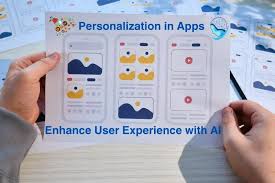
In an era where digital experiences increasingly shape how consumers engage with brands, personalization has become more than a luxury—it’s an expectation. Whether it’s a music app curating playlists, an e-commerce platform recommending products, or a streaming service queuing up binge-worthy shows, behind the magic lies a powerful force: Artificial Intelligence (AI). At the heart of this AI-driven personalization is one vital element—understanding user profiles and preferences.
This blog delves into the foundations of how AI understands and uses user profiles, how preferences are identified and interpreted, and why this knowledge is key to delivering seamless, relevant, and satisfying digital experiences.
The Concept of User Profiles in the AI Landscape
A user profile is essentially a structured representation of an individual’s digital identity. It encompasses demographic details, behavioral patterns, content interactions, preferences, purchase history, and sometimes even inferred emotions or intentions.
Unlike traditional customer databases, AI-enhanced user profiles are dynamic and constantly evolving. They adapt based on real-time inputs, learning from every click, swipe, pause, and scroll. This continuous learning enables a more personalized and predictive interaction, refining the digital experience to suit each unique user.
Why AI Personalization Begins with the User
AI systems don’t just personalize based on surface-level data. Instead, they dig deep into the user’s journey to understand the “why” behind the actions. Personalized content, recommendations, promotions, and layouts stem from the system’s ability to infer preferences, habits, and intent.
This deep understanding is important for several reasons:
- Relevance: Delivering content users are actually interested in increases engagement.
- Retention: Users are more likely to return to platforms that “understand” them.
- Conversion: Customized experiences often lead to better conversion rates.
- Customer Satisfaction: Meeting expectations through personalization improves overall satisfaction.
Key Elements of a User Profile in AI Personalization
To create an intelligent user profile, AI systems aggregate and synthesize data from various sources. The following components are commonly included:
1. Demographic Attributes
These are basic user characteristics such as:
- Age
- Gender
- Location
- Language
- Education level
- Occupation
Though relatively static, demographics help lay the groundwork for segmenting users into broad preference categories.
2. Behavioral Data
This is arguably the most valuable input. It includes:
- Browsing history
- Click patterns
- Time spent on specific pages
- Search queries
- Abandonment patterns
- Navigation flows
Behavioral data paints a real-time picture of user intent and interests. AI uses this to infer what users are looking for—even if they haven’t explicitly stated it.
3. Transactional History
AI also takes into account:
- Past purchases
- Subscription history
- Refunds or returns
- Payment method preferences
- Average order value
- Frequency of transactions
This helps in crafting tailored product recommendations, pricing offers, and targeted promotions.
4. Psychographic Insights
AI systems can now detect deeper traits, such as:
- Personal values
- Interests
- Hobbies
- Lifestyle preferences
- Emotional tone from reviews or messages
Advanced natural language processing (NLP) and sentiment analysis tools allow AI to extract psychographic details from unstructured text—such as product reviews, support chats, or social media posts.
5. Contextual Data
AI doesn’t just personalize for the person, but also for the moment. Contextual signals include:
- Device used (mobile, tablet, desktop)
- Time of day
- Current weather or location
- Connectivity (Wi-Fi or mobile data)
- Occasion or event (holiday, sale season)
This layer allows the system to offer what’s most relevant at any given time or environment.
How AI Understands and Predicts Preferences
Simply collecting data isn’t enough. The real power lies in AI’s ability to interpret and act upon it. Here’s how AI learns preferences:
1. Pattern Recognition
Machine learning algorithms scan data for recurring patterns. For instance, if a user frequently browses black sneakers but never makes a purchase, the system can start highlighting discounted black sneakers or offer bundle deals.
2. Clustering
Unsupervised learning techniques group users with similar behaviors or attributes together. This helps identify new segments or personas without needing predefined categories.
3. Reinforcement Learning
AI systems learn through trial and error. If a user clicks on a personalized recommendation, the model learns it was a success. If not, the model adjusts and tries a different approach next time.
4. Collaborative Filtering
This method uses information about similar users to make predictions. If users A and B have similar viewing habits and user A likes a new show, the system might recommend it to user B as well.
5. Content-Based Filtering
Here, AI recommends items with similar attributes to those a user has previously liked. For example, if a user enjoys romantic comedies, the system will suggest more titles from that genre.
Building and Maintaining Accurate User Profiles
Creating a profile is just the beginning. Keeping it accurate and useful is an ongoing process. AI systems ensure this by:
- Continuous Learning: Models adapt as users’ behaviors change over time.
- Multi-Touch Integration: Data is pulled from all points of interaction, online and offline.
- Feedback Loops: Explicit user feedback (ratings, reviews, surveys) helps validate and adjust assumptions.
- Data Hygiene: Systems must regularly remove outdated, duplicated, or irrelevant data to maintain profile accuracy.
Ethical Considerations in User Profiling
As personalization becomes more sophisticated, concerns around ethics and privacy rise. It’s essential to ensure:
- Transparency: Users should know when and how their data is being used.
- Consent: Data collection must be opt-in and clearly explained.
- Bias Monitoring: AI systems should be audited for discriminatory or unfair outcomes.
- Data Security: Personal profiles must be protected from breaches and misuse.
Responsible personalization balances intelligence with user trust.
Real-World Applications of AI-Powered Profiles
User profiles are actively transforming various industries. A few examples include:
- Retail: Personalized homepages, product recommendations, and promotions tailored to shopping habits.
- Media Streaming: Customized playlists, movie suggestions, and notification timing based on past consumption.
- Travel: Vacation deals curated to traveler preferences, seasonal habits, and destination interests.
- Healthcare: Personalized treatment plans or fitness suggestions based on medical history and lifestyle.
In each case, the goal remains the same: to treat users not as anonymous numbers, but as individuals with unique needs.
The Future of User Profiling in AI Personalization
As AI continues to evolve, so will the sophistication of user profiles. In the near future, we can expect:
- Emotionally Intelligent AI: Systems that sense mood and adjust tone or suggestions accordingly.
- Augmented Personalization: Real-time personalization in AR/VR environments.
- Hypergranular Segmentation: AI that understands even the smallest nuance in preferences.
- Predictive Assistance: Systems that preemptively provide solutions before a user even realizes the need.
With voice assistants, smart wearables, and biometric sensors joining the data mix, user profiles will become even richer—allowing hyper-personalized experiences across all aspects of daily life.
Conclusion
Understanding user profiles and preferences is the cornerstone of effective AI personalization. Through a mix of data collection, behavioral analysis, and machine learning, AI can shape experiences that are not only relevant but also intuitive and engaging.
The challenge lies in managing this personalization responsibly—respecting privacy, maintaining data accuracy, and delivering true value to the user. When done right, AI-powered personalization doesn’t just enhance engagement; it builds trust and loyalty in an increasingly digital world.