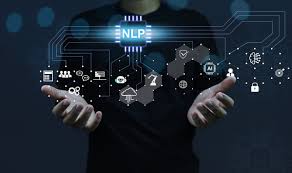
The digital age has shifted the way we communicate, learn, shop, and entertain ourselves. In today’s interconnected world, individuals expect digital systems to recognize their needs and respond in real time. The days of static, one-size-fits-all platforms are fading. Taking their place are intelligent systems powered by advanced technologies, among which Natural Language Processing (NLP) plays a crucial role.
NLP, a branch of artificial intelligence (AI), focuses on enabling machines to understand, interpret, generate, and respond to human language. With the help of NLP, brands can create deeper, more human-like interactions with their users. Whether it’s through chatbots, personalized product suggestions, automated support systems, or voice assistants, NLP is revolutionizing how humans and machines connect.
This article explores how NLP is being used to foster personalized experiences, boost user satisfaction, and help businesses adapt to the unique demands of every individual.
What Is Natural Language Processing?
Natural Language Processing bridges the gap between human communication and computer understanding. At its core, NLP enables machines to interpret text or spoken words in a way that captures meaning, emotion, and context.
Key components of NLP include:
- Tokenization: Breaking sentences into individual words or terms.
- Part-of-speech tagging: Identifying nouns, verbs, adjectives, etc.
- Named Entity Recognition (NER): Detecting names, organizations, locations.
- Sentiment analysis: Understanding emotions (positive, negative, neutral).
- Semantic analysis: Capturing the meaning and relationships between words.
- Text summarization: Condensing long passages into concise summaries.
- Language generation: Creating human-like responses or suggestions.
While these processes seem simple, they rely on intricate algorithms, machine learning models, and extensive training data to understand the nuances of human speech and writing.
The Rise of Personalization in the Digital Era
Modern users are constantly interacting with applications, websites, and digital services. With every click, search, or message, they generate data that reflects their preferences, behaviors, and intentions.
Personalization has evolved from merely inserting a customer’s name into an email. Today, it involves deeply understanding an individual’s needs and tailoring experiences accordingly — in real time. This is where NLP becomes indispensable.
By analyzing natural language inputs like typed queries, reviews, or spoken commands, NLP can determine what the user wants, how they feel, and what content or solution is most relevant to them.
Personalized Interactions Powered by NLP
NLP has penetrated multiple industries and applications to provide personalized engagement. Below are key use cases where NLP is transforming customer experience and delivering tailor-made results.
1. Conversational AI and Chatbots
One of the most visible applications of NLP is in conversational interfaces. Virtual assistants like Siri, Google Assistant, Alexa, and customer service bots use NLP to decipher user input and respond appropriately.
These bots don’t just follow scripts — they use intent recognition, context tracking, and sentiment detection to hold meaningful conversations. For instance, if a customer is expressing frustration in a support chat, the bot can escalate the query to a human or prioritize a faster resolution.
Advanced systems even remember previous conversations, allowing them to personalize replies based on past interactions, location, preferences, and user behavior.
2. Intelligent Recommendations
Many businesses now rely on recommendation engines to suggest products, services, articles, or entertainment options. NLP enhances these systems by analyzing unstructured text data such as product reviews, customer feedback, and user queries.
By understanding the language a user employs, NLP can infer preferences and match them to relevant items. For example, if someone frequently searches for “budget smartphones with good camera quality,” the system can filter out high-end devices and suggest mid-range phones with strong camera specs.
This semantic understanding boosts conversion rates by aligning suggestions with the user’s true intent — not just past behavior.
3. Personalized Email and Content Delivery
Marketers use NLP to go beyond demographic targeting. By evaluating the tone, language, and sentiment of customer interactions, NLP can help generate dynamic content that resonates with individual users.
For instance, NLP can segment email subscribers based on writing style in their messages or reviews — some may prefer casual language, while others respond better to formal tone. Automated systems can then generate tailored email copy for each group, increasing engagement and open rates.
