in user behavior and preferences. This allows businesses to deliver personalized recommendations at scale while maintaining high levels of accuracy and relevance. Knowledge graphs can efficiently process large datasets, ensuring that users receive tailored content without compromising on performance.
Use Cases of Knowledge Graphs in Personalized Recommendations
Knowledge graphs are being implemented across various industries to improve personalization efforts. Let’s look at some real-world use cases:
1. E-Commerce
In e-commerce, knowledge graphs can power product recommendations, helping customers discover items they are most likely to purchase. By analyzing customer preferences, purchase history, and product relationships, a knowledge graph can suggest items that match a user’s style or needs. Additionally, it can predict which products a customer is most likely to buy next based on their interactions and connections between different product categories, brands, and features.
For example, if a customer frequently purchases eco-friendly products, a knowledge graph can recommend other environmentally conscious brands or related items, such as sustainable home goods or clothing. This level of personalization enhances the shopping experience and increases the likelihood of repeat purchases.
2. Media and Entertainment
Streaming platforms like Netflix and Spotify use knowledge graphs to personalize content recommendations. By analyzing the relationships between users, genres, actors, directors, songs, and more, knowledge graphs can suggest movies, TV shows, or music that align with a user’s taste. For example, a user who enjoys science fiction films might receive recommendations for other films or TV shows in the same genre, but also related content that shares similar themes or plot elements.
Knowledge graphs also help improve content discovery by identifying recommendations based on a broader range of factors, such as mood, time of day, or even seasonal preferences.
3. Healthcare
In healthcare, knowledge graphs can be used to provide personalized recommendations for treatments, medications, or wellness products. By understanding the relationships between patient data, medical conditions, treatments, and outcomes, healthcare providers can deliver more tailored care. For example, a patient with a specific condition may be recommended a personalized treatment plan based on the effectiveness of certain medications or therapies for similar patients.
4. Online News and Media
News outlets can use knowledge graphs to personalize content delivery based on a user’s reading history, preferences, and current events. By analyzing the relationships between topics, articles, and users, a knowledge graph can suggest articles that align with the user’s interests, even if they haven’t explicitly searched for them. This can enhance user engagement by providing a more dynamic and relevant news feed.
Conclusion
Knowledge graphs represent a significant leap forward in the way businesses approach personalization. By organizing and understanding the relationships between entities, businesses can offer more personalized, contextually aware, and relevant recommendations. Knowledge graphs enable richer user experiences by capturing hidden patterns, improving content discovery, and scaling personalized experiences for large user bases.
As businesses continue to collect more data, leveraging knowledge graphs to understand the complexities of customer preferences and behaviors will become increasingly important. By incorporating knowledge graphs into their recommendation systems, companies can provide more insightful and accurate suggestions, ultimately leading to higher customer satisfaction, engagement, and loyalty.