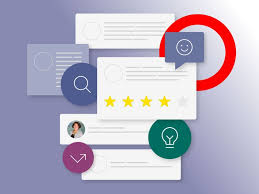
In a market where customers demand relevance and immediacy, personalization has evolved from being a nice-to-have to a core business strategy. While many organizations rely on behavioral data, purchase history, and user segmentation to shape personalization, one of the most underutilized sources of insight is direct customer feedback. Integrating this feedback into your personalization engine can dramatically elevate the quality and authenticity of user experiences.
Customer feedback is a goldmine of information. It reveals what customers truly think, feel, and expect. When combined with artificial intelligence and personalization systems, it unlocks the potential for dynamic and context-aware user experiences. This blog explores how to effectively integrate customer feedback into your personalization engine, the types of feedback to prioritize, and the tools and strategies that can guide this transformation.
Why Customer Feedback Matters in Personalization
While algorithms can infer preferences based on user actions, they often lack context. Feedback bridges that gap. It allows you to understand why users behave a certain way or feel dissatisfied.
Here’s why it matters:
- Feedback offers emotional context, often explaining dissatisfaction or delight.
- It helps validate personalization strategies by confirming whether users perceive recommendations as relevant.
- Feedback loops improve AI models by feeding in human evaluations that machines can learn from.
- It builds trust, as users feel heard when their input influences their experience.
Incorporating this into personalization not only makes it smarter but also more human.
Types of Customer Feedback to Integrate
Not all feedback is created equal. Different forms provide different insights, and each can serve distinct roles in personalizing user journeys.
1. Explicit Feedback
This includes ratings, reviews, survey responses, or direct comments. It’s valuable because it’s unambiguous and tied to specific actions or experiences.
Examples:
- A user rates a product 2 stars and explains the issue.
- A customer selects preferences in an onboarding form.
Use this type of feedback to directly influence content, recommendations, or communication preferences.
2. Implicit Feedback
This comes from user behavior, such as clicks, scrolls, time spent on a page, or abandoned carts. While not directly stated, this behavioral data indicates preferences or frustrations.
Examples:
- A user repeatedly skips certain types of content.
- An email campaign sees unusually low engagement from a segment.
This data can fine-tune personalization algorithms by identifying trends that may not be articulated explicitly.
3. Contextual Feedback
This includes responses captured during customer service chats, support tickets, or chatbot interactions. Often, users express needs or frustrations in real-time, providing actionable feedback.
Examples:
- “I couldn’t find the checkout button.”
- “The site doesn’t recommend what I usually buy.”
These inputs can improve personalization in navigation, UX design, or even predictive product recommendations.
4. Social Feedback
Mentions, reviews, or discussions on social media also reflect customer sentiment. These can offer valuable insights into how your personalization efforts are perceived beyond owned channels.
Building a Feedback-Informed Personalization Loop
To effectively integrate feedback into personalization, businesses need to build a feedback loop that includes collection, analysis, action, and refinement.
Step 1: Capture Feedback Seamlessly
Design multiple touchpoints where feedback can be naturally collected without disrupting the user experience.
Examples:
- Micro-surveys after key interactions (e.g., purchase, search).
- Feedback buttons on product pages or app features.
- Chatbots prompting for ratings or suggestions post-interaction.
Keep it short, relevant, and contextual to encourage higher response rates.
Step 2: Centralize and Organize Data
Feedback collected across platforms needs to be consolidated into a central system—often a Customer Data Platform (CDP) or a unified CRM.
Tag and categorize feedback based on:
- Source (web, app, email, support)
- Sentiment (positive, negative, neutral)
- Topic (product, UI, delivery, price)
This organization enables your AI models to access relevant inputs for decision-making.
Step 3: Analyze with AI and NLP
Manual analysis of feedback at scale is impractical. Use Natural Language Processing (NLP) tools to:
- Detect sentiment and tone
- Identify recurring themes
- Extract intent or suggestions
Modern NLP platforms can understand context, sarcasm, and even compare feedback trends over time. These insights serve as input features for your personalization engine.
Step 4: Feed Into the Personalization System
Once feedback is processed, the insights can be injected into various layers of your personalization engine.
Example implementations:
- Adjust recommendation weightings based on negative feedback.
- Suppress certain categories from being promoted to users who disliked them.
- Prioritize design fixes if multiple users raise navigation complaints.
Make sure the system can respond in real time or within a reasonable time window to deliver relevant changes.
Step 5: Close the Loop with the Customer
The final step is often overlooked—letting customers know their feedback was acted upon. This reinforces loyalty and encourages future engagement.
Examples:
- “We’ve updated your feed based on your preferences.”
- “Thanks for your feedback! We’ve improved our checkout process.”
Acknowledging feedback not only improves sentiment but also enhances trust in your brand.
Real-World Applications of Feedback-Driven Personalization
Case 1: E-commerce Customization
An online clothing retailer gathers post-purchase reviews where customers can mention fit, fabric, and style. These reviews are analyzed for sentiment and used to refine product suggestions.
Customers who disliked tight fits, for example, are later shown looser options in similar styles. As a result, return rates drop and repeat purchases increase.
Case 2: Content Streaming Personalization
A video streaming platform captures explicit feedback on watched content and analyzes skipped scenes or early exits. If viewers complain about too many horror recommendations, the system recalibrates its algorithms to favor different genres, improving watch time and satisfaction.
Case 3: SaaS Onboarding Optimization
A SaaS platform integrates chatbot feedback to understand pain points during onboarding. Many users mention confusion during the tutorial. Based on this feedback, the tutorial is personalized—novices get a guided walkthrough, while advanced users get shortcuts and tooltips.
User retention improves significantly as onboarding feels more tailored and efficient.
Tools and Technologies That Help
Here are tools that facilitate seamless integration of feedback into personalization workflows:
- Hotjar / FullStory – for collecting user behavior and contextual feedback.
- Typeform / SurveyMonkey – to create engaging surveys at key touchpoints.
- MonkeyLearn / AWS Comprehend / Google Cloud NLP – for feedback analysis using NLP.
- Segment / mParticle – to unify feedback with behavioral and transactional data.
- Salesforce / HubSpot / Zoho CRM – for managing customer profiles and acting on feedback-driven triggers.
Common Pitfalls and How to Avoid Them
Ignoring Feedback Volume
A single piece of negative feedback shouldn’t overhaul your personalization strategy. Look for patterns, not isolated incidents.
Over-Automation
While automation is critical, injecting some human oversight ensures your personalization remains empathetic and not mechanical.
Delayed Action
If feedback takes months to impact personalization, it’s too late. Strive for faster feedback-to-action loops, ideally within days.
Privacy Concerns
Always ensure user feedback is handled with respect to data privacy regulations (like GDPR) and transparency. Clearly inform users how their feedback will be used.
Conclusion
Integrating customer feedback into your personalization engine turns user insights into real-time, intelligent experiences. It complements data-driven inference with real-world context, making personalization not only more accurate but also more human.
When users see their input shaping the platform they interact with, they become more invested and loyal. By thoughtfully designing feedback loops, leveraging AI for analysis, and feeding insights back into your systems, you build a personalization engine that evolves with your audience—and earns their trust every step of the way.