NLP also assists in headline optimization, A/B testing language choices, and curating news or blog content relevant to a reader’s interests.
4. Sentiment-Aware Customer Support
Every interaction a user has with a brand — whether it’s a support ticket, a social media comment, or an online review — contains valuable emotional data. NLP techniques like sentiment analysis allow businesses to assess how customers feel and act accordingly.
For example, if a customer leaves a comment like, “I’m extremely disappointed with the delivery delay,” an NLP-driven system can flag it as negative and alert the support team. On the other hand, messages like, “Loved the packaging, great job!” can be tagged as positive and used in testimonials.
This approach enables brands to resolve issues faster, reward loyal customers, and build stronger emotional bonds.
5. Voice Interfaces and Accessibility
NLP also powers voice-driven interfaces and accessibility tools. From translating speech into commands to interpreting nuanced verbal instructions, these systems offer inclusive access to technology for users with disabilities or those in hands-free environments.
Moreover, voice assistants learn user habits over time. If you frequently ask, “What’s the weather like at 8 AM?” an intelligent assistant might start delivering morning weather reports proactively.
Such personalized auditory interactions enhance user convenience and foster a sense of connection with the digital environment.
The Role of Machine Learning in NLP
While rule-based systems once dominated early NLP, today’s personalization capabilities are largely due to machine learning — particularly deep learning models like transformers (e.g., BERT, GPT).
These models are trained on vast corpora of text data and can grasp the subtleties of grammar, syntax, and even idiomatic expressions. They don’t just respond to keywords; they understand sentence structure, word dependencies, and implied meanings.
By continuously learning from new data, they improve their performance over time. As more users engage with the system, it refines its ability to deliver accurate, context-aware, and personalized responses.
Real-World Applications Across Industries
Many sectors are reaping the benefits of NLP for personalization:
- Healthcare: NLP extracts insights from medical records, enabling doctors to receive relevant literature or treatment suggestions based on patient notes.
- Finance: Chatbots help users track expenses or get investment advice using natural queries.
- E-learning: Educational platforms adapt lesson recommendations based on how students phrase their questions or explain concepts.
- Travel: Personalized itinerary suggestions based on user-generated descriptions or travel reviews.
These examples demonstrate that NLP is not just a tech innovation — it’s a business strategy for creating unique and memorable user journeys.
Challenges and Ethical Considerations
Despite its advantages, NLP-based personalization also raises challenges:
- Bias in Data: If the training data is biased, models can reproduce or even amplify those biases.
- Privacy Concerns: Analyzing personal messages or voice inputs raises ethical questions around data usage and consent.
- Over-Personalization: Systems that over-tailor experiences might limit exposure to diverse perspectives, creating “information bubbles.”
To overcome these issues, businesses must prioritize transparency, secure user consent, and implement fairness checks in their NLP models.
The Future of Personalized NLP
Looking forward, NLP will continue to evolve in both accuracy and complexity. Future advancements may include:
- Emotion-aware NLP: Systems that not only detect emotion but respond empathetically.
- Multilingual personalization: Delivering context-aware responses in users’ native languages across regions.
- Real-time learning: Models that adapt mid-conversation to user feedback.
- Privacy-first NLP: Federated learning and edge computing may enable personalization without compromising data security.
The ultimate goal is to blur the line between human and machine interaction — making AI-driven systems indistinguishable from real-life assistants.
Conclusion
Natural Language Processing has revolutionized how we experience personalization in the digital world. From chatbots that understand sarcasm to systems that know your preferences better than you do, NLP enables technology to feel more human. As we move toward hyper-personalized, voice-first, and emotionally intelligent interfaces, NLP will be the bridge that connects cold code to real conversation.
Organizations that harness the power of NLP for personalized interactions will stand at the forefront of innovation — delighting users, increasing loyalty, and setting new standards for engagement.