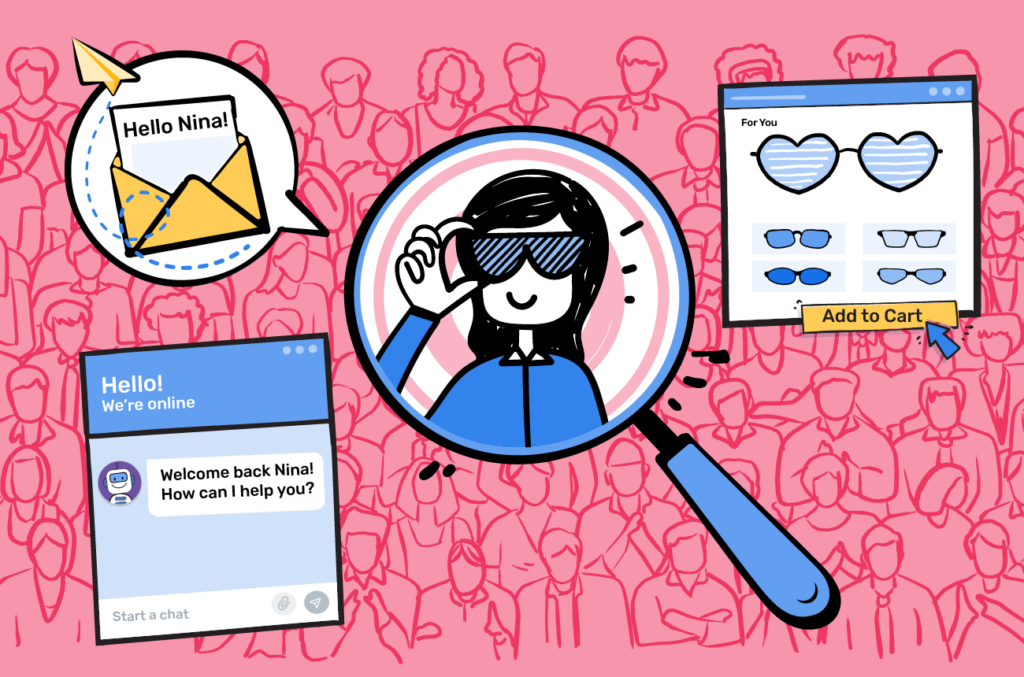
In today’s digital economy, personalization has become a defining feature of successful customer experiences. Whether it’s product recommendations, tailored news feeds, or customized learning paths, businesses rely on AI-driven personalization to engage users more effectively. However, the success of these systems doesn’t just depend on sophisticated algorithms or large volumes of data—it hinges significantly on user feedback.
User feedback serves as a critical tool in evaluating, refining, and improving AI personalization systems. In this blog, we’ll explore why feedback is essential, the types of feedback that exist, how to collect it responsibly, and how to use it to enhance personalization efforts.
Why User Feedback Matters in AI Personalization
AI personalization is fundamentally a prediction task—using past behavior and contextual signals to forecast what an individual will want or do next. But AI systems are not flawless. They may misinterpret signals, fail to adapt to changing preferences, or overlook subtle behavioral shifts.
User feedback closes this gap. It gives AI models a reality check, aligning machine predictions with actual user expectations and experiences. The importance of this alignment lies in:
- Improving Accuracy: Feedback helps correct incorrect assumptions or inferences.
- Increasing Trust: When users see their input being acted upon, it builds confidence in the platform.
- Personalization at Scale: Aggregated feedback helps refine recommendations for both individuals and broader audience segments.
- Continuous Learning: Feedback enables dynamic model tuning, allowing systems to evolve in real-time.
Types of User Feedback
Feedback can be broadly categorized into explicit and implicit forms.
1. Explicit Feedback
This is direct input from users regarding their preferences or satisfaction. Examples include:
- Ratings (e.g., 1 to 5 stars)
- Like/dislike buttons
- Surveys and polls
- Written reviews and comments
- Manual preference settings
Advantages:
- Clear and easy to interpret
- High confidence in the user’s intent
Challenges:
- Requires user effort
- Can be sparse, especially in systems with passive users
2. Implicit Feedback
This refers to behavioral signals that suggest user preferences. Examples include:
- Click-through rates
- Time spent on content
- Scrolling behavior
- Purchase history
- Navigation paths
Advantages:
- Passive and unobtrusive
- Large volume of data available
Challenges:
- Requires inference (e.g., time spent might indicate interest or confusion)
- Potential for misinterpretation
Both types are valuable and often used in combination to create more robust personalization models.
