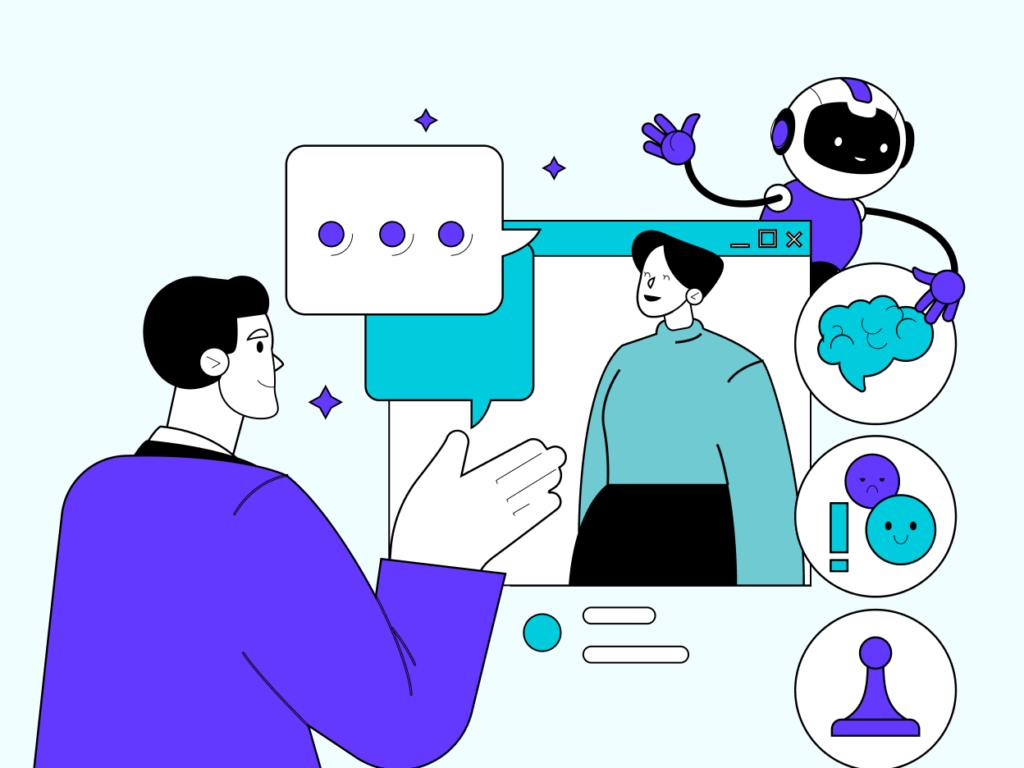
In the rapidly evolving landscape of artificial intelligence (AI), personalization has emerged as a key driver of user satisfaction, loyalty, and engagement. Whether it’s a product recommendation, a healthcare plan, or a learning path, the effectiveness of AI systems depends largely on how well they understand and respond to individual user needs. Enter multimodal AI—a powerful advancement that enables machines to process and interpret information from multiple sources such as text, images, audio, video, and sensor data simultaneously.
By integrating diverse types of data, multimodal AI allows for deeper context understanding, nuanced interpretation, and more accurate personalization. This blog explores what multimodal AI is, how it works, and how it’s transforming personalized experiences across various sectors.
What Is Multimodal AI?
Multimodal AI refers to artificial intelligence systems that can analyze and synthesize multiple modes or types of data. These may include:
- Text: Written language such as documents, messages, or emails
- Images: Visual inputs like photos, graphics, or scanned documents
- Audio: Spoken words, tone, pitch, or ambient noise
- Video: Movement, gestures, facial expressions, or scene context
- Sensor Data: From IoT devices such as fitness trackers or smart home systems
Unlike unimodal AI, which focuses on one data type, multimodal AI systems can combine these inputs to derive more comprehensive insights and create richer, more tailored experiences.
Why Multimodality Matters for Personalization
People naturally process information in a multimodal way. We don’t rely solely on what someone says—we also interpret facial expressions, tone of voice, gestures, and context. Similarly, effective personalization must account for multiple dimensions of user behavior.
Multimodal AI enables personalization that is:
- More contextual: It captures situational and emotional nuances.
- More accurate: It reduces ambiguity and error by cross-referencing multiple data points.
- More engaging: It delivers content in the most effective format based on user preferences and behavior.
By simulating how humans process information, multimodal AI brings a higher degree of empathy and relevance to digital interactions.
Core Technologies Behind Multimodal AI
Several technological advances underpin the development of multimodal AI:
1. Multimodal Transformers
Inspired by models like OpenAI’s GPT and Google’s BERT, multimodal transformers process different types of input data within a unified framework. Examples include CLIP (Contrastive Language–Image Pretraining) and Flamingo, which understand both visual and textual data.
2. Cross-Modal Learning
This technique allows models to learn relationships between modalities—for example, associating the word “cat” with an image of a cat, enabling better understanding and generation of content.
3. Attention Mechanisms
Attention layers in neural networks prioritize the most relevant parts of input data. In multimodal AI, attention mechanisms help the system decide which visual cue, word, or sound is most important at a given moment.
