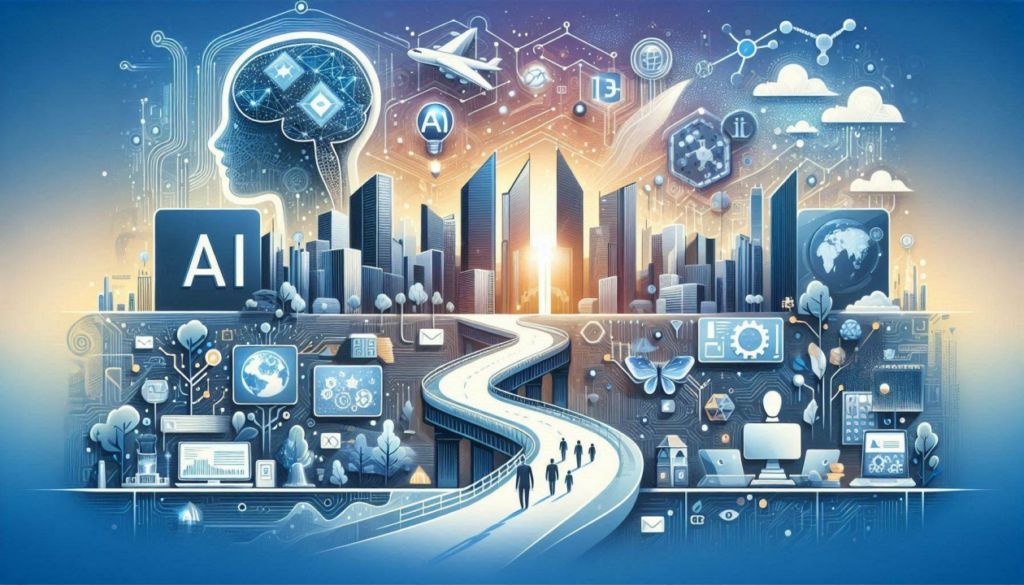
As artificial intelligence (AI) personalization becomes central to customer engagement strategies across industries, the importance of robust data management and governance cannot be overstated. AI systems thrive on data—the more accurate, timely, and relevant the data, the more powerful and precise the personalization outcomes. However, with great data comes great responsibility. Mismanaged or poorly governed data can lead to privacy breaches, compliance violations, biased outputs, and loss of customer trust.
This blog explores how businesses can establish effective data management and governance frameworks to support AI personalization, ensuring both ethical standards and business performance are upheld.
Understanding AI Personalization and Its Data Dependence
AI personalization involves tailoring content, services, recommendations, or interactions to individual users based on their preferences, behavior, context, and past activity. To enable this, AI models require access to large volumes of data, including:
- Demographics
- Purchase history
- Browsing patterns
- Location data
- Interaction logs
- Social media activity
The complexity and sensitivity of this data demand a rigorous approach to how it is collected, stored, processed, and used. Without structured data management and governance, the risk of inefficiencies, inaccuracies, and ethical missteps increases significantly.
What Is Data Management in AI Personalization?
Data management refers to the practices, policies, and tools used to collect, organize, store, and maintain data used in AI systems. For AI personalization, effective data management ensures that:
- Data is accessible and interoperable across systems
- Information is clean, accurate, and consistent
- Updates and new inputs are tracked and versioned
- Data pipelines are scalable and secure
Without these fundamentals, even the most sophisticated AI algorithms will produce flawed or untrustworthy results.
What Is Data Governance?
Data governance is the overarching strategy and structure that defines how data is handled within an organization. It includes:
- Policies on data usage, privacy, and security
- Roles and responsibilities for data access and control
- Compliance with regulations like GDPR, CCPA, HIPAA
- Ethical standards for data handling and AI deployment
Data governance ensures that data-driven personalization aligns with legal, ethical, and organizational standards.
Why Data Management and Governance Are Crucial for AI Personalization
1. Ensuring Data Quality
AI personalization depends on high-quality data to function accurately. Incomplete, duplicate, or inconsistent data can lead to:
- Irrelevant recommendations
- Misidentification of customers
- Poor customer experience
- Mistrust in personalization features
Governance frameworks enforce validation and verification rules to ensure only reliable data is used.
2. Maintaining Customer Trust
With rising consumer awareness about data usage, transparency in how personal data is collected and applied is key. Data governance enforces:
- Consent-based data collection
- Transparent data processing policies
- Options for data access, correction, or deletion
Trust is essential for sustained user engagement and long-term personalization efforts.
