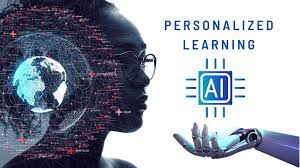
In today’s digital age, personalization has become a key factor in building customer loyalty, engagement, and satisfaction. Machine learning (ML) plays a significant role in enhancing personalized services by harnessing data, understanding customer preferences, and delivering tailored experiences. This technological advancement allows businesses to create more relevant and impactful interactions with their customers, fostering stronger connections.
Machine learning is a subset of artificial intelligence (AI) that empowers systems to learn from data, identify patterns, and make decisions without being explicitly programmed. By applying ML techniques, businesses can leverage vast amounts of customer data, generate insights, and provide tailored recommendations. Let’s explore how machine learning enhances personalized services across various industries and customer touchpoints.
1. Personalized Recommendations in E-commerce
The e-commerce industry has significantly benefited from machine learning, especially when it comes to product recommendations. Leading e-commerce giants like Amazon and eBay utilize ML algorithms to analyze user behavior, past purchases, browsing history, and even social media interactions. These algorithms generate personalized product recommendations that are more likely to appeal to individual customers.
By using collaborative filtering, content-based filtering, and hybrid models, ML systems can predict what products a customer might be interested in, increasing the likelihood of a purchase. For instance, when a customer frequently browses for running shoes, the platform will recommend shoes, accessories, or workout gear that align with their interests. This personalized experience not only enhances customer satisfaction but also drives higher conversion rates.
2. Dynamic Pricing Models
Pricing is a critical factor in any business, especially in industries like travel, retail, and hospitality. Machine learning enhances dynamic pricing models by analyzing a wide range of variables, including demand, competition, customer preferences, and market trends. These models allow businesses to adjust prices in real-time, offering personalized pricing strategies for each customer.
For example, a hotel might use machine learning to adjust room rates based on factors such as time of booking, customer loyalty, and the customer’s previous interactions. By analyzing historical data, the hotel can offer discounted rates or special offers to loyal customers, encouraging repeat visits. Similarly, ride-sharing companies like Uber utilize ML to set fare prices based on real-time demand and traffic conditions, ensuring a personalized and optimized pricing strategy.
3. Tailored Content in Media and Entertainment
The media and entertainment industry also benefits from machine learning, especially in providing personalized content recommendations. Streaming platforms like Netflix and Spotify use ML algorithms to analyze user preferences, viewing habits, and interactions. By continuously tracking these behaviors, ML systems can suggest movies, TV shows, or songs that align with a user’s tastes.
Personalization in this context goes beyond simple recommendations. Machine learning can predict which genres or types of content a user is likely to enjoy based on their past behavior. For example, if a user frequently watches romantic comedies, the system will recommend similar content in the same genre, increasing user satisfaction and engagement. This level of personalization has revolutionized the way people consume media, creating a highly customized and enjoyable entertainment experience.
4. Personalized Healthcare
Machine learning is making significant strides in the healthcare industry, particularly in delivering personalized treatment plans and improving patient outcomes. By analyzing patient data such as medical history, genetic information, and lifestyle factors, ML algorithms can help healthcare providers make more informed decisions about diagnoses, treatments, and medications.
Personalized medicine is at the forefront of this transformation. ML models can identify patterns in a patient’s data that might not be immediately apparent to human doctors, helping to detect early signs of diseases or predict future health risks. For example, ML can predict a patient’s risk of developing chronic conditions like diabetes or heart disease, allowing healthcare providers to recommend preventive measures or lifestyle changes tailored to the individual.
Additionally, ML-driven chatbots and virtual assistants are being used in healthcare to offer personalized health advice, appointment scheduling, and medication reminders, providing a more accessible and personalized service to patients.
5. Customer Support and Chatbots
Customer support is a vital aspect of delivering personalized services. Machine learning enhances customer service by powering intelligent chatbots and virtual assistants that can handle customer queries and provide tailored assistance. These ML-driven chatbots can understand natural language, learn from previous interactions, and provide personalized responses based on customer data.
For instance, a customer contacting a telecommunications provider may be greeted by a chatbot that instantly recognizes their account information and service history. The chatbot can then provide personalized solutions based on the customer’s previous interactions, such as troubleshooting an issue or recommending an upgrade. Over time, these systems continue to learn and improve, offering increasingly relevant and accurate responses to customers.
Moreover, machine learning can help predict customer service needs based on historical data. For example, if a customer frequently calls about billing issues, ML algorithms can flag the account and ensure that future interactions are handled by an agent with expertise in that area, leading to faster and more efficient resolutions.
6. Financial Services and Personalized Banking
In the financial sector, machine learning is playing a transformative role in delivering personalized banking services. By analyzing customer transaction data, spending habits, and financial goals, ML algorithms can provide personalized recommendations for saving, investing, and budgeting.
For example, a banking app powered by machine learning might analyze a user’s income and spending patterns to suggest personalized budgeting tips or offer tailored investment advice. It could also provide real-time alerts and reminders for upcoming bills or savings goals, helping users manage their finances more effectively.
Machine learning is also used in fraud detection. By analyzing transactional data in real-time, ML models can identify unusual patterns or suspicious activity, alerting both the customer and the financial institution. This not only enhances security but also provides a more personalized and secure banking experience.
7. Enhancing Customer Experience in Retail
In the retail industry, machine learning is revolutionizing the way customers interact with brands. Personalized experiences are now a core element of customer loyalty, and ML plays a central role in delivering these experiences across various touchpoints.
Retailers use ML to analyze customer behavior both online and offline, tailoring their marketing strategies and product offerings. For example, ML algorithms can predict which products a customer is likely to purchase based on their past shopping patterns, ensuring that relevant products are featured in their email campaigns, advertisements, or in-store displays.
Moreover, machine learning helps optimize the in-store experience. Retailers use ML to understand customer foot traffic, inventory patterns, and sales trends, allowing them to personalize in-store promotions and product placements. For instance, if a customer frequently buys organic food, the store can place similar products in their usual shopping path, enhancing the likelihood of a purchase.
8. Predictive Analytics for Better Customer Insights
Machine learning is a powerful tool for predictive analytics, allowing businesses to forecast customer behavior and trends. By analyzing historical data, businesses can predict what products or services customers are likely to need in the future. This capability is particularly useful for industries like fashion, electronics, and hospitality, where trends and customer demands change rapidly.
For example, a fashion retailer can use predictive analytics to forecast which styles or sizes will be in demand during an upcoming season. By analyzing past purchase data and external factors like weather forecasts, the retailer can stock inventory more effectively and tailor their marketing campaigns to target specific customer segments.
Similarly, businesses can use predictive analytics to identify customers who are likely to churn or discontinue their services. By recognizing these patterns early, companies can intervene with personalized offers or services to retain those customers.
Conclusion
Machine learning is revolutionizing the way businesses provide personalized services across industries. By harnessing the power of data, ML enables companies to understand customer preferences, predict behaviors, and deliver tailored experiences that enhance satisfaction and loyalty. From personalized recommendations in e-commerce to dynamic pricing, content delivery, and healthcare, the applications of machine learning in personalization are vast and transformative.
As machine learning technologies continue to evolve, the potential for creating even more personalized and impactful services will only grow. Businesses that embrace these advancements will be better equipped to meet the unique needs of their customers, fostering deeper connections and driving long-term success. The future of personalized services is undoubtedly intertwined with the continued development of machine learning, offering exciting opportunities for businesses to enhance their customer relationships and thrive in a competitive landscape.