Techniques to Collect and Integrate Feedback
1. In-App Interactions
Prompting users for quick feedback (e.g., “Was this recommendation helpful?”) directly within the app ensures high contextual relevance. Keep it minimal to avoid disrupting the experience.
2. A/B Testing
Use A/B or multivariate testing to compare different versions of personalized experiences. Measure performance based on key feedback-driven metrics like click-through rates or session duration.
3. Surveys and NPS Scores
Net Promoter Scores (NPS) and short surveys provide insight into user satisfaction and overall experience. While not specific to each personalization element, they can indicate larger trends.
4. Feedback Loops
Incorporate mechanisms where users can actively correct personalization—for instance, removing items from a recommendation list or adjusting interest categories.
5. Review Analysis
Use natural language processing (NLP) to mine reviews, comments, and support tickets for sentiment and suggestions related to personalization quality.
Incorporating Feedback into Model Improvement
Once feedback is collected, it must be integrated into the AI pipeline to improve results. This happens at several stages:
1. Training and Retraining Models
Feedback data becomes part of the training set, helping models learn from real-world interactions. For instance, if users consistently ignore a category of recommendations, that signal can de-prioritize similar items in future outputs.
2. Feature Engineering
Feedback can create new features such as:
- Time-to-click after a recommendation
- Consistency of preference over time
- Repeated rejections of similar content
These features can greatly enhance model performance.
3. Personalization Rules
In hybrid systems that combine AI and rule-based logic, feedback can inform rules like:
- “Suppress recommendations from category X if rejected three times”
- “Show more diversity if engagement drops for three sessions”
4. Reinforcement Learning
In advanced systems, user feedback acts as a reward signal. Reinforcement learning models use this to continuously optimize actions based on reward maximization—where positive feedback increases the likelihood of similar future recommendations.
Case Studies: Feedback in Action
Netflix
Netflix is a prime example of feedback-driven personalization. The platform not only uses viewing history (implicit feedback) but also lets users give thumbs up/down (explicit). This dual feedback system enables Netflix to fine-tune recommendations, even adjusting artwork thumbnails based on what users respond to visually.
Amazon
Amazon leverages both purchase behavior and wishlists to understand user intent. They also let users mark irrelevant recommendations and report inaccurate content, allowing their AI systems to adjust accordingly.
Spotify
Spotify’s “Discover Weekly” and “Daily Mix” playlists use listening habits and skip rates to refine future recommendations. Feedback from millions of users is aggregated to spot emerging trends and recalibrate algorithm parameters.
Ethical and Privacy Considerations
Feedback collection—especially when it involves personal behavior—must be handled ethically and transparently.
1. User Consent
Clearly communicate how feedback data is used and obtain consent, particularly when collecting implicit signals or sensitive data.
2. Data Anonymization
Aggregate and anonymize feedback data wherever possible to protect user identities.
3. Opt-Out Options
Always give users the ability to disable personalization or feedback-based tracking.
4. Bias Awareness
Feedback loops can amplify biases (e.g., reinforcing popularity). Monitor models for unintended feedback-driven discrimination or content homogenization.
Best Practices for Feedback-Driven Personalization
- Make Feedback Easy to Give: Use one-click responses, visual cues, and unobtrusive prompts.
- Respond to Feedback Quickly: Reflect changes in the user interface or recommendations so users feel heard.
- Educate Users: Let them know how their feedback improves the experience.
- Segment Feedback: Analyze feedback across user segments (e.g., new vs. returning users) to gain nuanced insights.
- Measure Feedback Quality: Track metrics like feedback response rate, satisfaction improvement, and reduced churn.
Future of Feedback in AI Personalization
With advances in technology, feedback collection and utilization are becoming more sophisticated. Emerging trends include:
- Conversational Interfaces: Chatbots and voice assistants collecting real-time, contextual feedback during interactions.
- Emotion Detection: AI analyzing facial expressions or voice tone (with consent) to interpret satisfaction.
- Federated Learning: Personalized models trained on decentralized feedback data to enhance privacy.
As AI systems become more ubiquitous, user feedback will play an increasingly central role—not just in fine-tuning outputs, but in shaping ethical, human-centered AI experiences.
Conclusion
User feedback is not a mere add-on to AI personalization—it is the lifeblood of its continuous improvement. By actively collecting, analyzing, and integrating feedback, businesses can build systems that not only predict user needs but also learn and grow alongside them.
In the era of data-driven engagement, those who listen closely to their users and reflect their voices in their personalization strategies will stand out—not just for their innovation, but for their empathy, responsiveness, and user-centric approach.