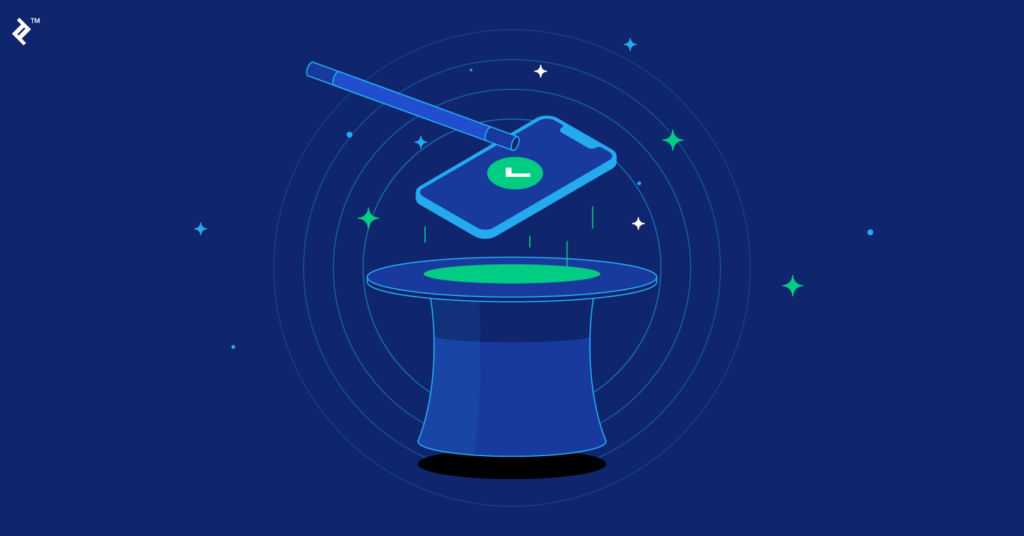
In the digital era, personalization has become the norm rather than the exception. Consumers expect tailored experiences, customized recommendations, and seamless interactions across platforms. While current personalization strategies are reactive—responding to user actions or preferences—anticipatory personalization is pushing the boundaries further by predicting needs before they arise.
Anticipatory personalization leverages advanced artificial intelligence (AI), machine learning (ML), predictive analytics, and behavioral data to offer proactive experiences. Rather than waiting for the user to make a move, the system acts as a digital assistant, forecasting needs and fulfilling them in advance. This shift toward proactive engagement is shaping the future of digital interactions in sectors like retail, healthcare, finance, education, and more.
In this blog, we will explore what anticipatory personalization is, how it works, its applications across industries, the underlying technologies, benefits, challenges, and the future outlook.
What is Anticipatory Personalization?
Anticipatory personalization refers to the use of predictive AI systems to forecast user needs, preferences, or actions before the user explicitly expresses them. This method relies on analyzing historical data, contextual signals, and behavioral patterns to deliver timely and relevant content or services.
Key Characteristics:
- Proactive rather than reactive
- Predictive rather than descriptive
- Context-aware and adaptive
- Deeply embedded in user journeys
Rather than responding to a search query, for instance, an anticipatory system might suggest content, services, or products just as a need begins to form—perhaps even before the user consciously realizes it.
How Anticipatory Personalization Works
The foundation of anticipatory personalization lies in collecting and analyzing a wide range of data:
1. Data Collection
Data is sourced from:
- Browsing history
- Purchase behavior
- Device usage patterns
- Location and time
- Calendar events
- Wearable and IoT sensors
- Social media interactions
2. Pattern Recognition and Machine Learning
Machine learning algorithms identify correlations between user behavior and outcomes. For example, if a user typically orders food around 7 PM on Fridays, the system can anticipate this and suggest meals or restaurants in advance.
3. Contextual Awareness
Combining real-time context—such as weather, location, and mood—with long-term preferences allows the system to adapt responses dynamically.
4. Action and Delivery
Personalized recommendations, alerts, content, or services are delivered proactively via email, app notifications, digital assistants, or smart interfaces.