3. Regulatory Compliance
Personalization activities often involve handling sensitive personal data. Non-compliance with privacy laws can lead to penalties, lawsuits, and reputational damage. Governance ensures:
- Auditable data trails
- Clear data retention policies
- Proper anonymization or pseudonymization methods
4. Bias and Fairness Management
AI systems can inadvertently reinforce biases if trained on skewed data. Strong data governance practices help:
- Monitor training data for representational balance
- Document data sources and usage
- Review algorithm outputs for discriminatory patterns
This ensures personalization is inclusive and equitable.
Key Components of a Data Management Strategy for AI Personalization
To support AI personalization, your data management system should include the following components:
1. Data Inventory and Cataloging
Create a comprehensive inventory of all data sources relevant to personalization. This includes structured and unstructured data from CRM systems, websites, mobile apps, customer service logs, and social platforms.
Action Step: Use metadata tagging to enhance discoverability and lineage tracking.
2. Data Integration
Consolidate data from disparate systems to create a unified customer view. Integration ensures the AI engine can access comprehensive, cross-channel insights.
Action Step: Implement APIs, data lakes, or customer data platforms (CDPs) to facilitate integration.
3. Data Quality Controls
Establish procedures for deduplication, normalization, error correction, and consistency enforcement.
Action Step: Use automated tools for real-time data cleansing and validation.
4. Scalability and Storage Management
As personalization scales, so does the data. Adopt cloud-based or distributed storage solutions to manage large volumes of data effectively.
Action Step: Ensure your infrastructure can handle spikes in data ingestion and query loads.
Building a Strong Data Governance Framework
Data governance structures must be designed to align with both business needs and regulatory obligations. Key components include:
1. Data Ownership and Stewardship
Assign clear ownership of data assets. Data stewards are responsible for ensuring the integrity and accessibility of data within their domain.
Tip: Define roles like data owners, custodians, analysts, and auditors.
2. Data Usage Policies
Create explicit policies that define:
- Who can access what data
- How data can be used
- Rules for sharing and transferring data
- Procedures for data disposal
These policies must be communicated and enforced across the organization.
3. Consent Management
Implement systems to capture, manage, and update user consent for data collection and processing.
Tool Suggestion: Consent management platforms (CMPs) can automate this process and ensure compliance.
4. Auditability and Monitoring
Regular audits help verify that data practices align with policies and regulations. Monitoring tools should be in place to detect anomalies or unauthorized access.
Best Practice: Maintain logs for all data interactions and model decision outputs.
Tools and Technologies for Data Management and Governance
To streamline implementation, consider using:
- Customer Data Platforms (CDPs): Segment, Tealium, BlueConic
- Data Governance Tools: Collibra, Alation, Informatica
- Data Quality Platforms: Talend, Trifacta, Ataccama
- Compliance Tools: OneTrust, TrustArc
- AI Auditing Tools: Fiddler AI, Arthur AI
These tools offer automation, visualization, and AI-driven insights for smarter data control.
Challenges and How to Overcome Them
1. Legacy Systems
Older systems may not support modern integration or data governance protocols.
Solution: Use middleware or APIs to bridge gaps or prioritize modernization initiatives.
2. Data Silos
Organizational departments may hoard data or lack incentives to share.
Solution: Promote a data-driven culture and enforce governance mandates across teams.
3. Volume and Velocity of Data
Real-time personalization requires rapid data processing and response.
Solution: Invest in scalable infrastructure like cloud-native platforms and in-memory data processing.
4. Cultural Resistance
Teams may resist changes to data access or privacy controls.
Solution: Provide training, showcase ROI of personalization, and align governance with business objectives.
Real-World Example: AI Personalization in Retail
A retail brand uses AI to personalize product recommendations, marketing emails, and in-store experiences. Here’s how they implement data governance:
- Collect consent through a unified login and preferences center
- Centralize data in a CDP linked to all touchpoints
- Apply real-time data cleansing before feeding into AI models
- Use AI audit tools to monitor fairness in recommendations
- Maintain compliance with GDPR and CCPA through automated reports and deletion workflows
The result: a significant increase in click-through and conversion rates, alongside enhanced customer trust.
Conclusion
AI personalization offers enormous potential to deliver better experiences, drive customer engagement, and increase revenue. But without robust data management and governance, these benefits can be short-lived or come at a cost. By investing in structured, ethical, and compliant data practices, organizations lay the foundation for AI personalization that is not only effective but also responsible and sustainable.
Data is the fuel for personalization—and how you manage and govern that fuel determines whether you ignite innovation or risk a fire.