4. Fusion Techniques
Fusion involves combining features from multiple data sources. Early fusion integrates raw data, while late fusion merges outputs from separate unimodal models. Hybrid approaches combine the two for optimal performance.
Applications of Multimodal AI in Personalized Experiences
1. Healthcare: Personalized Diagnostics and Monitoring
Multimodal AI is transforming healthcare by combining patient data (EHRs), medical images (X-rays, MRIs), voice inputs (symptom descriptions), and wearable sensor data. This enables:
- More accurate diagnoses by cross-verifying symptoms and test results
- Customized treatment plans based on lifestyle and history
- Real-time health alerts through continuous monitoring
For instance, a diabetic patient wearing a glucose monitor and fitness tracker can receive personalized dietary suggestions based on activity, vitals, and historical data—something unimodal AI systems cannot deliver as effectively.
2. Retail: Richer E-Commerce Recommendations
E-commerce platforms increasingly use multimodal AI to tailor product recommendations. These models combine:
- Text (user reviews, search queries)
- Images (product photos)
- Behavior (browsing history, eye tracking)
- Audio (voice search inputs)
A multimodal system can suggest products based on what a user has seen, said, clicked, or heard. This results in higher conversion rates and improved user satisfaction.
3. Education: Adaptive Learning Paths
In edtech, multimodal AI enables personalized learning experiences by assessing:
- Written inputs (essays, quiz answers)
- Spoken language (oral responses)
- Visual cues (facial expressions, engagement levels via webcam)
- Interaction data (time spent on modules, mouse movement)
Based on this, the system can recommend different content formats, adjust difficulty levels, and offer targeted feedback—making learning more efficient and inclusive.
4. Entertainment: Tailored Content Curation
Streaming services are using multimodal AI to personalize content suggestions by analyzing:
- Viewing history
- Genre preferences
- Emotional response (via sentiment or biometric data)
- Social trends and user comments
For example, a platform might recommend an upbeat comedy if it detects that the user is stressed, based on wearable sensor data and recent search behavior.
5. Smart Assistants and Virtual Agents
Voice-based assistants like Siri, Alexa, or Google Assistant are evolving with multimodal capabilities. These systems now combine voice inputs, screen touch, facial recognition, and contextual data to provide smarter responses.
A smart assistant could, for instance, recognize a confused facial expression while explaining something, and automatically switch to a simpler explanation or offer a visual aid.
Benefits of Multimodal AI for Personalized Experiences
1. Higher Accuracy and Relevance
By analyzing multiple data types, multimodal AI reduces misunderstandings and improves the accuracy of predictions and recommendations.
2. Deeper Emotional Understanding
It can pick up on non-verbal cues like facial expressions or vocal tone to infer emotional states—making interactions more empathetic.
3. Improved Accessibility
Multimodal systems adapt to various user preferences or limitations (e.g., audio descriptions for visually impaired users), promoting inclusivity.
4. Context-Aware Responses
The ability to process situational context leads to smarter, more relevant outputs.
5. Cross-Platform Integration
Multimodal AI allows for seamless transitions between devices and formats, enhancing the continuity of user experiences.
Challenges in Multimodal AI Implementation
Despite its potential, multimodal AI faces several challenges:
1. Data Alignment and Integration
Synchronizing and integrating diverse data types (e.g., video and text) requires complex preprocessing and robust models.
2. Model Complexity
Multimodal models are often large and computationally intensive, demanding significant hardware and energy.
3. Privacy Concerns
Collecting audio, video, and biometric data raises ethical and legal concerns. Companies must ensure transparency and compliance with regulations like GDPR.
4. Bias and Fairness
If one modality is overrepresented or biased, it can skew results. Balancing and diversifying training data is crucial.
The Future of Multimodal AI in Personalization
The next wave of innovation in AI personalization will likely be driven by:
1. Multimodal Foundation Models
Like GPT or Gemini but multimodal from inception, these models will power a wide range of applications—from virtual tutors to AI therapists.
2. On-Device Multimodal AI
Edge computing will enable privacy-preserving, real-time personalization directly on user devices without cloud dependency.
3. Generative Multimodal Systems
These will not only interpret inputs but generate personalized content—like a customized workout video based on body metrics and user goals.
4. Emotion-Aware AI
Combining speech, facial expression, and physiological data will help machines respond to emotional states with empathy and precision.
How Businesses Can Get Started
Organizations aiming to leverage multimodal AI for personalization should consider:
- Identifying Valuable Use Cases: Start with customer touchpoints that can benefit from deeper personalization—like onboarding, support, or recommendations.
- Data Strategy: Ensure access to diverse, high-quality data across modalities while maintaining ethical standards.
- Technology Partnerships: Use cloud-based AI platforms (e.g., Azure Cognitive Services, Google Cloud AI, or Hugging Face) for faster prototyping.
- Pilot and Iterate: Begin with focused pilots, gather feedback, and scale gradually.
Conclusion
Multimodal AI represents a transformative leap in delivering rich, nuanced, and human-like personalized experiences. By harnessing the full spectrum of sensory and behavioral data, it empowers systems to understand users more deeply and serve them more effectively. As this technology becomes more accessible, organizations that embrace multimodal AI will be well-positioned to lead the next era of intelligent, individualized engagement.