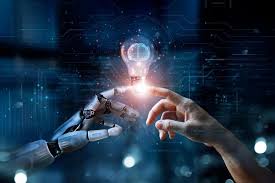
In the rapidly evolving digital age, customers expect more than just quality products—they demand seamless, relevant, and personalized experiences. Artificial Intelligence (AI) is emerging as a powerful enabler of these personalized services, revolutionizing how businesses interact with users, understand behaviors, and deliver tailored offerings in real time. If you’re just beginning to explore AI for personalization, this guide is your starting point.
Understanding AI-Powered Personalization
AI-powered personalization refers to the use of algorithms, machine learning, and data-driven strategies to customize content, products, services, or communication for individual users. Unlike traditional personalization techniques, which might rely on predefined rules, AI can learn from data patterns, user interactions, and behaviors to continuously adapt and optimize the experience.
Think of Netflix suggesting a movie, Amazon recommending products, or Spotify crafting daily playlists—all these services use AI to analyze user behavior and serve content that feels uniquely curated.
Why Personalized Services Matter
Modern consumers are overwhelmed with choices. What sets a brand apart is the ability to make customers feel understood and valued. Personalized services:
- Increase engagement by providing relevant content
- Improve customer satisfaction and loyalty
- Boost conversion rates and revenue
- Reduce churn by addressing individual needs
According to a McKinsey study, businesses that personalize effectively can generate 40% more revenue than those that don’t.
Laying the Groundwork: What You Need Before Starting
Before implementing AI, you need a strong foundation. Jumping into automation without clarity can lead to wasted time and poor outcomes. Here’s what to prepare:
1. Clear Objectives
Define what you hope to achieve with personalization. Are you trying to increase sales? Improve user retention? Enhance product recommendations? A clearly defined goal will shape your AI strategy and prevent scope creep.
2. Quality Data Collection
AI thrives on data. Begin collecting relevant user data from multiple touchpoints such as websites, apps, customer support chats, purchase history, and feedback forms. The richer and cleaner the data, the better the AI will perform.
Types of data you might gather:
- Demographics (age, location, device)
- Behavioral data (pages visited, time spent)
- Transactional data (previous purchases, cart history)
- Interaction data (emails opened, support queries)
3. Data Privacy and Compliance
Personalization should never come at the cost of user trust. Ensure your data handling is in line with GDPR, CCPA, or other relevant privacy regulations. Be transparent with users about how you collect and use their data, and provide opt-in/out options.
4. Integration Capabilities
Assess whether your existing systems (CRM, CMS, eCommerce platform, etc.) can integrate with AI tools or APIs. Seamless integration is key to implementing real-time personalization.
Step-by-Step: Implementing AI for Personalization
Once your foundation is in place, you’re ready to begin.
Step 1: Choose the Right Use Case
Start with a small, high-impact area. Don’t try to personalize everything at once. Pick one or two use cases where personalization can immediately improve user experience or drive ROI.
Popular use cases include:
- Personalized product or content recommendations
- Dynamic website experiences based on user behavior
- Tailored email marketing campaigns
- Intelligent chatbots for customer service
- Personalized pricing or offers
Step 2: Select Your AI Tools and Platforms
There are many AI-powered platforms designed for personalization, from all-in-one marketing tools to specialized recommendation engines. Some popular options include:
- Salesforce Einstein – AI for CRM and marketing
- Adobe Sensei – Personalized content and marketing
- Dynamic Yield – Experience optimization
- Optimizely – A/B testing with machine learning
- IBM Watson – General AI services with personalization capabilities
Choose a platform that fits your business size, budget, and technical expertise.
Step 3: Train Your AI with Relevant Data
Once your AI system is in place, it needs to learn. Feed it historical and real-time data, segment your users based on behaviors or attributes, and let the system start finding patterns. Depending on the complexity, this can take days or weeks.
Machine learning algorithms will look for trends, such as:
- What products are frequently bought together
- When certain users prefer to engage
- Which content types generate higher interactions
Over time, these patterns will shape personalized experiences that adapt with user behavior.
Step 4: Test, Measure, Refine
One of the biggest mistakes in AI adoption is assuming it will work perfectly right away. AI needs time to adjust, and businesses must continuously test and refine.
Use A/B testing to compare AI-driven personalization against control experiences. Monitor KPIs such as:
- Click-through rates
- Time on site
- Conversion rates
- Customer satisfaction scores
Use insights to further refine models, rules, and data inputs.
Common Challenges and How to Overcome Them
1. Data Silos
When data is stored in separate systems that don’t communicate, AI has a limited view. Solve this by creating a unified data warehouse or using integration platforms to connect disparate systems.
2. Lack of Technical Expertise
AI can be complex, but it’s not inaccessible. Use low-code/no-code AI tools or partner with vendors who provide implementation support. Upskill your team gradually as the system matures.
3. Over-Personalization
Too much personalization can feel intrusive or even creepy. Avoid referencing hyper-specific behavior that may make users uncomfortable. Focus on relevance without violating personal boundaries.
4. Inadequate Data
Without sufficient data, AI models won’t deliver accurate insights. Ensure you have enough users, sessions, or transactions before rolling out full personalization.
Future Trends in AI-Driven Personalization
AI and personalization are evolving together. Here are some exciting developments on the horizon:
Hyper-Personalization
Combines real-time data, predictive analytics, and user intent to offer next-level personalization, such as anticipating needs before users express them.
Conversational Personalization
AI chatbots are becoming more intelligent, adapting tone, language, and recommendations based on individual conversations.
Multimodal AI
Systems that integrate data from voice, image, text, and behavioral inputs to create a 360-degree view of the user.
Ethical AI
Growing focus on fairness, accountability, and transparency in personalization algorithms to avoid bias and maintain trust.
Real-World Success Stories
Amazon
Amazon’s recommendation engine drives 35% of its revenue. It uses AI to suggest products based on browsing, purchase history, and even what others are buying.
Netflix
The platform uses deep learning to recommend shows based on watch history, genres, and similar user preferences. It even customizes thumbnails for each user to increase engagement.
Sephora
Through its AI chatbot and mobile app, Sephora offers tailored product suggestions, virtual try-ons, and personalized skincare routines.
Final Thoughts
Implementing AI for personalized services is no longer a futuristic idea—it’s a competitive necessity. With thoughtful planning, quality data, and the right tools, any business can start delivering tailored experiences that delight users and drive results.
Begin with a single use case, learn from the data, and expand as you gain confidence. The journey to personalization is iterative, but every step you take brings you closer to deeper customer loyalty, better insights, and stronger business growth.