Real-World Applications of Anticipatory Personalization
1. Retail and E-commerce
E-commerce platforms use anticipatory personalization to recommend products based on seasonal trends, cart abandonment patterns, and prior purchases. For example:
- Predicting when a customer will run out of household supplies and prompting a restock
- Suggesting accessories based on past purchases and upcoming weather changes
- Pre-loading product recommendations during peak hours to reduce friction
2. Healthcare and Wellness
Healthcare systems and wellness apps can forecast health events or user needs:
- Suggesting a hydration reminder based on activity and heat levels
- Alerting users to schedule checkups based on historical medical data
- Predicting potential illnesses using biometric data from wearables
3. Finance and Banking
Financial services use predictive models to:
- Alert users to unusual spending patterns
- Recommend saving or investment opportunities before account balances drop
- Suggest bill payments or transfers based on historical schedules
4. Travel and Hospitality
Travel companies can anticipate and cater to traveler needs:
- Suggesting hotel upgrades or amenities before arrival
- Recommending activities based on weather, travel history, and local events
- Offering real-time itinerary changes when delays or disruptions occur
5. Media and Entertainment
Streaming platforms use anticipatory personalization to:
- Autoplay content aligned with user mood and time of day
- Suggest new releases based on prior viewing sequences
- Create dynamic playlists anticipating energy levels and preferences
Technologies Powering Anticipatory Personalization
1. Artificial Intelligence and Machine Learning
These are the engines behind pattern recognition, user behavior analysis, and prediction algorithms.
2. Natural Language Processing (NLP)
NLP enables systems to understand and predict intent from user queries, messages, and voice inputs.
3. Sensor Integration (IoT/Wearables)
Smart devices and wearables provide real-time physical data—like steps, heart rate, location, and environment—that influence predictions.
4. Predictive Analytics
This involves using statistical techniques to forecast future behaviors, such as churn prediction or demand forecasting.
5. Cloud Computing and Big Data Infrastructure
Scalable infrastructure enables real-time processing and analysis of massive data sets required for personalization.
Benefits of Anticipatory Personalization
1. Enhanced User Experience
Proactive engagement reduces friction, improves satisfaction, and offers a sense of being understood without effort.
2. Increased Engagement and Loyalty
When systems anticipate and meet user needs, customers return more frequently and stay longer within the ecosystem.
3. Improved Conversion Rates
In retail, suggesting products before the user even searches can lead to quicker decisions and higher sales.
4. Optimized Operational Efficiency
Anticipating needs allows businesses to manage inventory, staffing, and resources more effectively.
5. Preventive Intervention
In healthcare or finance, predicting negative outcomes can prevent crises or unnecessary costs.
Challenges in Implementing Anticipatory Personalization
1. Privacy and Data Ethics
Anticipatory systems require deep access to user data. Without transparency and consent, users may feel their privacy is violated.
2. Over-Personalization
Being overly proactive can feel intrusive or annoying. Striking the right balance between helpful and invasive is critical.
3. Bias and Inaccuracy
AI models trained on biased data can lead to incorrect assumptions or unfair treatment of certain user groups.
4. Complexity and Cost
Developing and deploying predictive systems requires significant investment in infrastructure, talent, and time.
5. User Trust and Transparency
Users may be skeptical of systems making decisions for them. Clear communication about how and why recommendations are made is essential.
Best Practices for Deploying Anticipatory Personalization
- Start with Clear Objectives
Define what you want to predict and how it will improve user experience or business outcomes. - Ensure Transparency and Consent
Inform users about what data is being collected and how it’s being used. Offer opt-in and opt-out options. - Build Ethical AI Models
Regularly audit models for bias, fairness, and accuracy. - Prioritize Relevance Over Intrusion
Personalization should feel natural, not forced. Allow users to give feedback and control over suggestions. - Continuously Learn and Adapt
Feedback loops should inform the system and refine personalization over time.
Future Outlook
Anticipatory personalization is not just a feature—it’s becoming a strategic differentiator for forward-thinking organizations. In the near future, we can expect:
- Emotion-aware personalization using facial recognition or voice analysis
- Hyper-contextual AI that understands real-time scenarios in granular detail
- Unified personal ecosystems, where different apps and devices share data to offer seamless predictions
- Digital twins that simulate user behavior for pre-emptive problem-solving
With the rise of autonomous agents, anticipatory personalization will evolve into full-scale digital concierge experiences, making interactions effortless, intelligent, and increasingly human-like.
Conclusion
Anticipatory personalization is a powerful shift from reactive to proactive digital engagement. By forecasting needs before they arise, businesses can elevate user experience, enhance loyalty, and optimize outcomes across sectors. However, it also demands responsible use of data, ethical AI practices, and a strong focus on user trust.
As organizations continue to explore the frontier of personalization, those who invest early in anticipatory strategies will be best positioned to lead in the age of predictive digital intelligence.